Machine learning detects multiplicity of the first stars in stellar archaeology data
The Astrophysical Journal(2023)
摘要
In unveiling the nature of the first stars, the main astronomical clue is the elemental compositions of the second generation of stars, observed as extremely metal-poor (EMP) stars, in our Milky Way Galaxy. However, no observational constraint was available on their multiplicity, which is crucial for understanding early phases of galaxy formation. We develop a new data-driven method to classify observed EMP stars into mono- or multi-enriched stars with Support Vector Machines. We also use our own nucleosynthesis yields of core-collapse supernovae with mixing-fallback that can explain many of observed EMP stars. Our method predicts, for the first time, that $31.8\% \pm 2.3\%$ of 462 analyzed EMP stars are classified as mono-enriched. This means that the majority of EMP stars are likely multi-enriched, suggesting that the first stars were born in small clusters. Lower metallicity stars are more likely to be enriched by a single supernova, most of which have high carbon enhancement. We also find that Fe, Mg. Ca, and C are the most informative elements for this classification. In addition, oxygen is very informative despite its low observability. Our data-driven method sheds a new light on solving the mystery of the first stars from the complex data set of Galactic archaeology surveys.
更多查看译文
关键词
Population III stars,Population II stars,Milky Way formation,Support vector machine
AI 理解论文
溯源树
样例
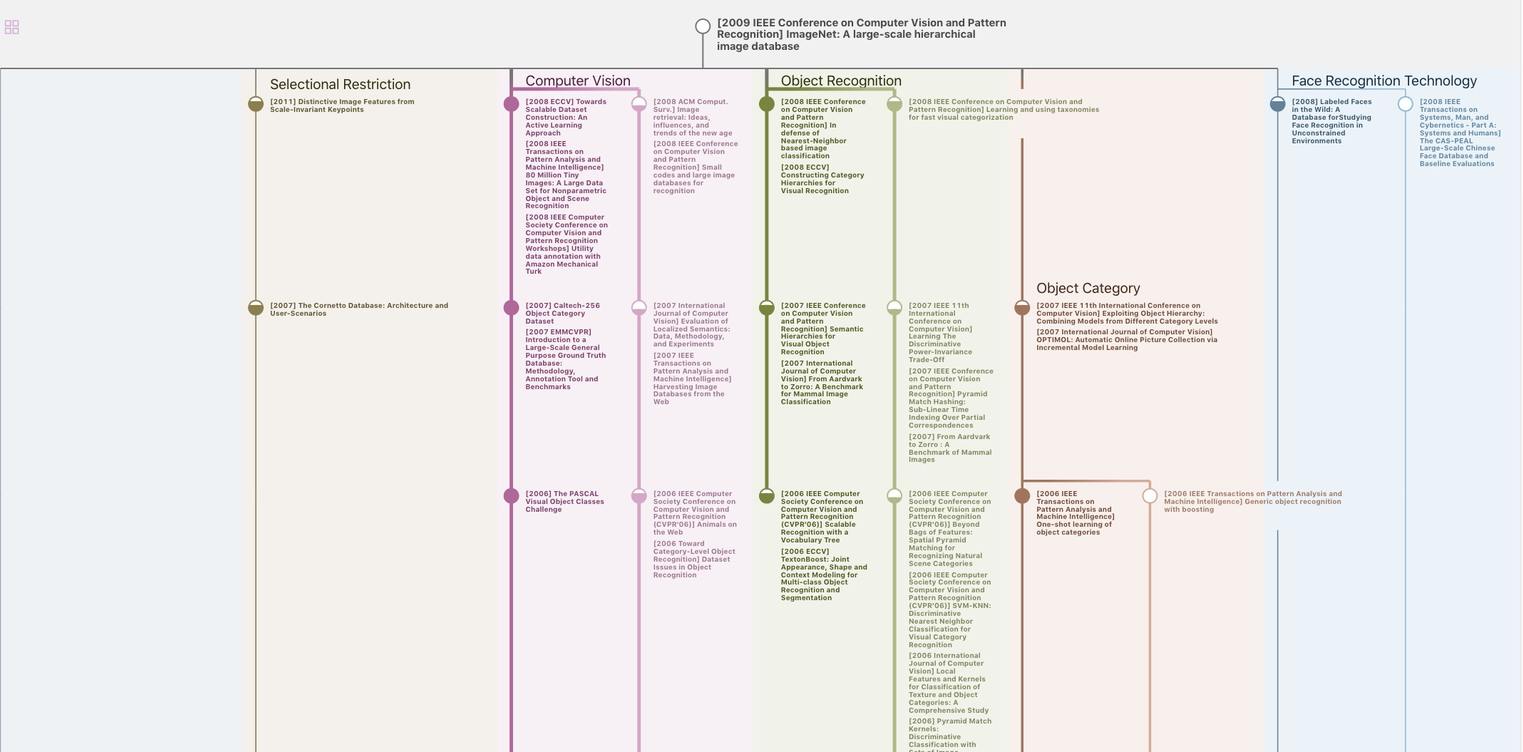
生成溯源树,研究论文发展脉络
Chat Paper
正在生成论文摘要