MFR-DTA: a multi-functional and robust model for predicting drug-target binding affinity and region
BIOINFORMATICS(2023)
摘要
Motivation: Recently, deep learning has become the mainstream methodology for drug-target binding affinity prediction. However, two deficiencies of the existing methods restrict their practical applications. On the one hand, most existing methods ignore the individual information of sequence elements, resulting in poor sequence feature representations. On the other hand, without prior biological knowledge, the prediction of drug-target binding regions based on attention weights of a deep neural network could be difficult to verify, which may bring adverse interference to biological researchers. Results: We propose a novel Multi-Functional and Robust Drug-Target binding Affinity prediction (MFR-DTA) method to address the above issues. Specifically, we design a new biological sequence feature extraction block, namely BioMLP, that assists the model in extracting individual features of sequence elements. Then, we propose a new Elem-feature fusion block to refine the extracted features. After that, we construct a Mix-Decoder block that extracts drug-target interaction information and predicts their binding regions simultaneously. Last, we evaluate MFR-DTA on two benchmarks consistently with the existing methods and propose a new dataset, sc-PDB, to better measure the accuracy of binding region prediction. We also visualize some samples to demonstrate the locations of their binding sites and the predicted multi-scale interaction regions. The proposed method achieves excellent performance on these datasets, demonstrating its merits and superiority over the state-of-the-art methods. Availability and implementation: https://github.com/JU-HuaY/MFR. Contact: x.song@jiangnan.edu.cn or z.feng@surrey.ac.uk
更多查看译文
AI 理解论文
溯源树
样例
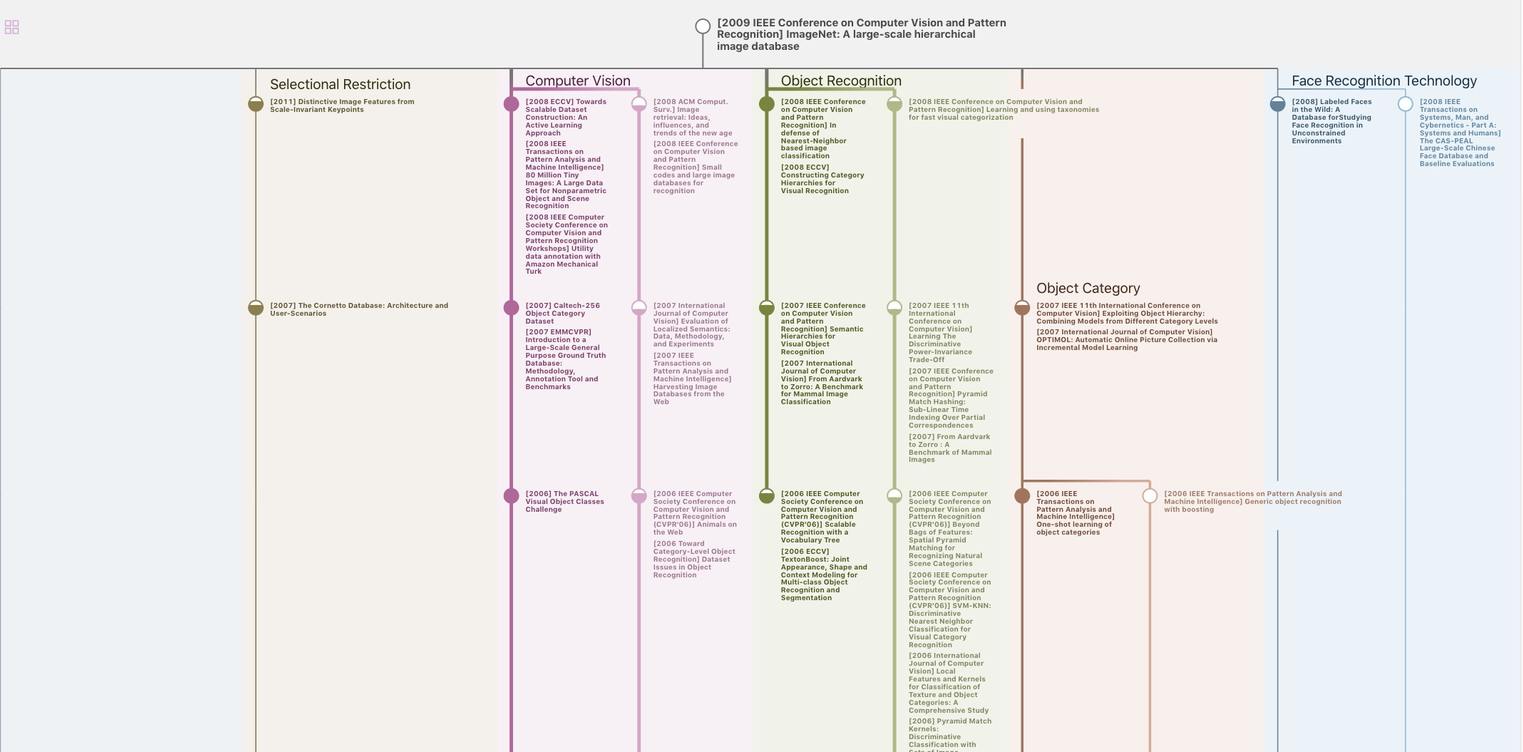
生成溯源树,研究论文发展脉络
Chat Paper
正在生成论文摘要