ACUTE: Attentional Communication Framework for Multi-Agent Reinforcement Learning in Partially Communicable Scenarios
ELECTRONICS(2022)
摘要
Multi-agent reinforcement learning (MARL) aims to study the behavior of multiple agents in a shared environment. Existing communication-based MARL methods seldom consider the case of communication interference. However, such situations are not rare in real-world inter-agent communication. The majority of previous MARL methods struggle to design effective communication techniques for better cooperation between agents without considering communication reliability or channel capacity constraints. In addition, these models are typically not ready to be extended to large-scale multi-agent systems. To address these issues, in this paper, we propose a method named the Attentional CommUnicaTion FramEwork (ACUTE), which enables efficient communication between agents in a dynamic environment and improves the effectiveness of decision-making by using the most useful information from other agents. Specifically, we introduce an attention mechanism for the feature extraction of information during communication which determines the importance of messages received by agents. We evaluate the performance of our approach under different channel capacity constraints. Experimental results show that our model can efficiently exploit messages transmitted in unreliable channels for higher returns when compared to existing methods and can be applied to large-scale multi-agent systems.
更多查看译文
关键词
multi-agent reinforcement learning,partial communication,attention mechanism,large-scale systems
AI 理解论文
溯源树
样例
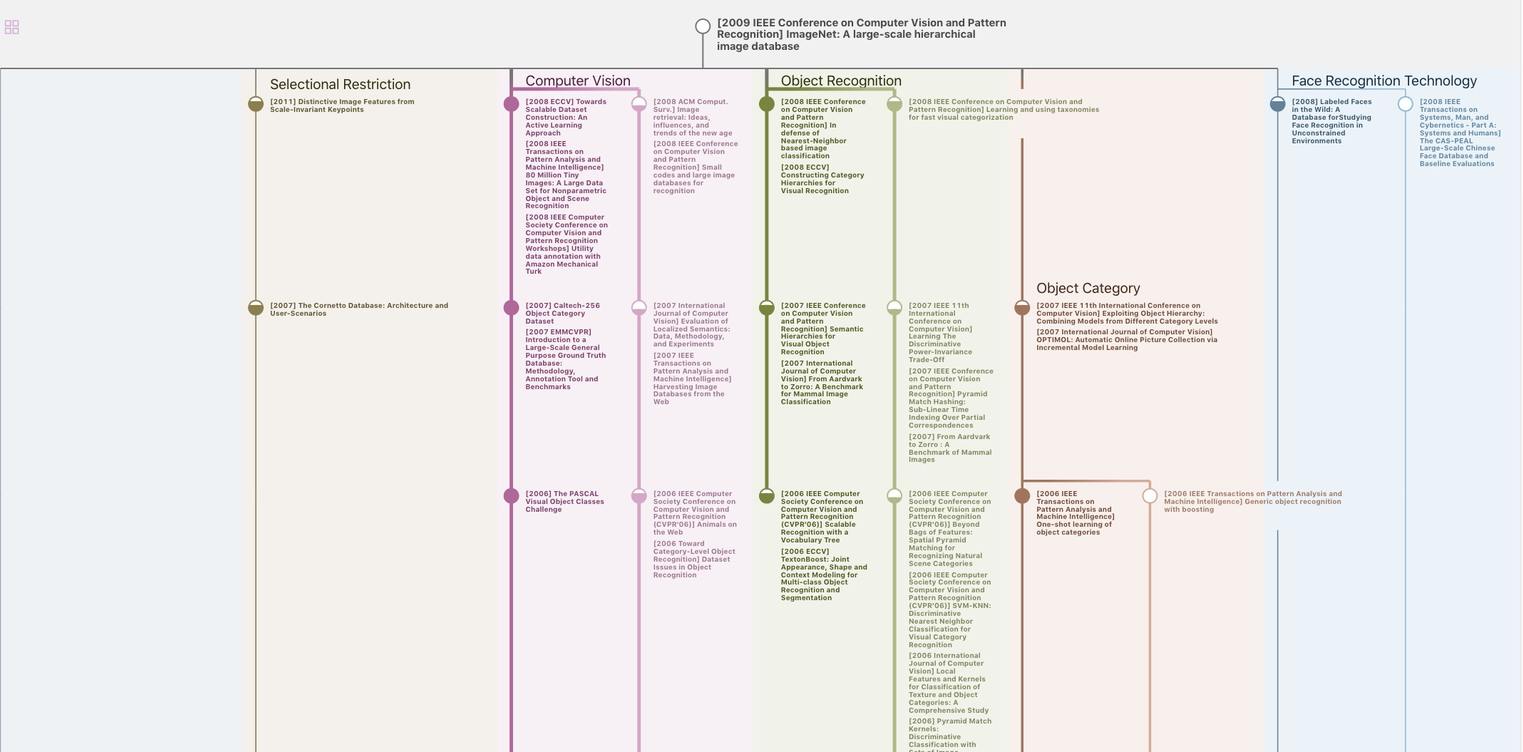
生成溯源树,研究论文发展脉络
Chat Paper
正在生成论文摘要