Animal Pose Estimation Algorithm Based on the Lightweight Stacked Hourglass Network
IEEE Access(2023)
摘要
Pose estimation has been a hot topic in the field of machine vision in recent years. Animals exist widely in nature, and the analysis of their shape and movement is important in many fields and industries. In the pose estimation task, to improve the detection accuracy, the existing models often need to consume a lot of computing and memory resources. Therefore, it is a key problem for the pose estimation methods to carry out a lightweight model and reduce the computational overhead on the premise of ensuring model accuracy. In this paper, we focus on the structure of the convolutional neural network in animal pose estimation, construct a lightweight and efficient stacked hourglass network model oriented to optimize the balance of model computation and accuracy, and implement the application algorithm design based on it. Aiming at the problem of large parameters in depthwise convolutional neural networks, a lightweight residual module is proposed, that is, based on the lightweight efficient channel attention improved conditional channel-weighted method (ICCW-Bottle), thereby reducing the weight of the network and obtaining the feature information of different scales. Given the problem that a large amount of feature information is easily lost after the network pooling operation, a lightweight dual-branch fusion module is proposed that fully integrates high-level semantic information and low-level detailed features under the condition of a small number of parameters. Finally, the same as the CC-SSL method: the model is trained jointly using synthetic and real animal datasets, but the CC-SSL method does not take into account the computational power of the model, which consumes a lot of time and memory to run. Through experiments, it is known that compared with the CC-SSL method, the PCK@0.05 of this method is increased by 5.5% on the TigDog dataset. The model in this paper reduces the number of parameters and calculations of the network while ensuring less information loss and model accuracy. The ablation experiment verifies the advancement and effectiveness of the overall network.
更多查看译文
关键词
Convolutional neural networks,Behavioral sciences,Pose estimation,Image resolution,Feature extraction,Animals,Computational efficiency,Animal pose estimation,stacked hourglass networks,lightweight,residual module,feature fusion
AI 理解论文
溯源树
样例
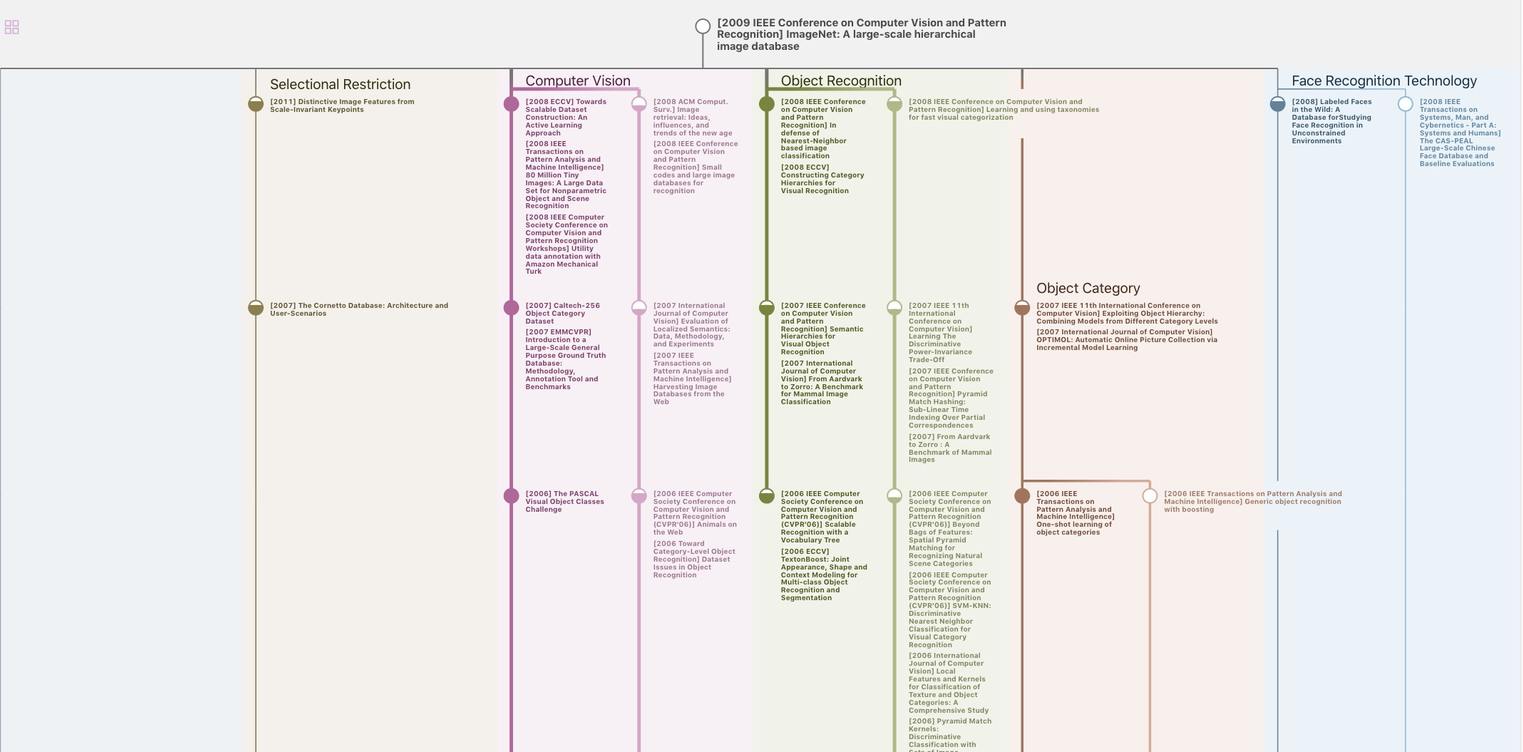
生成溯源树,研究论文发展脉络
Chat Paper
正在生成论文摘要