Self-Supervised Denoising of single OCT image with Self2Self-OCT Network
2022 IEEE 7th Optoelectronics Global Conference (OGC)(2022)
摘要
In recent years, supervised deep learning of image denoising has attracted extensive research interests. Those methods usually required numerous pairs of noisy image and its corresponding clean image in training processing. However, in most real situations, it is hard to collect high-quality clean images such as optical coherence tomography (OCT) images. Therefore, it is of great significance to study a effective de-noising network without clean images for supervising, which is only trained with noisy image. In this article, for a single OCT image, we propose a self-supervised deep learning model called Self2Self-OCT network by improved the Self2Self network and added a loss function that can effectively remove the background noise of OCT images, which makes the whole training do not need correlative clean images. Specifically, we use gated convolution to replace the partial convolution layer of the encoder’s block in Self2Self. The input image and its Bernoulli sampling instance are put into our network respectively, and the background noise attenuation loss is added to loss function during training. The result is estimated based on the average value of multiple prediction outputs. The experiments with different OCT images indicate that proposed model not only has obvious advantages compared with the existing single deep learning methods and non-learning methods, but also surpasses the supervised learning of a small number of sample training.
更多查看译文
关键词
self-supervised,speckle noise,denoising,optical coherence tomography image
AI 理解论文
溯源树
样例
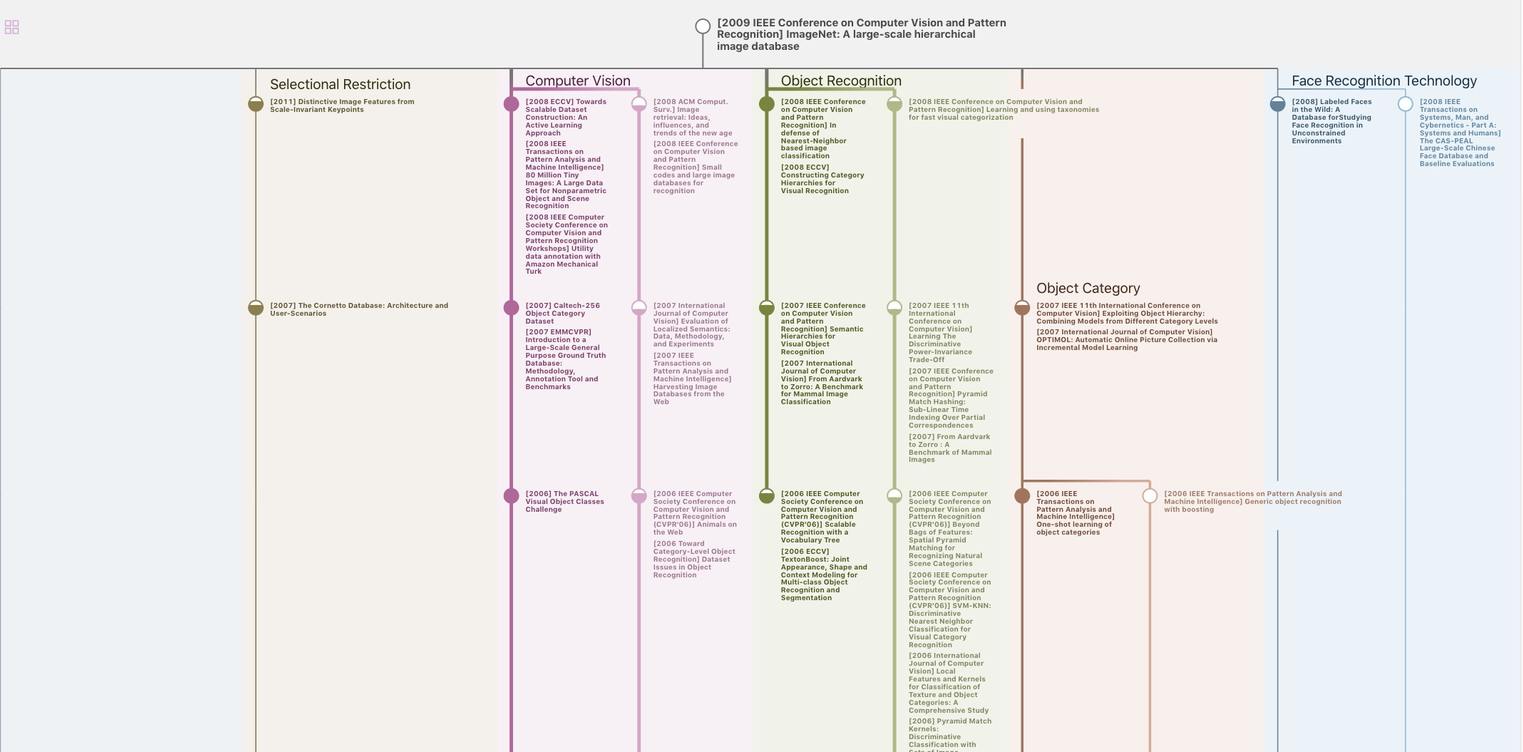
生成溯源树,研究论文发展脉络
Chat Paper
正在生成论文摘要