GLUECons: A Generic Benchmark for Learning Under Constraints
arxiv(2023)
摘要
Recent research has shown that integrating domain knowledge into deep learning architectures is effective -- it helps reduce the amount of required data, improves the accuracy of the models' decisions, and improves the interpretability of models. However, the research community is missing a convened benchmark for systematically evaluating knowledge integration methods. In this work, we create a benchmark that is a collection of nine tasks in the domains of natural language processing and computer vision. In all cases, we model external knowledge as constraints, specify the sources of the constraints for each task, and implement various models that use these constraints. We report the results of these models using a new set of extended evaluation criteria in addition to the task performances for a more in-depth analysis. This effort provides a framework for a more comprehensive and systematic comparison of constraint integration techniques and for identifying related research challenges. It will facilitate further research for alleviating some problems of state-of-the-art neural models.
更多查看译文
关键词
constraints,generic benchmark,learning,gluecons
AI 理解论文
溯源树
样例
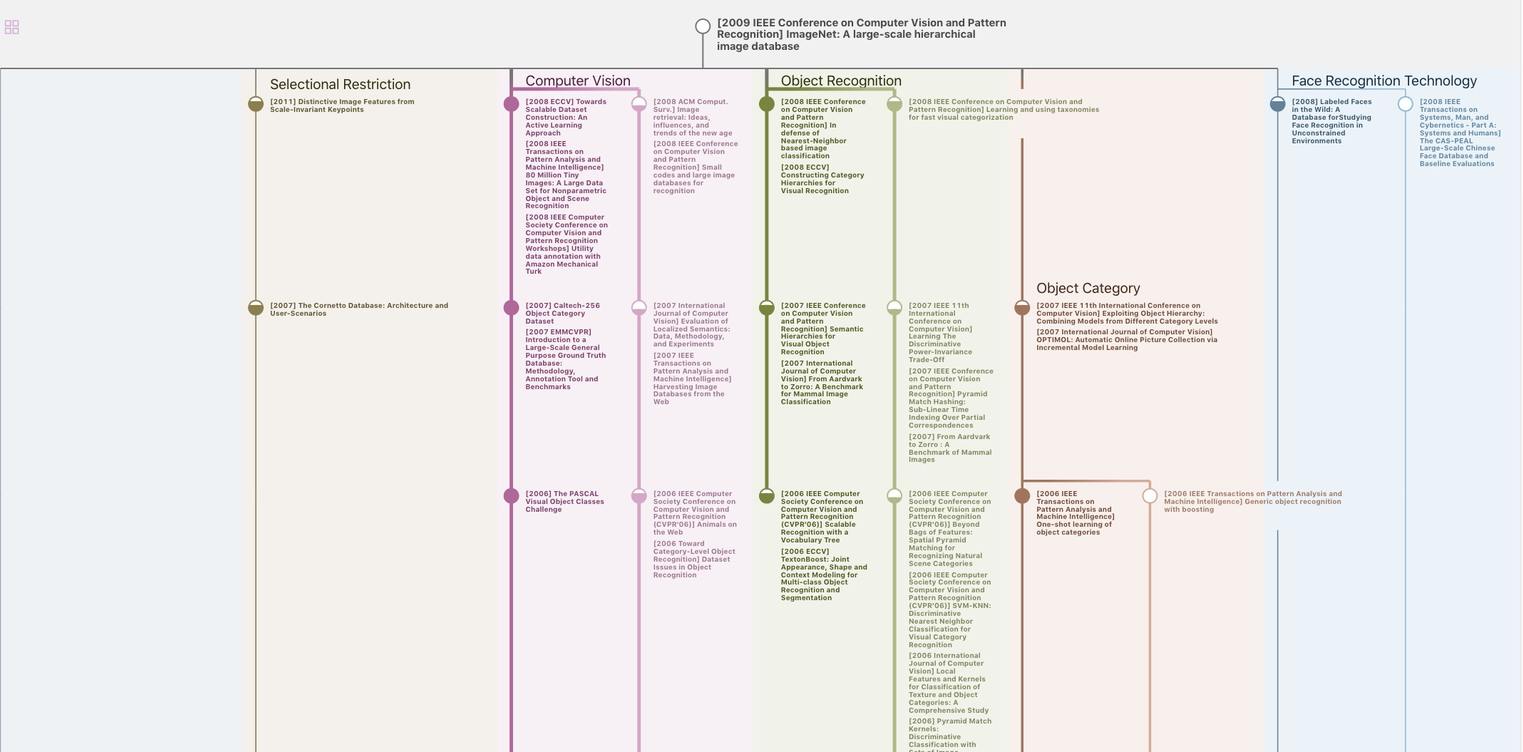
生成溯源树,研究论文发展脉络
Chat Paper
正在生成论文摘要