Automated classification of seismic signals recorded on the & Aring;knes rock slope, Western Norway, using a convolutional neural network
Earth Surface Dynamics(2023)
摘要
A convolutional neural network (CNN) was implemented to automatically classify 15 years of seismic signals recorded by an eight-geophone network installed around the back scarp of the & Aring;knes rock slope in Norway. Eight event classes could be identified and are adapted from the typology proposed by Provost et al. (2018), of which five could be directly related to movements on the slope. Almost 60 000 events were classified automatically based on their spectrogram images. The performance of the classifier is estimated to be near 80 %. The statistical analysis of the results shows a strong seasonality of the microseismic activity at & Aring;knes with an annual increase in springtime when snow melts and the temperature oscillates around the freezing point, mainly caused by events within classes of low-frequency slope quakes and tremors. The clear link between annual temperature variations and microseismic activity could be confirmed, supporting thawing and freezing processes as the origins. Other events such as high-frequency and successive slope quakes occur throughout the year and are potentially related to the steady creep of the sliding plane. The huge variability in the annual event number cannot be solely explained by average temperatures or varying detectability of the network. Groundwater recharge processes and their response to precipitation episodes are known to be a major factor of sliding at & Aring;knes, but the relationship with microseismic activity is less obvious and could not be demonstrated.
更多查看译文
关键词
seismic signals,convolutional neural network,neural network,automated classification
AI 理解论文
溯源树
样例
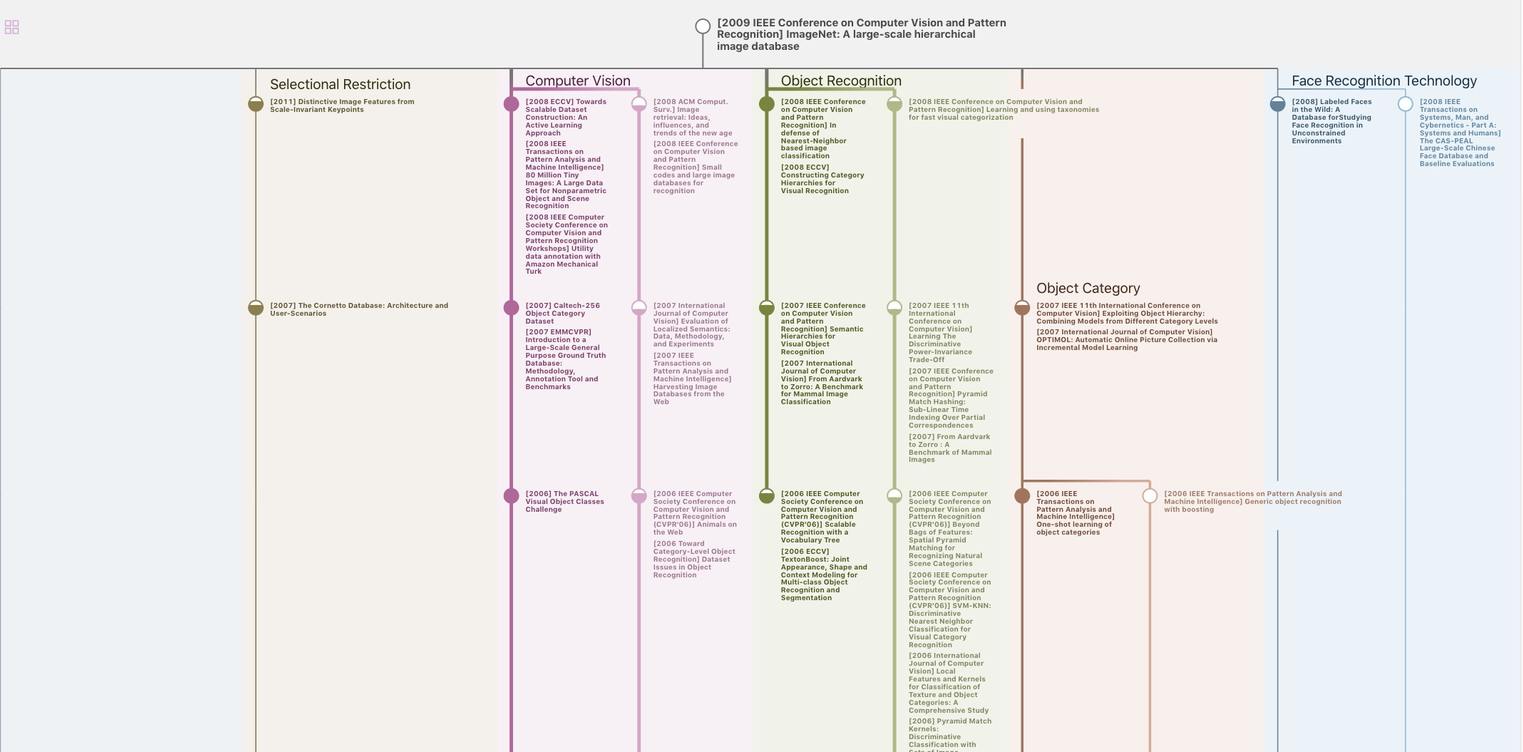
生成溯源树,研究论文发展脉络
Chat Paper
正在生成论文摘要