LaMPP: Language Models as Probabilistic Priors for Perception and Action
CoRR(2023)
摘要
Language models trained on large text corpora encode rich distributional information about real-world environments and action sequences. This information plays a crucial role in current approaches to language processing tasks like question answering and instruction generation. We describe how to leverage language models for *non-linguistic* perception and control tasks. Our approach casts labeling and decision-making as inference in probabilistic graphical models in which language models parameterize prior distributions over labels, decisions and parameters, making it possible to integrate uncertain observations and incomplete background knowledge in a principled way. Applied to semantic segmentation, household navigation, and activity recognition tasks, this approach improves predictions on rare, out-of-distribution, and structurally novel inputs.
更多查看译文
关键词
language models,probabilistic priors,perception
AI 理解论文
溯源树
样例
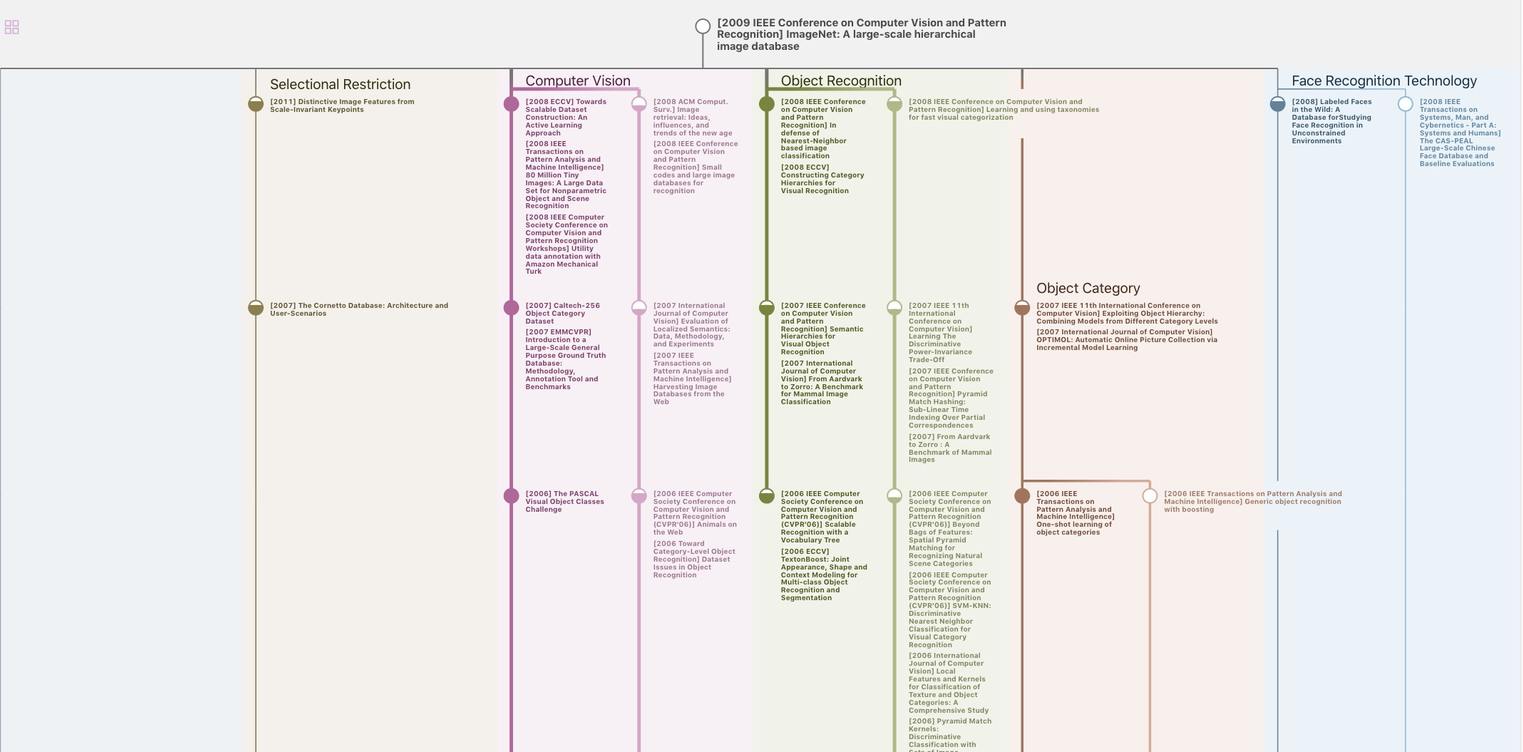
生成溯源树,研究论文发展脉络
Chat Paper
正在生成论文摘要