Probabilistic or Convolutional-LSTM neuronal networks: a comparative study of discrimination capacity on frozen - thawed fish fillets
2022 11th International Conference On Software Process Improvement (CIMPS)(2022)
摘要
The quality and safety of frozen-refrigerated hydro- biological products depend on the efficiency of the cold chain; therefore, there is growing interest in the discrimination of products subjected to inefficient cold chains, thus avoiding food poisoning and loss of products. For this purpose; computer vision systems coupled with chemometric tools, such as convolutional neural networks (CNN Convolutional Neural Network), have shown potential in discrimination tasks of three-band (RGB) images. Nevertheless; multi-band images, as hyperspectral images, require specialized convolutional networks such as those using LSTM (Large Short-Term Memory) layers. This paper compares radial-based artificial neural networks and LSTM convolutional networks for discrimination of fish fillets subject to freeze-thaw cycles. Fresh fish samples were filleted and subsequently potted to two freezing-thawing cycles (FTC). Then, hyperspectral images in the range of 400 to 1000 nm were acquired from fresh samples after each FTC. Subsequently, twenty spectral profiles of each of the fillets were extracted and pre-treated (smoothed); from these profiles, classification models were built using radial basis neural networks (RBNN - radial basis neuronal network) and CNN-LSTM. Finally, the metrics of each model were calculated, summarizing these in the value F-measure. The CNN-LSTM presented F-measure =3D 0.9075 ± 0.011, value relatively higher than the 0.8509 ± 0.003 of the RBNN. So, for discrimination of mackerel fillets subjected to different CCD, the CNN-LSTM shown to be better to the RBNN.
更多查看译文
关键词
RBNN,LSTM,Convolution,neural network,discrimination,food quality
AI 理解论文
溯源树
样例
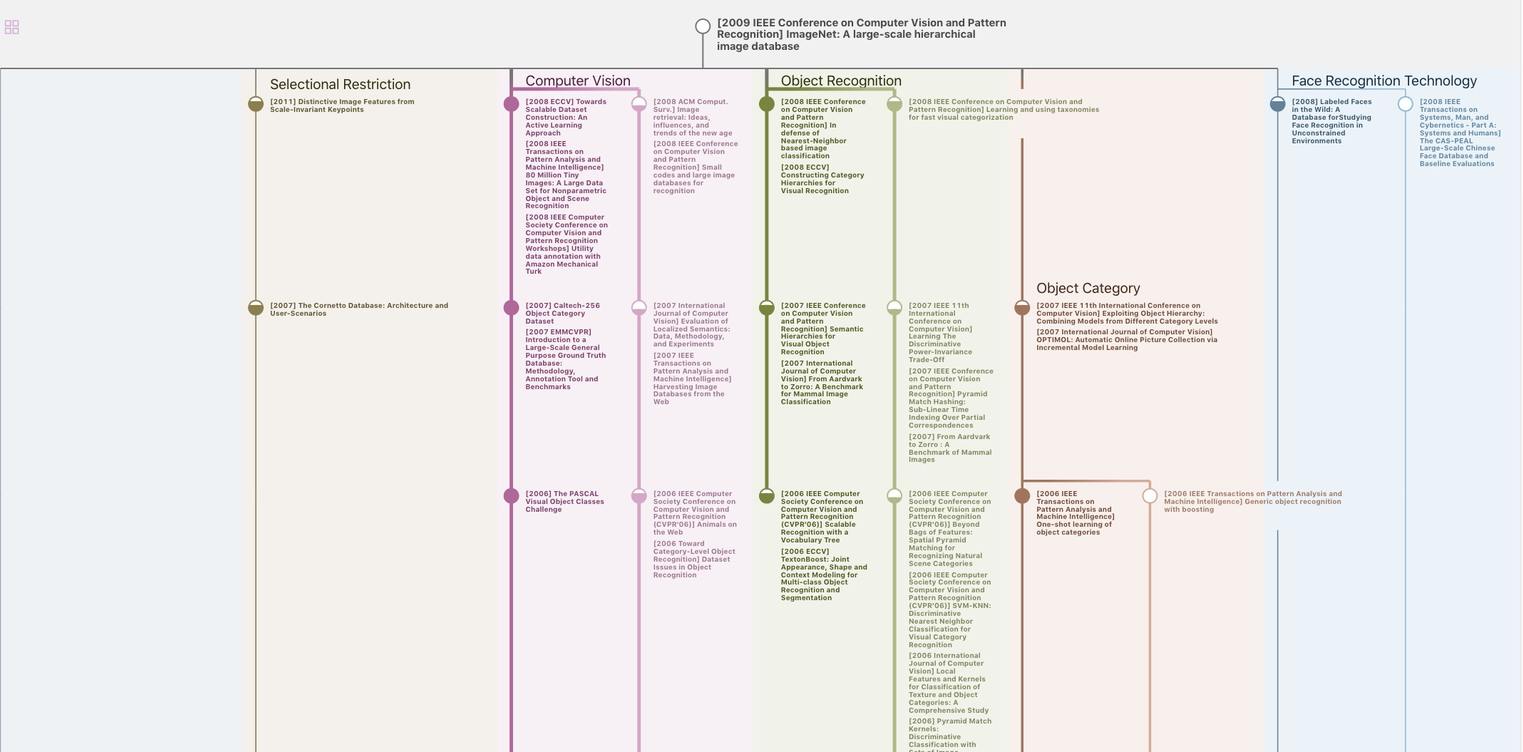
生成溯源树,研究论文发展脉络
Chat Paper
正在生成论文摘要