DoubleSG-DTA: Deep Learning for Drug Discovery: Case Study on the Non-Small Cell Lung Cancer with EGFR T 790 M Mutation.
Pharmaceutics(2023)
摘要
Drug-targeted therapies are promising approaches to treating tumors, and research on receptor-ligand interactions for discovering high-affinity targeted drugs has been accelerating drug development. This study presents a mechanism-driven deep learning-based computational model to learn double drug sequences, protein sequences, and drug graphs to project drug-target affinities (DTAs), which was termed the DoubleSG-DTA. We deployed lightweight graph isomorphism networks to aggregate drug graph representations and discriminate between molecular structures, and stacked multilayer squeeze-and-excitation networks to selectively enhance spatial features of drug and protein sequences. What is more, cross-multi-head attentions were constructed to further model the non-covalent molecular docking behavior. The multiple cross-validation experimental evaluations on various datasets indicated that DoubleSG-DTA consistently outperformed all previously reported works. To showcase the value of DoubleSG-DTA, we applied it to generate promising hit compounds of Non-Small Cell Lung Cancer harboring EGFRT790M mutation from natural products, which were consistent with reported laboratory studies. Afterward, we further investigated the interpretability of the graph-based "black box" model and highlighted the active structures that contributed the most. DoubleSG-DTA thus provides a powerful and interpretable framework that extrapolates for potential chemicals to modulate the systemic response to disease.
更多查看译文
关键词
cross-multi-head attention,drug discovery,drug–target affinity,graph isomorphism network,non-small cell lung cancer,squeeze-and-excitation network
AI 理解论文
溯源树
样例
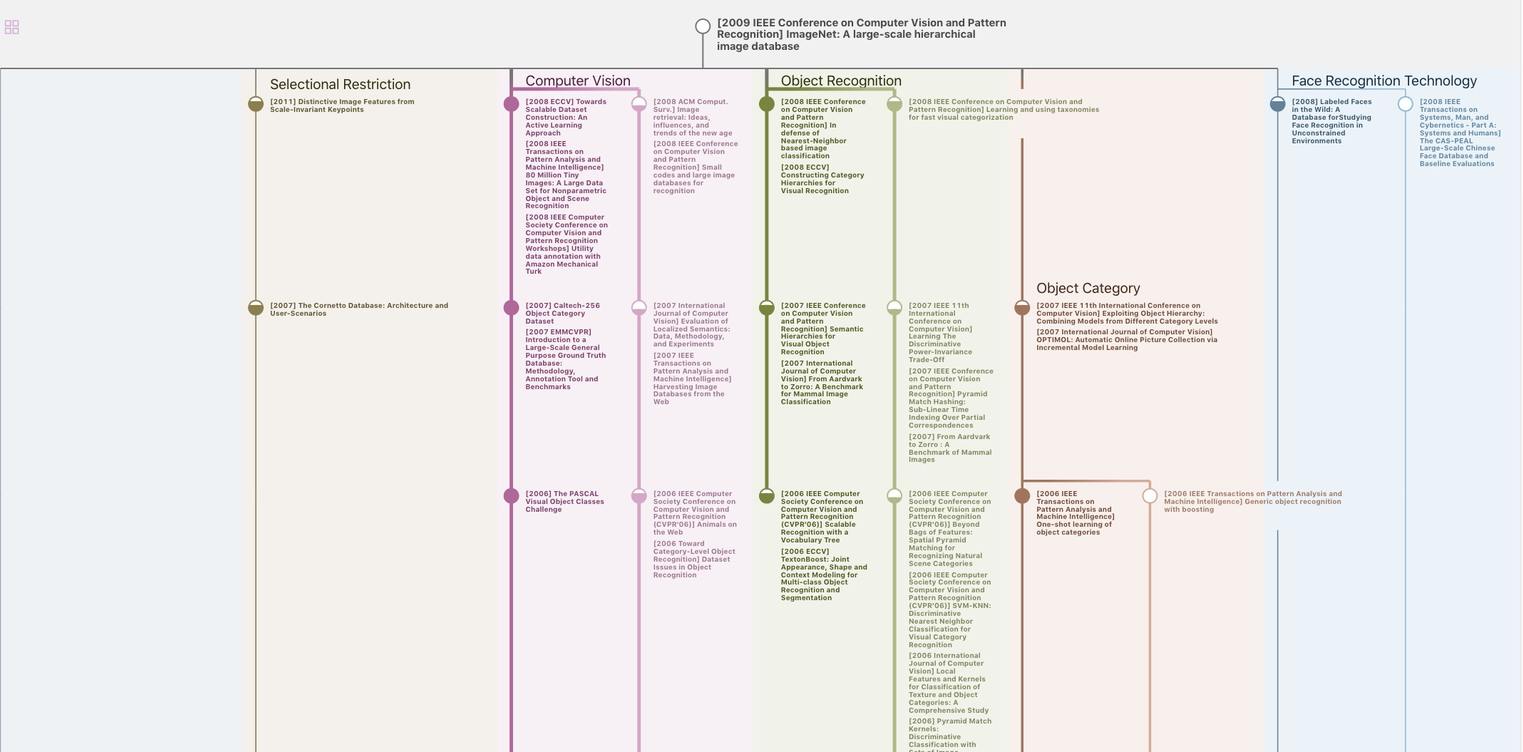
生成溯源树,研究论文发展脉络
Chat Paper
正在生成论文摘要