SSR-2D: Semantic 3D Scene Reconstruction from 2D Images
CoRR(2023)
Abstract
Most deep learning approaches to comprehensive semantic modeling of 3D indoor spaces require costly dense annotations in the 3D domain. In this work, we explore a central 3D scene modeling task, namely, semantic scene reconstruction without using any 3D annotations. The key idea of our approach is to design a trainable model that employs both incomplete 3D reconstructions and their corresponding source RGB-D images, fusing cross-domain features into volumetric embeddings to predict complete 3D geometry, color, and semantics with only 2D labeling which can be either manual or machine-generated. Our key technical innovation is to leverage differentiable rendering of color and semantics to bridge 2D observations and unknown 3D space, using the observed RGB images and 2D semantics as supervision, respectively. We additionally develop a learning pipeline and corresponding method to enable learning from imperfect predicted 2D labels, which could be additionally acquired by synthesizing in an augmented set of virtual training views complementing the original real captures, enabling more efficient self-supervision loop for semantics. In this work, we propose an end-to-end trainable solution jointly addressing geometry completion, colorization, and semantic mapping from limited RGB-D images, without relying on any 3D ground-truth information. Our method achieves state-of-the-art performance of semantic scene reconstruction on two large-scale benchmark datasets MatterPort3D and ScanNet, surpasses baselines even with costly 3D annotations. To our knowledge, our method is also the first 2D-driven method addressing completion and semantic segmentation of real-world 3D scans.
MoreTranslated text
Key words
semantic 3d scene reconstruction,images
AI Read Science
Must-Reading Tree
Example
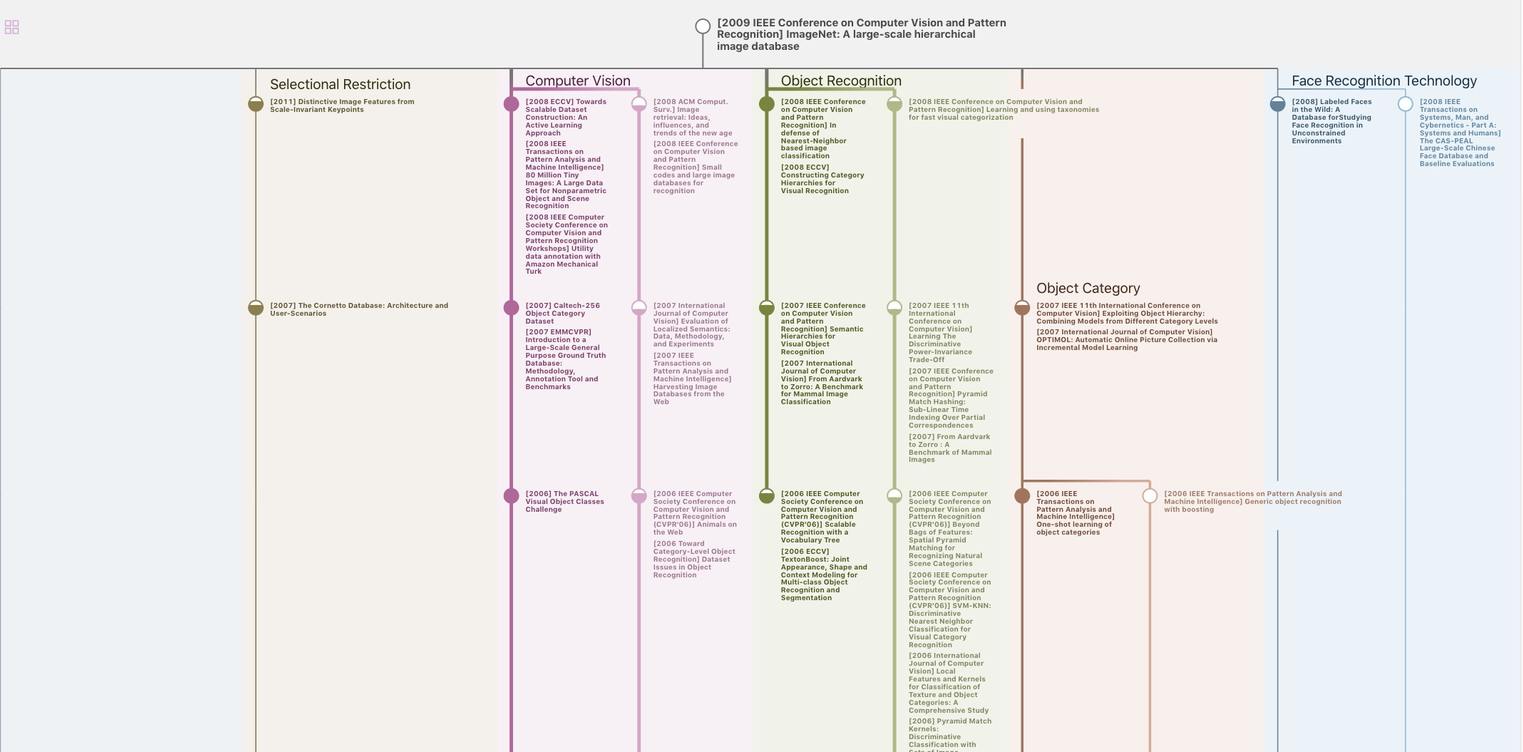
Generate MRT to find the research sequence of this paper
Chat Paper
Summary is being generated by the instructions you defined