Natural Language-conditioned Reinforcement Learning with Inside-out Task Language Development and Translation
arxiv(2023)
摘要
Natural Language-conditioned reinforcement learning (RL) enables the agents to follow human instructions. Previous approaches generally implemented language-conditioned RL by providing human instructions in natural language (NL) and training a following policy. In this outside-in approach, the policy needs to comprehend the NL and manage the task simultaneously. However, the unbounded NL examples often bring much extra complexity for solving concrete RL tasks, which can distract policy learning from completing the task. To ease the learning burden of the policy, we investigate an inside-out scheme for natural language-conditioned RL by developing a task language (TL) that is task-related and unique. The TL is used in RL to achieve highly efficient and effective policy training. Besides, a translator is trained to translate NL into TL. We implement this scheme as TALAR (TAsk Language with predicAte Representation) that learns multiple predicates to model object relationships as the TL. Experiments indicate that TALAR not only better comprehends NL instructions but also leads to a better instruction-following policy that improves 13.4% success rate and adapts to unseen expressions of NL instruction. The TL can also be an effective task abstraction, naturally compatible with hierarchical RL.
更多查看译文
关键词
reinforcement learning,language-conditioned development,inside-out
AI 理解论文
溯源树
样例
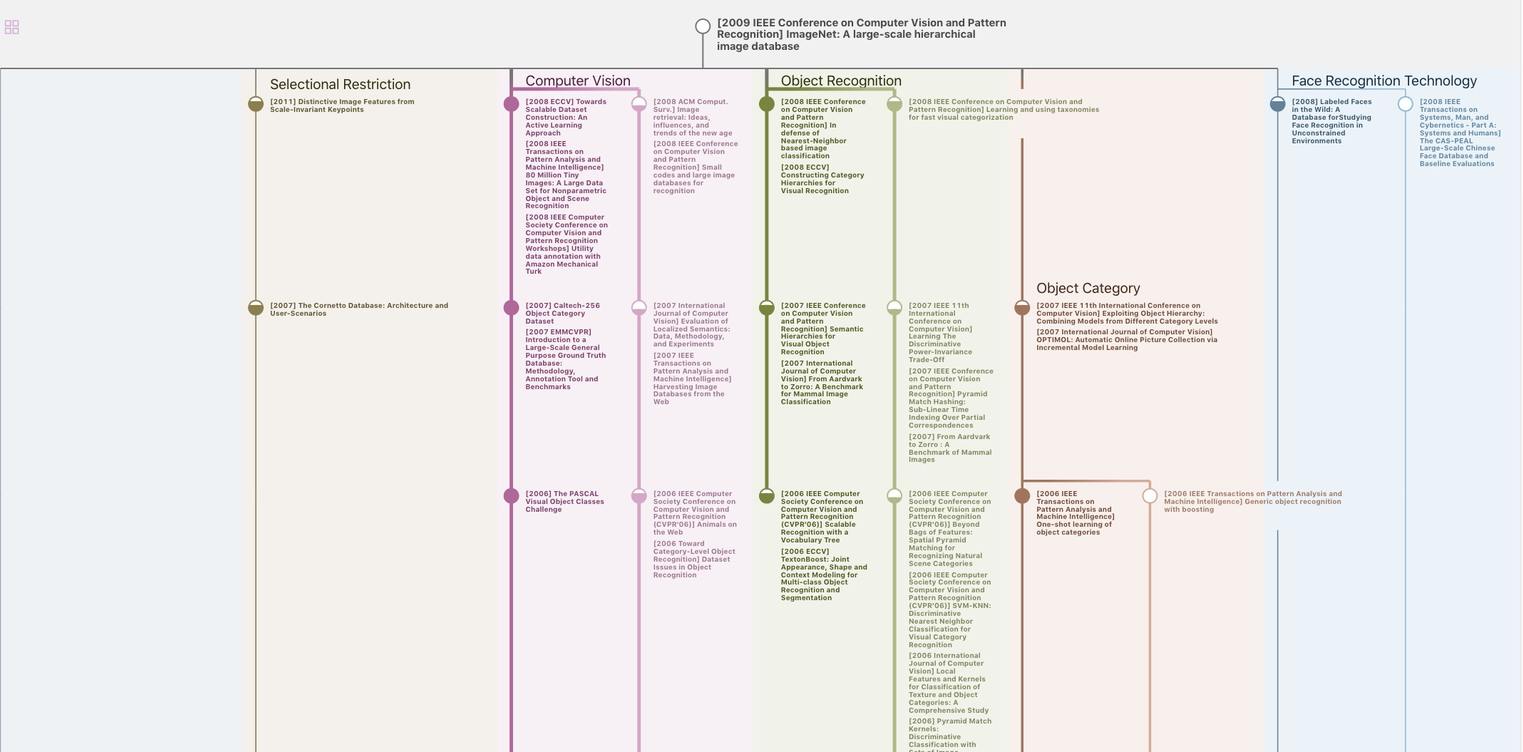
生成溯源树,研究论文发展脉络
Chat Paper
正在生成论文摘要