UDAPTER - Efficient Domain Adaptation Using Adapters
17TH CONFERENCE OF THE EUROPEAN CHAPTER OF THE ASSOCIATION FOR COMPUTATIONAL LINGUISTICS, EACL 2023(2023)
摘要
We propose two methods to make unsupervised domain adaptation ( UDA) more parameter efficient using adapters, small bottleneck layers interspersed with every layer of the largescale pre-trained language model (PLM). The first method deconstructs UDA into a two-step process: first by adding a domain adapter to learn domain-invariant information and then by adding a task adapter that uses domaininvariant information to learn task representations in the source domain. The second method jointly learns a supervised classifier while reducing the divergence measure. Compared to strong baselines, our simple methods perform well in natural language inference (MNLI) and the cross-domain sentiment classification task. We even outperform unsupervised domain adaptation methods such as DANN (Ganin et al., 2016) and DSN (Bousmalis et al., 2016) in sentiment classification, and we are within 0.85% F1 for natural language inference task, by fine-tuning only a fraction of the full model parameters. We release our code at https://github.com/declare-lab/domadapter.
更多查看译文
AI 理解论文
溯源树
样例
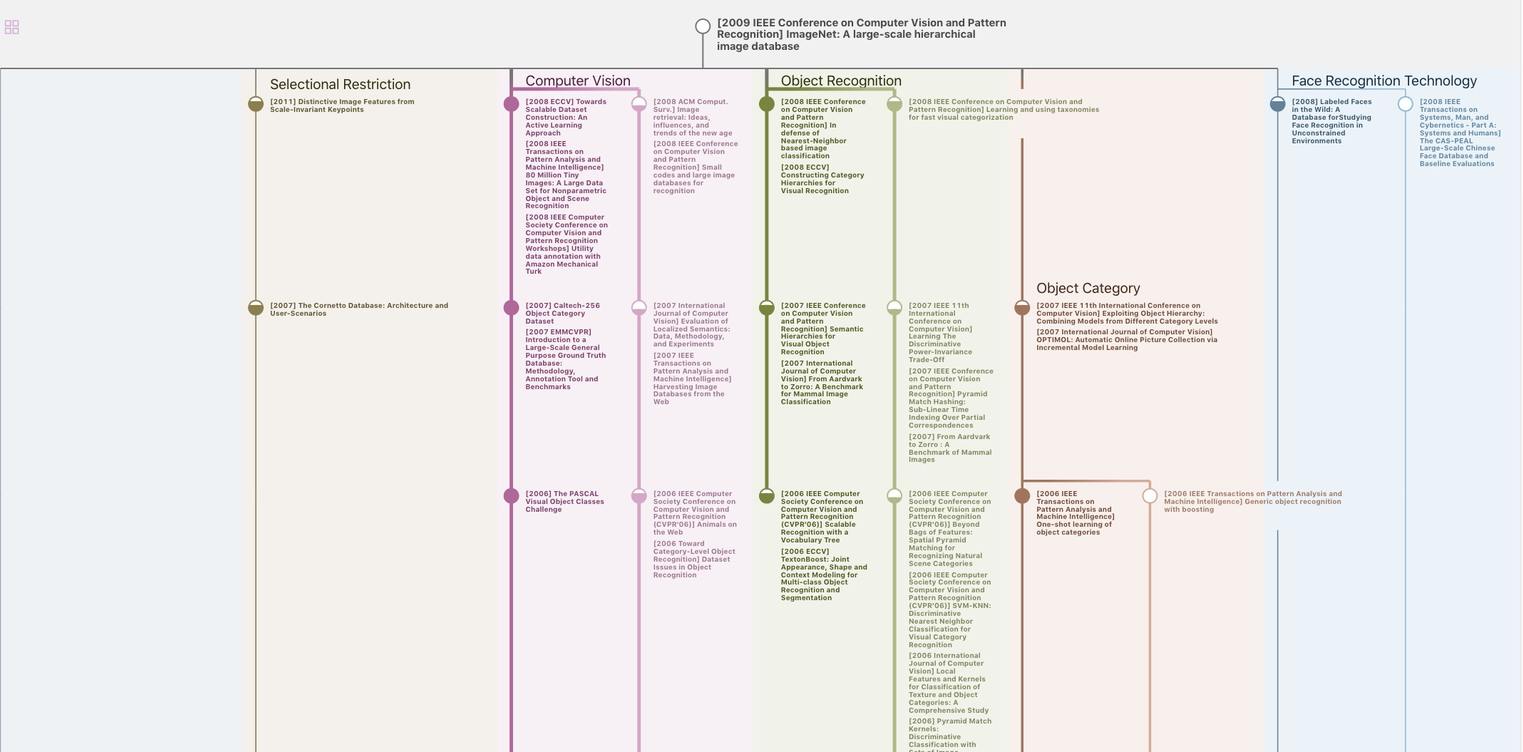
生成溯源树,研究论文发展脉络
Chat Paper
正在生成论文摘要