Towards a real-time demand response framework for smart communities using clustering techniques
arxiv(2023)
摘要
The present study explores the use of clustering techniques for the design and implementation of a demand response (DR) program for commercial and residential prosumers. The goal of the program is to shift the participants' consumption behavior to mitigate two issues a) the reverse power flow at the primary substation, that occurs when generation from solar panels in the local grid exceeds consumption and b) the system wide peak demand, that typically occurs during hours of the late afternoon. For the clustering stage, three popular algorithms for electrical load clustering are employed -- namely k-means, k-medoids and a hierarchical clustering algorithm -- alongside two different distance metrics -- namely euclidean and constrained Dynamic Time Warping (DTW). We evaluate the methods using different validation metrics including a novel metric -- namely peak performance score (PPS) -- that we propose in the context of this study. The best setup is employed to divide daily prosumer load profiles into clusters and each cluster is analyzed in terms of load shape, mean entropy and distribution of load profiles from each load type. These characteristics are then used to distinguish the clusters that would be most likely to aid with the DR schemes would fit each cluster. Finally, we conceptualize a DR system that combines forecasting, clustering and a price-based demand projection engine to produce daily individualized DR recommendations and pricing policies for prosumers participating in the program. The results of this study can be useful for network operators and utilities that aim to develop targeted DR programs for groups of prosumers within flexible energy communities.
更多查看译文
AI 理解论文
溯源树
样例
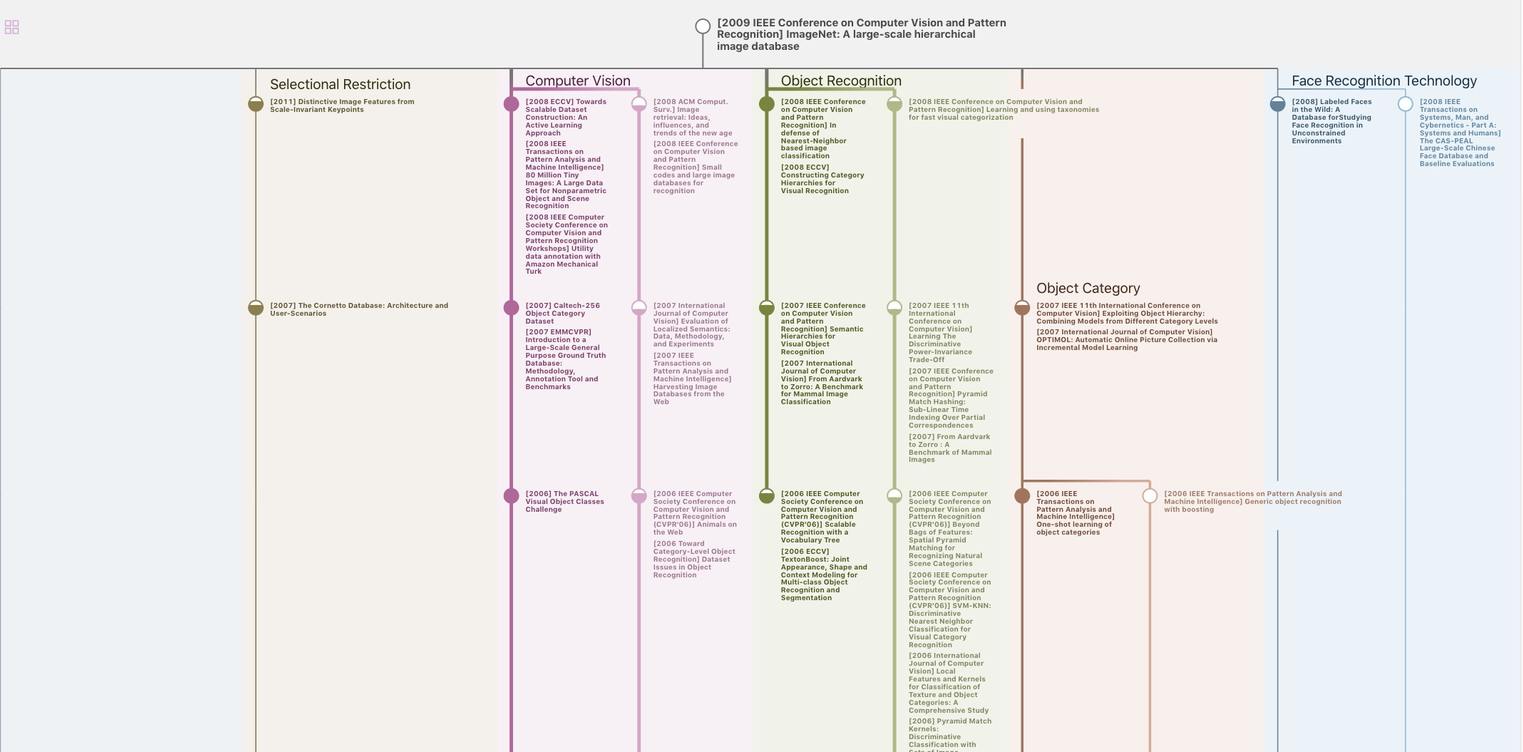
生成溯源树,研究论文发展脉络
Chat Paper
正在生成论文摘要