Scenario Compaction and Ensemble with RAN Digital Twin for Efficient and Robust Learning.
GLOBECOM (Workshops)(2022)
摘要
As wireless systems and protocols evolve to support various services requiring high performance, deploying and operating the wireless mobile networks optimally become more challenging. To address this, artificial intelligence (AI) is getting a tremendous amount of interest for its ability to provide adaptability, scalability, and optimality in an automated and data-driven manner. Operating the network involves decision making processes in highly non-deterministic environments. This calls for the need for RAN Digital Twin, which replicates the states and behaviors of the real networks into virtual simulated networks, so making trial-and-error based optimization possible. However, there still remains challenges in terms of resource-efficiency and real-world applicability. To address efficiency, we propose scenario compaction, which generates a representative condensed scenario using the large volume of real data. We also propose scenario ensemble to train a model robust to the gap between the real and the replicated. As a target application, we tackle a cell on/off based energy-saving operation using reinforcement learning in this paper. Through experiments, we show that our methods provide learning efficiency and they provide an AI module with robust performance when applied to a real base station.
更多查看译文
关键词
ran digital twin,ensemble,learning,compaction
AI 理解论文
溯源树
样例
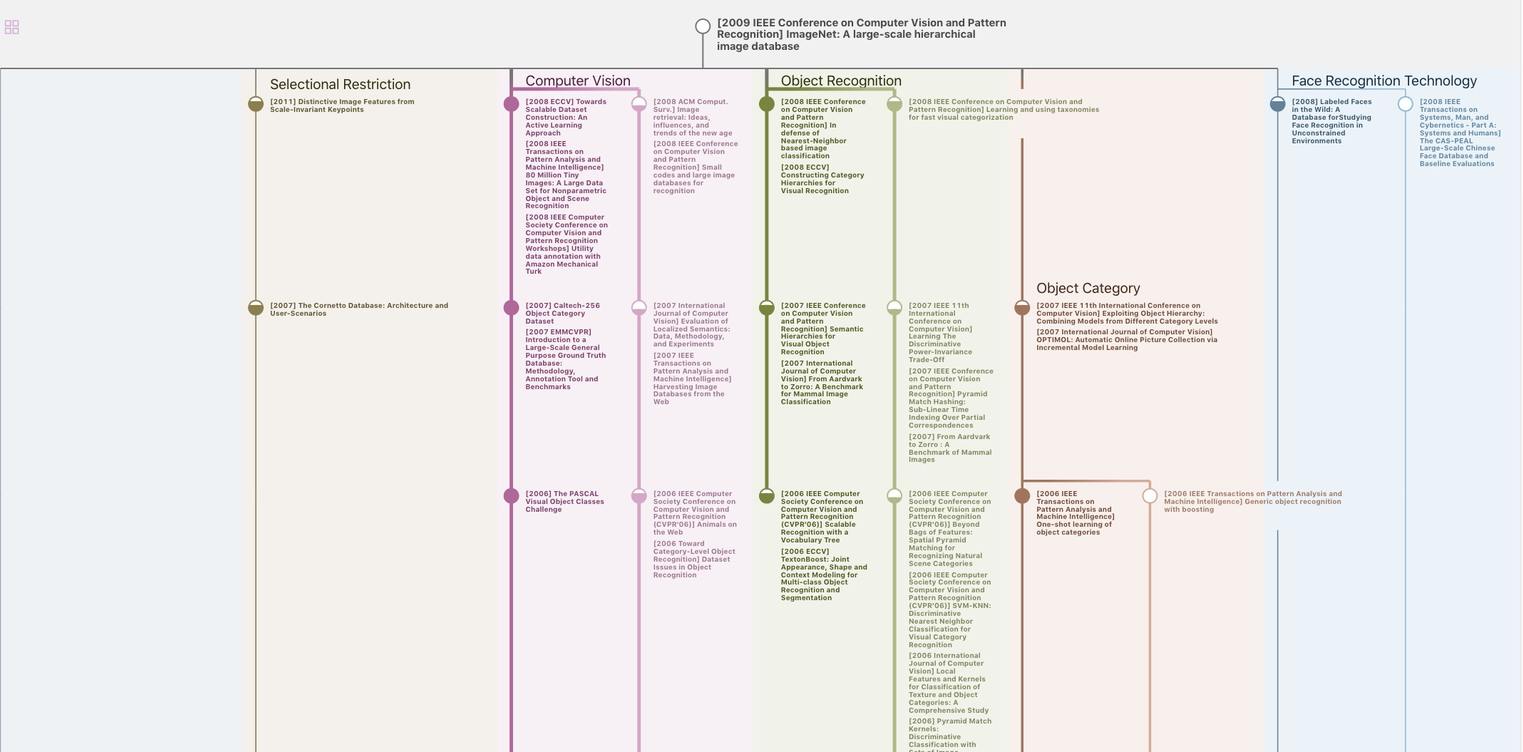
生成溯源树,研究论文发展脉络
Chat Paper
正在生成论文摘要