Leveraging Jumpy Models for Planning and Fast Learning in Robotic Domains
arxiv(2023)
摘要
In this paper we study the problem of learning multi-step dynamics prediction models (jumpy models) from unlabeled experience and their utility for fast inference of (high-level) plans in downstream tasks. In particular we propose to learn a jumpy model alongside a skill embedding space offline, from previously collected experience for which no labels or reward annotations are required. We then investigate several options of harnessing those learned components in combination with model-based planning or model-free reinforcement learning (RL) to speed up learning on downstream tasks. We conduct a set of experiments in the RGB-stacking environment, showing that planning with the learned skills and the associated model can enable zero-shot generalization to new tasks, and can further speed up training of policies via reinforcement learning. These experiments demonstrate that jumpy models which incorporate temporal abstraction can facilitate planning in long-horizon tasks in which standard dynamics models fail.
更多查看译文
关键词
jumpy models,planning,fast learning,robotic
AI 理解论文
溯源树
样例
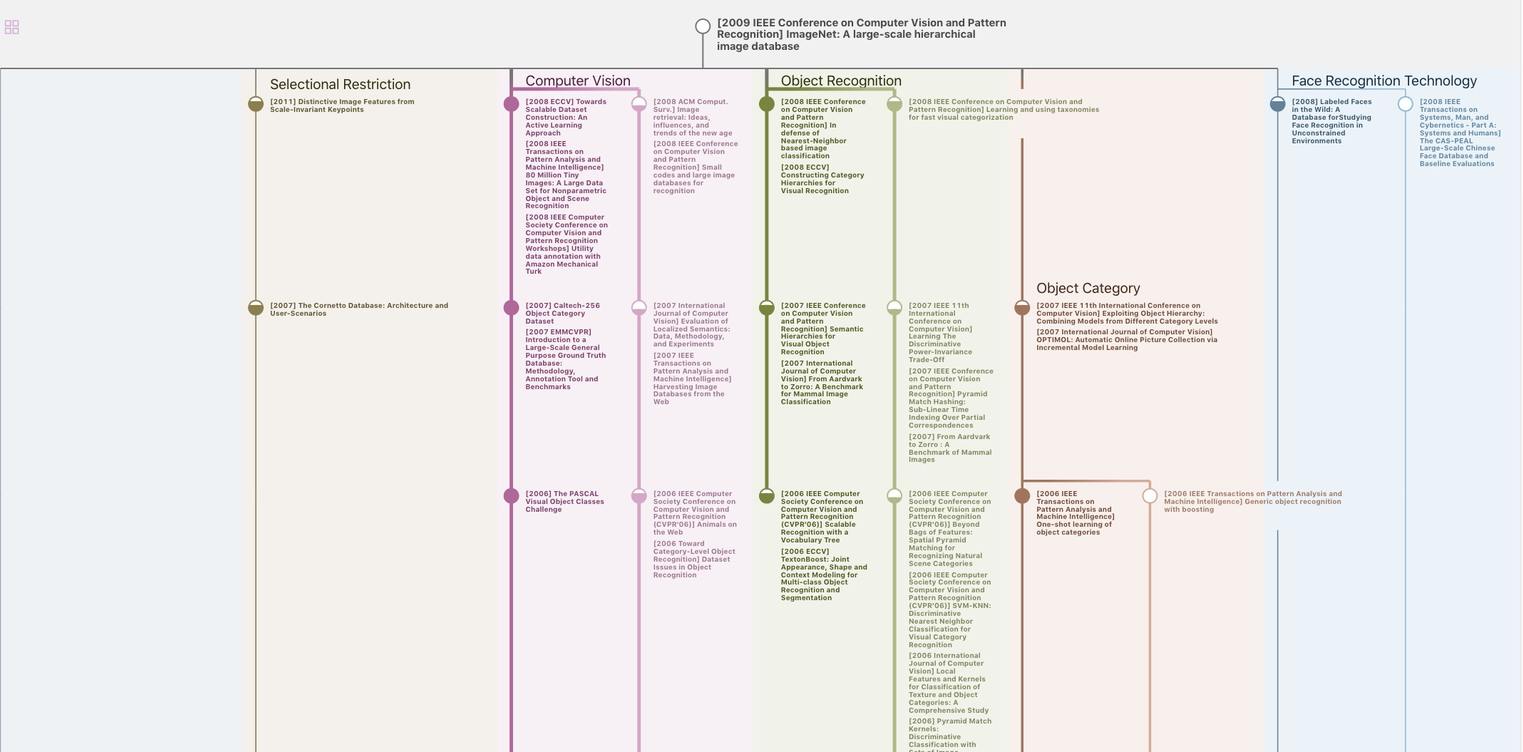
生成溯源树,研究论文发展脉络
Chat Paper
正在生成论文摘要