SATBA: An Invisible Backdoor Attack Based On Spatial Attention
arxiv(2023)
摘要
Backdoor attacks pose a new and emerging threat to AI security, where Deep Neural Networks (DNNs) are trained on datasets added to hidden trigger patterns. Although the poisoned model behaves normally on benign samples, it produces anomalous results on samples containing the trigger pattern. Nevertheless, most existing backdoor attacks face two significant drawbacks: their trigger patterns are visible and easy to detect by human inspection, and their injection process leads to the loss of natural sample features and trigger patterns, thereby reducing the attack success rate and the model accuracy. In this paper, we propose a novel backdoor attack named SATBA that overcomes these limitations by using spatial attention mechanism and U-type model. Our attack leverages spatial attention mechanism to extract data features and generate invisible trigger patterns that are correlated with clean data. Then it uses U-type model to plant these trigger patterns into the original data without causing noticeable feature loss. We evaluate our attack on three prominent image classification DNNs across three standard datasets and demonstrate that it achieves high attack success rate and robustness against backdoor defenses. Additionally, we also conduct extensive experiments on image similarity to highlight the stealthiness of our attack.
更多查看译文
关键词
invisible backdoor attack,spatial attention
AI 理解论文
溯源树
样例
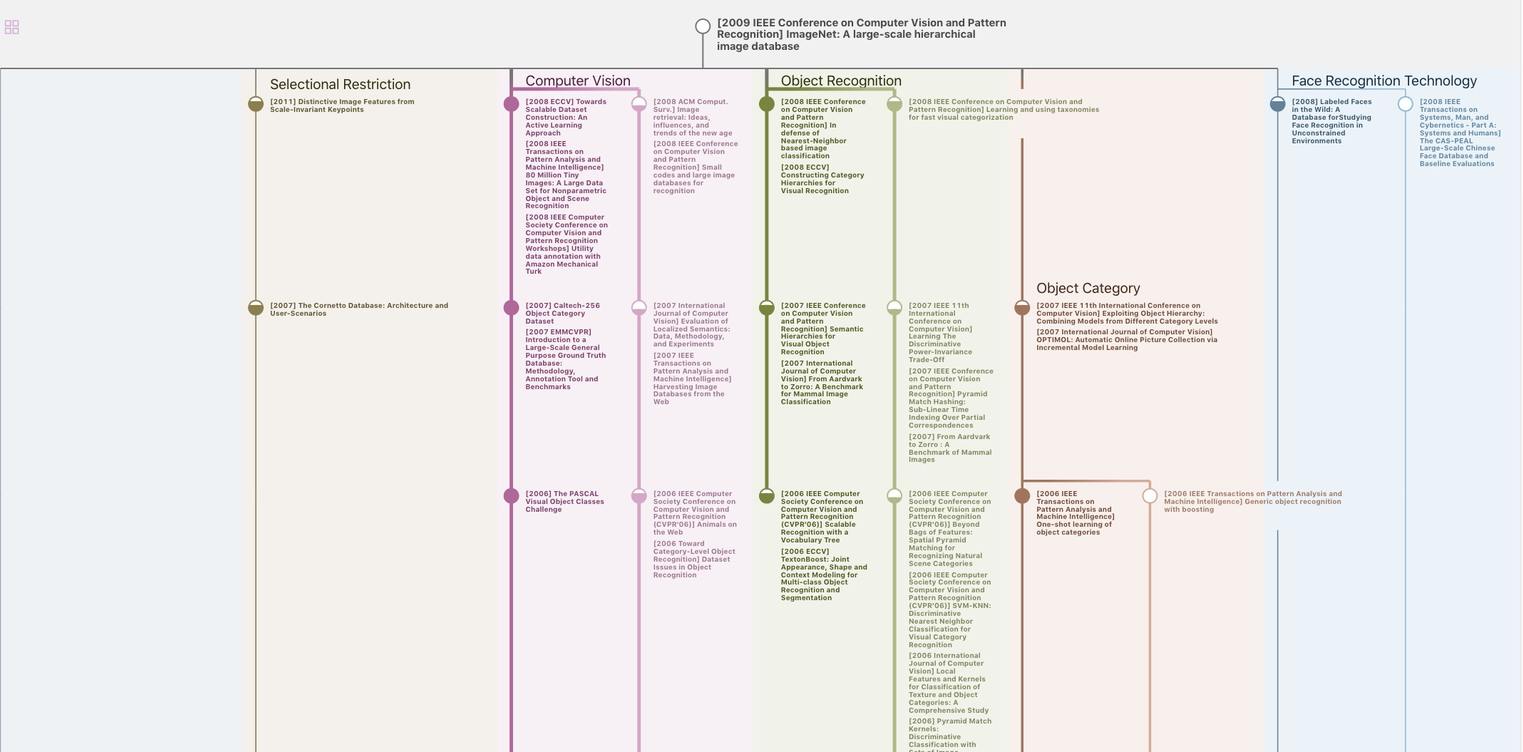
生成溯源树,研究论文发展脉络
Chat Paper
正在生成论文摘要