Freshness Evaluation of Beef using MOS Based E-Nose
2023 International Conference on Intelligent Data Communication Technologies and Internet of Things (IDCIoT)(2023)
摘要
Beef is a typical agricultural product that is abundant in high quality protein, vitamins, minerals and acts as a rich source of iron that helps to cut risk of anemia but unfortunately, it is a perishable food type as it contains high moisture content, and there are concerns regarding health hazards, quality, stability and safety if consumed. A freshness monitoring system for food is required to guarantee that the food ingested is of good quality. Recently, the freshness of the food is detected using many techniques such as spectroscopy, subjective sensory evaluation, computer vision, chemical or instrumentation test, multispectral and hyperspectral imaging and electronic nose. In this study, we have used a self-made, low-cost portable electronic nose which is designed to mimic human olfaction and to detect and discriminate among complex odors using an array of eight MOS sensors (chemical sensors). An e-nose is used in the quality control of food and beverages, evaluation of freshness and detection of contaminants or adulteration. The e- nose model developed is primarily aimed at determining the freshness of various beef samples and the two main components of e-nose are a sensing system and automated pattern recognition system. The obtained analog outputs are recorded in an excel sheet, which is then analyzed by using various machine learning techniques that helps to obtain the accuracy of the collected data set, among which Support Vector Machine (SVM) algorithm gives more accuracy, sensitivity, specificity of 91.5%, 91.08%, 90.90% respectively. In future, an improved version of this model can be used to analyze the quality of other food materials also.
更多查看译文
关键词
Electronic Nose,Sensors,Machine Learning,Beef
AI 理解论文
溯源树
样例
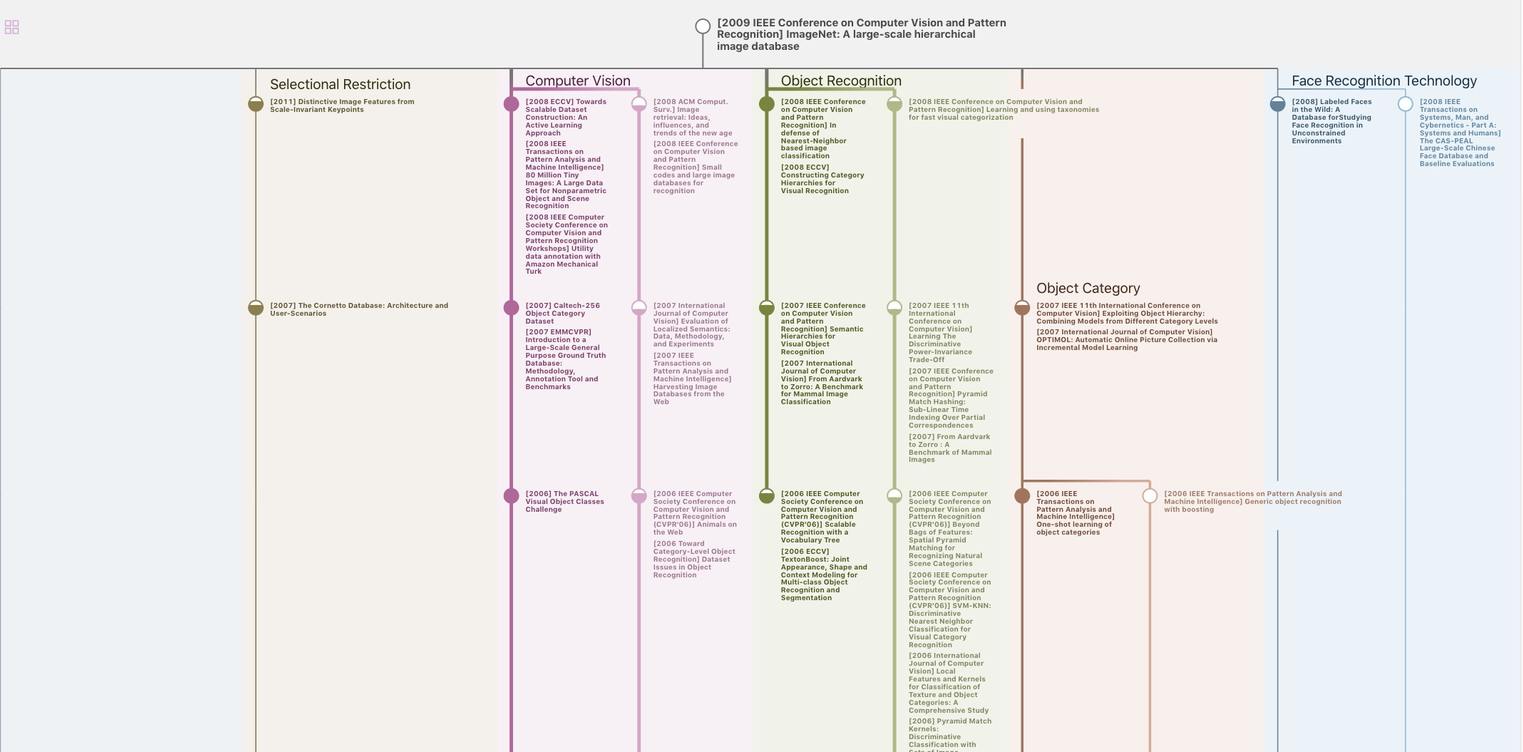
生成溯源树,研究论文发展脉络
Chat Paper
正在生成论文摘要