St-mfnet mini: knowledge distillation-driven frame interpolation
2023 IEEE INTERNATIONAL CONFERENCE ON IMAGE PROCESSING, ICIP(2023)
摘要
Currently, one of the major challenges in deep learning-based video frame interpolation (VFI) is the large model size and high computational complexity associated with many high performance VFI approaches. In this paper, we present a distillation-based two-stage workflow for obtaining compressed VFI models which perform competitively compared to the state of the art, but with significantly reduced model size and complexity. Specifically, an optimisation-based network pruning method is applied to a state of the art frame interpolation model, ST-MFNet, which suffers from large model size. The resulting network architecture achieves a 91% reduction in parameter numbers and a 35% increase in speed. The performance of the new network is further enhanced through a teacher-student knowledge distillation training process using a Laplacian distillation loss. The final low complexity model, ST-MFNet Mini, achieves a comparable performance to most existing high-complexity VFI methods, only outperformed by the original ST-MFNet. Our source code is available at https://github.com/crispianm/ST-MFNet-Mini
更多查看译文
关键词
Video frame interpolation,model compression,knowledge distillation
AI 理解论文
溯源树
样例
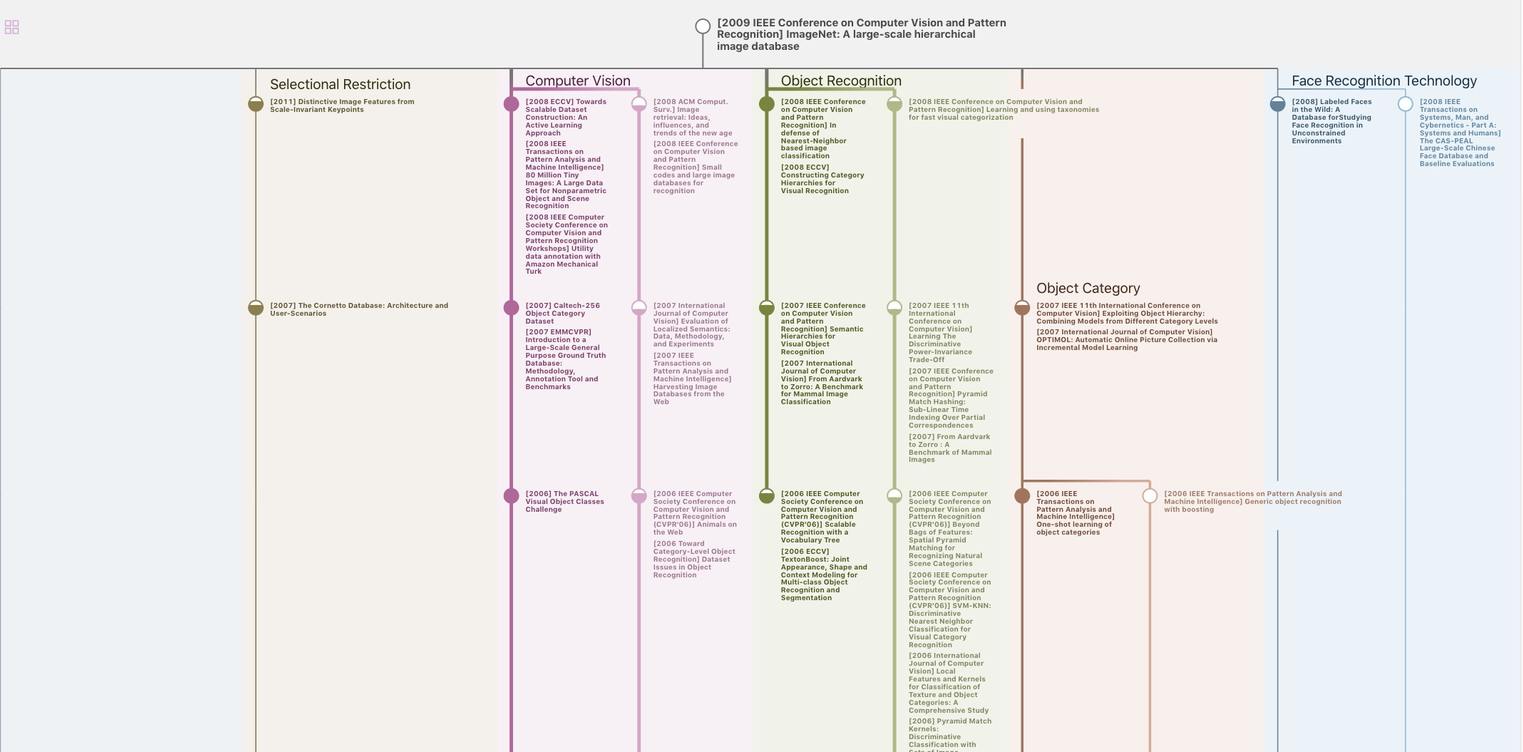
生成溯源树,研究论文发展脉络
Chat Paper
正在生成论文摘要