SLIM: Sparsified Late Interaction for Multi-Vector Retrieval with Inverted Indexes
PROCEEDINGS OF THE 46TH INTERNATIONAL ACM SIGIR CONFERENCE ON RESEARCH AND DEVELOPMENT IN INFORMATION RETRIEVAL, SIGIR 2023(2023)
摘要
This paper introduces Sparsified Late Interaction for Multi-vector (SLIM) retrieval with inverted indexes. Multi-vector retrieval methods have demonstrated their effectiveness on various retrieval datasets, and among them, ColBERT is the most established method based on the late interaction of contextualized token embeddings of pre-trained language models. However, efficient ColBERT implementations require complex engineering and cannot take advantage of off-the-shelf search libraries, impeding their practical use. To address this issue, SLIM first maps each contextualized token vector to a sparse, high-dimensional lexical space before performing late interaction between these sparse token embeddings. We then introduce an efficient two-stage retrieval architecture that includes inverted index retrieval followed by a score refinement module to approximate the sparsified late interaction, which is fully compatible with off-the-shelf lexical search libraries such as Lucene. SLIM achieves competitive accuracy on MS MARCO Passages and BEIR compared to ColBERT while being much smaller and faster on CPUs. To our knowledge, we are the first to explore using sparse token representations for multi-vector retrieval. Source code and data are integrated into the Pyserini IR toolkit.
更多查看译文
关键词
Neural IR,Late Interaction,Inverted Indexes,Sparse Retrieval
AI 理解论文
溯源树
样例
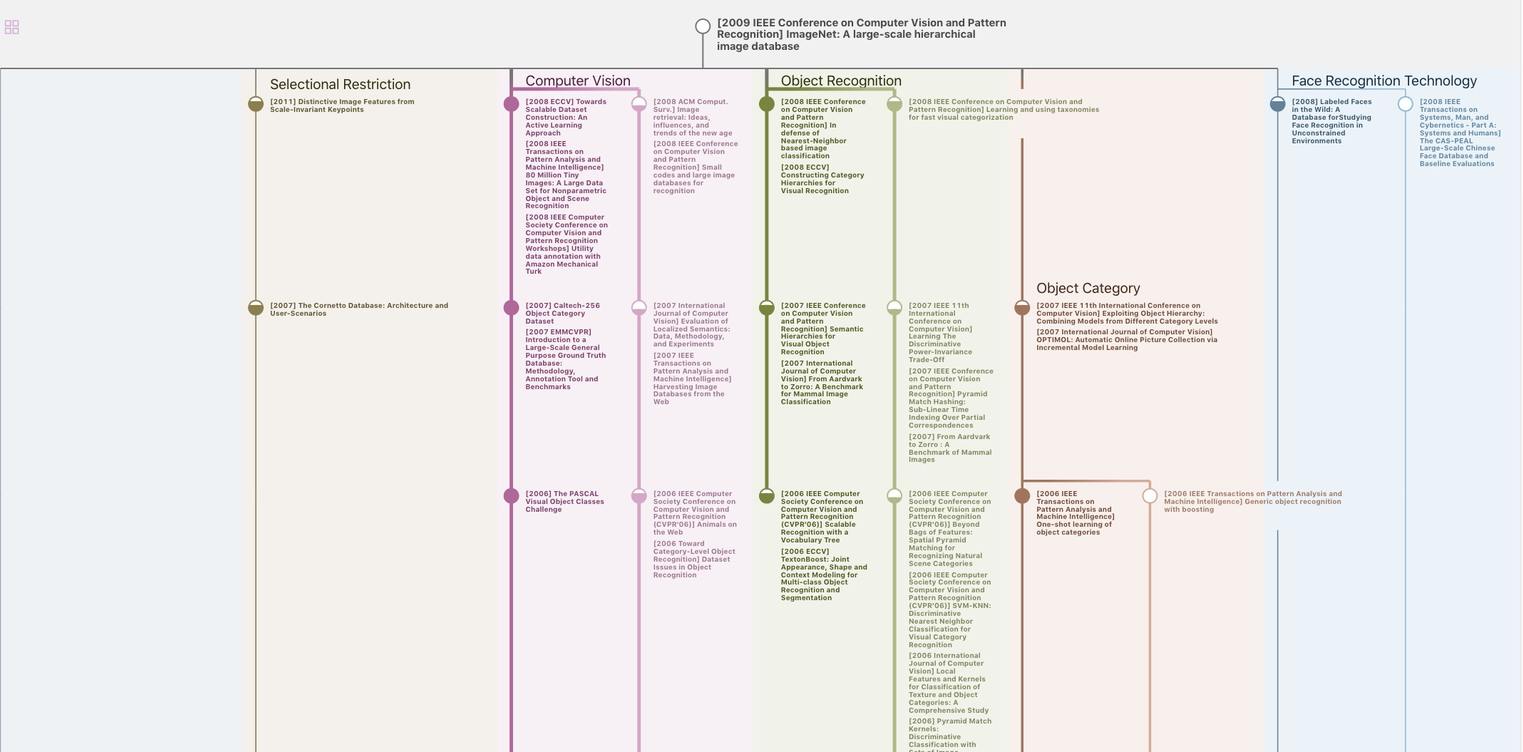
生成溯源树,研究论文发展脉络
Chat Paper
正在生成论文摘要