Unified Detection of Digital and Physical Face Attacks
2023 IEEE 17th International Conference on Automatic Face and Gesture Recognition (FG)(2023)
Abstract
State-of-the-art defense mechanisms against face attacks achieve near perfect accuracies within one of three attack categories, namely adversarial, digital manipulation, or physical spoofs, however, they fail to generalize well when tested across all three categories. Poor generalization can be attributed to learning incoherent attacks jointly. To over-come this shortcoming, we propose a unified attack detection framework, namely UniFAD, that can automatically cluster 25 coherent attack types belonging to the three categories. Using a multi-task learning framework along with k-means clustering, UniFAD learns joint representations for coherent attacks, while uncorrelated attack types are learned separately. Proposed UniFAD outperforms prevailing defense methods and their fusion with an overall TDR = 94.73% @ 0.2% FDR on a large fake face dataset consisting of 341K bona fide images and 448K attack images of 25 types across all 3 categories. Proposed method can detect an attack within 3 milliseconds on a Nvidia 2080Ti. UniFAD can also identify the attack categories with 97.37% accuracy. Code and dataset will be publicly available.
MoreTranslated text
AI Read Science
Must-Reading Tree
Example
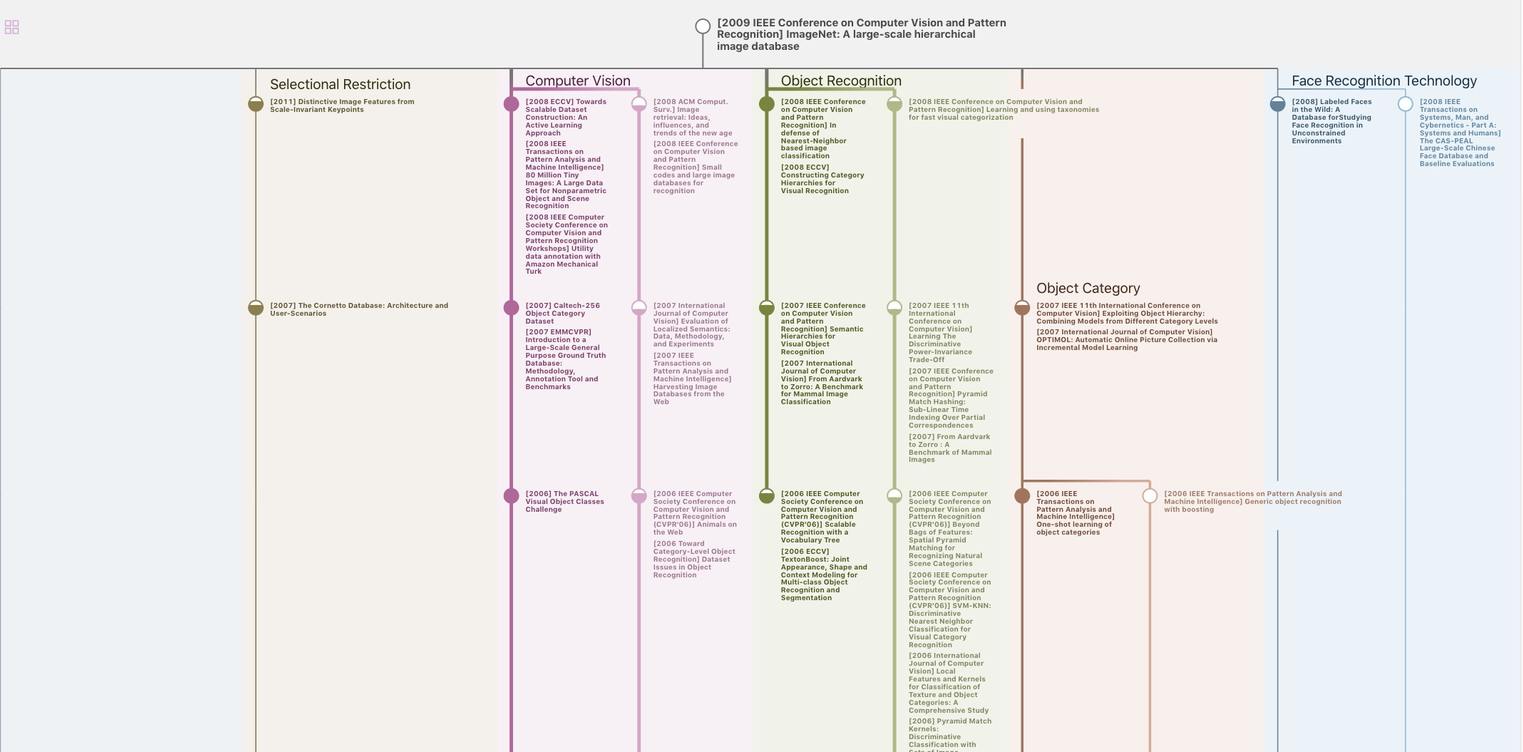
Generate MRT to find the research sequence of this paper
Chat Paper
Summary is being generated by the instructions you defined