Double-loop randomized quasi-Monte Carlo estimator for nested integration
arxiv(2023)
摘要
Characterized by an outer integral connected to an inner integral through a
nonlinear function, nested integration is a challenging problem in various
fields, such as engineering and mathematical finance. The available numerical
methods for nested integration based on Monte Carlo (MC) methods can be
prohibitively expensive owing to the error propagating from the inner to the
outer integral. Attempts to enhance the efficiency of these approximations
using the quasi-MC (QMC) or randomized QMC (rQMC) method have focused on either
the inner or outer integral approximation. This work introduces a novel nested
rQMC method that simultaneously addresses the approximation of the inner and
outer integrals. This method leverages the unique nested integral structure to
offer a more efficient approximation mechanism. By incorporating Owen's
scrambling techniques, we address integrands exhibiting infinite variation in
the Hardy–Krause sense, enabling theoretically sound error estimates. As the
primary contribution, we derive asymptotic error bounds for the bias and
variance of our estimator, along with the regularity conditions under which
these bounds can be attained. In addition, we provide nearly optimal sample
sizes for the rQMC approximations underlying the numerical implementation of
the proposed method. Moreover, we indicate how to combine this method with
importance sampling to remedy the measure concentration arising in the inner
integral. We verify the estimator quality through numerical experiments in the
context of expected information gain estimation. We compare the computational
efficiency of the nested rQMC method against standard nested MC integration for
two case studies: one in thermomechanics and the other in pharmacokinetics.
These examples highlight the computational savings and enhanced applicability
of the proposed approach.
更多查看译文
AI 理解论文
溯源树
样例
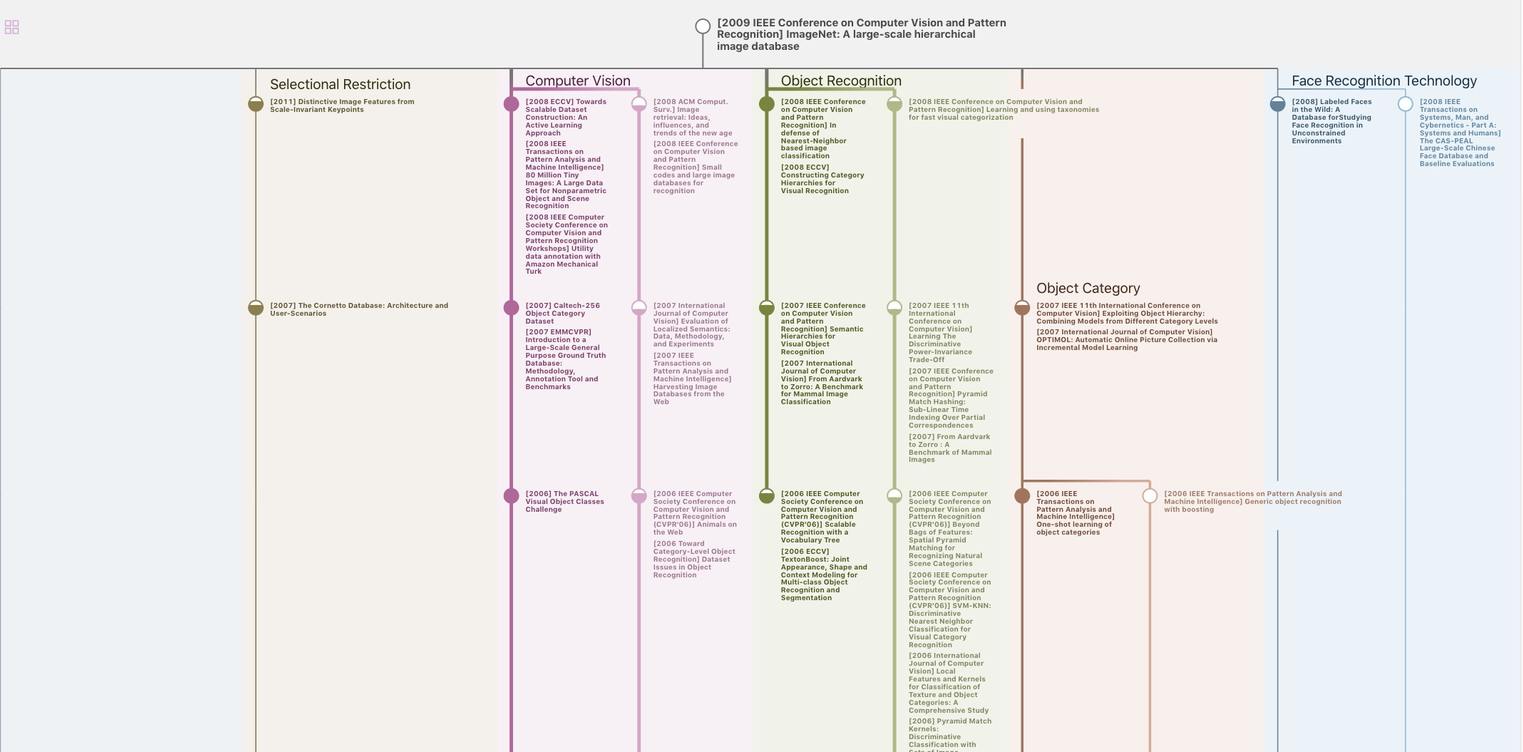
生成溯源树,研究论文发展脉络
Chat Paper
正在生成论文摘要