A Simple Way to Incorporate Target Structural Information in Molecular Generative Models.
biorxiv(2023)
摘要
Deep learning generative models are now being applied in various fields including drug discovery. In this work, we propose a novel approach to include target 3D structural information in molecular generative models for structure-based drug design. The method combines a message-passing neural network model that predicts docking scores with a generative neural network model as its reward function to navigate the chemical space searching for molecules that bind favorably with a specific target. A key feature of the method is the construction of target-specific molecular sets for training, designed to overcome potential transferability issues of surrogate docking models through a two-round training process. Consequently, this enables accurate guided exploration of the chemical space without reliance on the collection of prior knowledge about active and inactive compounds for the specific target. Tests on eight target proteins showed a 100-fold increase in hit generation compared to conventional docking calculations and the ability to generate molecules similar to approved drugs or known active ligands for specific targets without prior knowledge. This method provides a general and highly efficient solution for structure-based molecular generation.
更多查看译文
关键词
target structural information,structural information,molecular,models
AI 理解论文
溯源树
样例
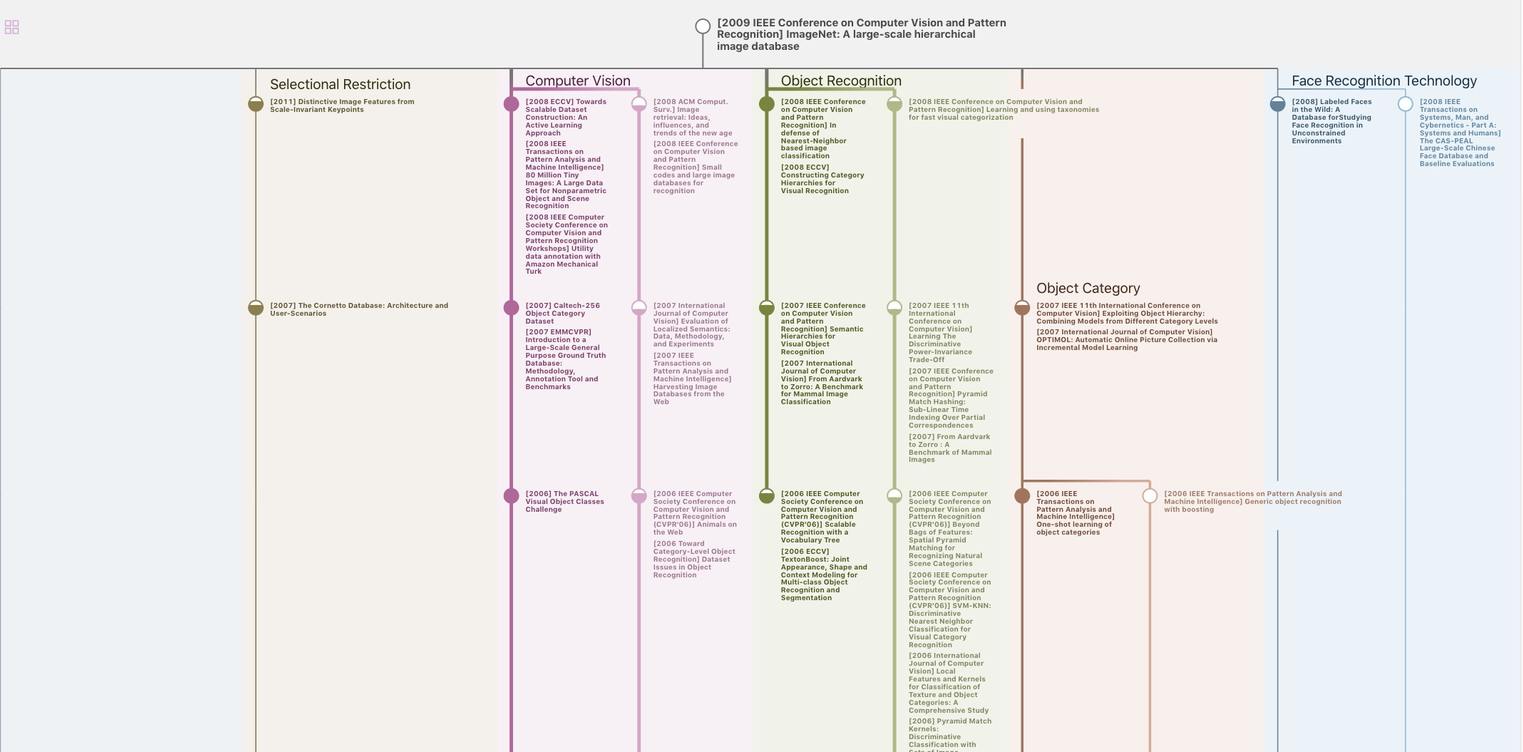
生成溯源树,研究论文发展脉络
Chat Paper
正在生成论文摘要