A Random Forest Approach to Body Motion Detection: Multisensory Fusion and Edge Processing
IEEE Sensors Journal(2023)
摘要
Low-complexity and privacy-respecting human sensing is a challenging task in smart environments as it requires the orchestration of multiple sensors, low-impact machine learning (ML) methods, and resource-constrained Internet of Things (IoT) devices. Client/server-based architectures are typically employed to support sensor fusion. However, these architectures need data to be moved to/from the cloud or data centers, which is contrary to the fundamental requirement of the IoT applications to limit costs, complexity, memory footprint, processing, and communication resources. In this article, we propose the design and implementation of an integrated edge device targeting human sensing for indoor smart spaces applications envisioned in Industry 5.0 applications. The proposed device implements the cumulative sum (CUSUM) method for data distillation from multiple sensors and adopts a low-complexity random forest algorithm (RFA) to sense and classify body movements: in particular, the device integrates both infrared (IR) and ultrasonic (US) sensors. This article discusses the benefits of the combined use of CUSUM and RFA methods against classical ML approaches in terms of accuracy, complexity, computing time, and storage. The proposed architecture and processing steps are validated experimentally by targeting the fall detection problem in a smart space environment. RFA reduces the complexity by at least three times compared to classical ML tools based on the analysis of space and time features (convolutional neural networks and long short-term memory): processing time is in the order of 0.1 s while accuracy is about 94%.
更多查看译文
关键词
Edge processing,fall detection,Internet of Things (IoT),machine learning (ML),random forest,sensor fusion
AI 理解论文
溯源树
样例
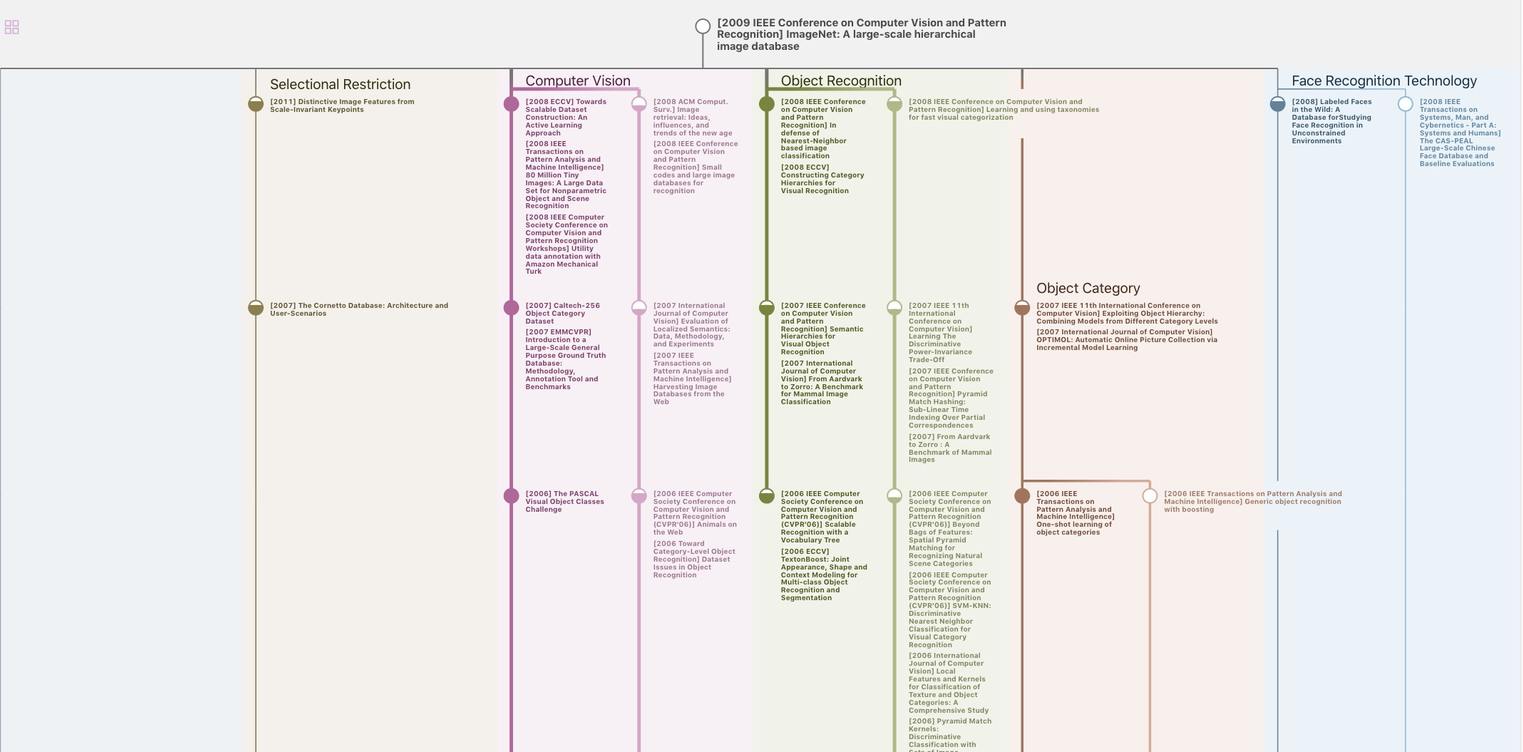
生成溯源树,研究论文发展脉络
Chat Paper
正在生成论文摘要