A Theoretical Formalization of Consequence-Based Decision-Making
biorxiv(2023)
摘要
Learning to make adaptive decisions depends on exploring options, experiencing their consequence, and reassessing one's strategy for the future. Although several studies have analyzed various aspects of value-based decision-making, most of them have focused on decisions in which gratification is cued and immediate. By contrast, how the brain gauges delayed consequence for decision-making remains poorly understood. To investigate this, we designed a novel decision-making task in which each decision altered future options to decide upon. The task was organized in groups of inter-dependent trials, and the participants were instructed to maximize cumulative reward value within each group. In the absence of any explicit performance feedback, the participants had to test and internally assess specific criteria to make decisions. The absence of explicit feedback was key to specifically study how the assessment of consequence forms and influences decisions as learning progresses. We formalized this operation mathematically by means of a multi-layered decision-making model. It uses a mean-field approximation to describe the dynamics of two populations of neurons which characterize the binary decision-making process. The resulting decision-making policy is dynamically modulated by an internal oversight mechanism based on the prediction of consequence. This policy is reinforced by rewarding outcomes. The model was validated by fitting each individual participants' behavior. It faithfully predicted non-trivial patterns of decision-making, regardless of performance level. These findings provide an explanation to how delayed consequence may be computed and incorporated into the neural dynamics of decision-making, and to how adaptation occurs in the absence of explicit feedback.
### Competing Interest Statement
The authors have declared no competing interest.
更多查看译文
关键词
decision-making decision-making,theoretical formalization,consequence-based
AI 理解论文
溯源树
样例
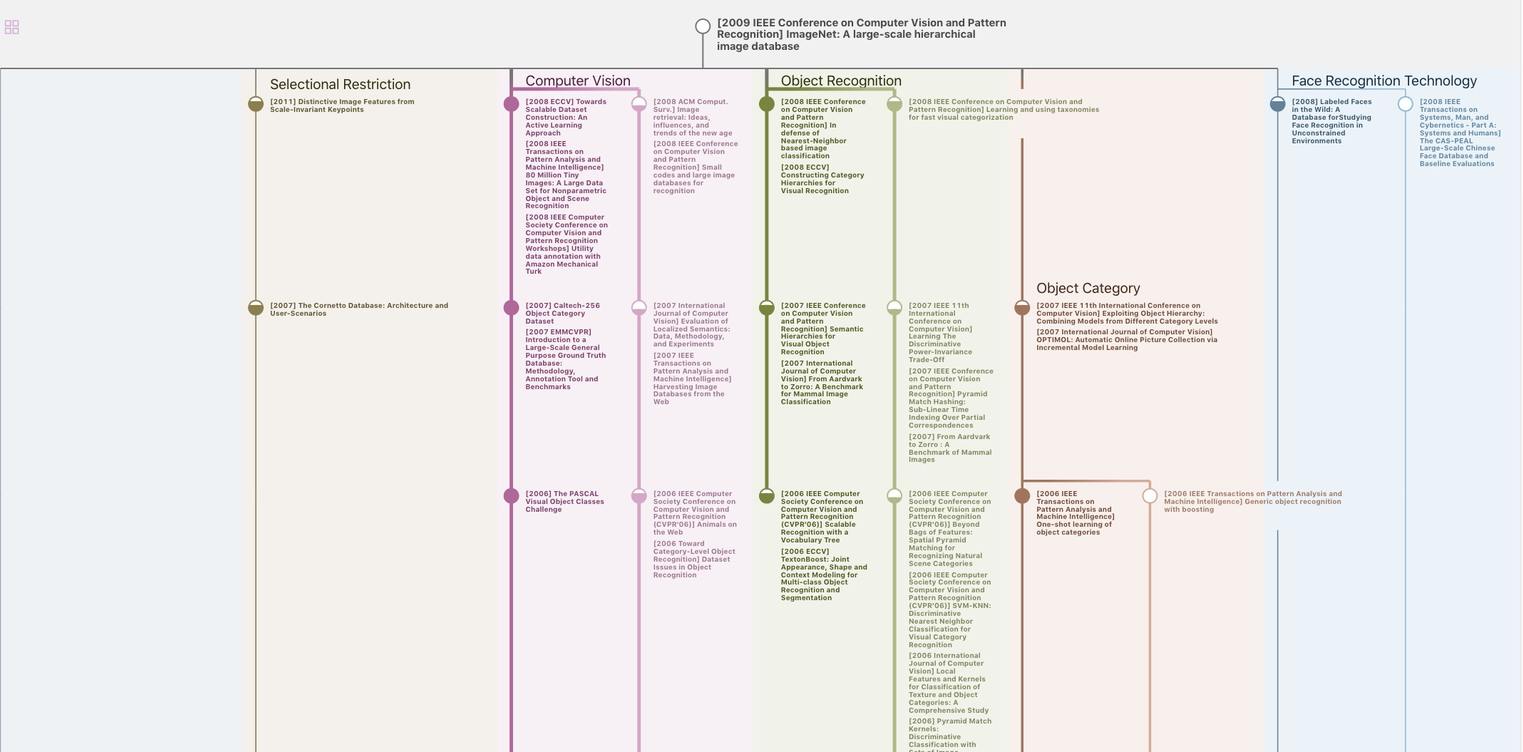
生成溯源树,研究论文发展脉络
Chat Paper
正在生成论文摘要