LipFormer: Learning to Lipread Unseen Speakers based on Visual-Landmark Transformers
arxiv(2023)
摘要
Lipreading refers to understanding and further translating the speech of a speaker in the video into natural language. State-of-the-art lipreading methods excel in interpreting overlap speakers, i.e., speakers appear in both training and inference sets. However, generalizing these methods to unseen speakers incurs catastrophic performance degradation due to the limited number of speakers in training bank and the evident visual variations caused by the shape/color of lips for different speakers. Therefore, merely depending on the visible changes of lips tends to cause model overfitting. To address this problem, we propose to use multi-modal features across visual and landmarks, which can describe the lip motion irrespective to the speaker identities. Then, we develop a sentence-level lipreading framework based on visual-landmark transformers, namely LipFormer. Specifically, LipFormer consists of a lip motion stream, a facial landmark stream, and a cross-modal fusion. The embeddings from the two streams are produced by self-attention, which are fed to the cross-attention module to achieve the alignment between visuals and landmarks. Finally, the resulting fused features can be decoded to output texts by a cascade seq2seq model. Experiments demonstrate that our method can effectively enhance the model generalization to unseen speakers.
更多查看译文
关键词
lipread unseen speakers,lipformer,learning,visual-landmark
AI 理解论文
溯源树
样例
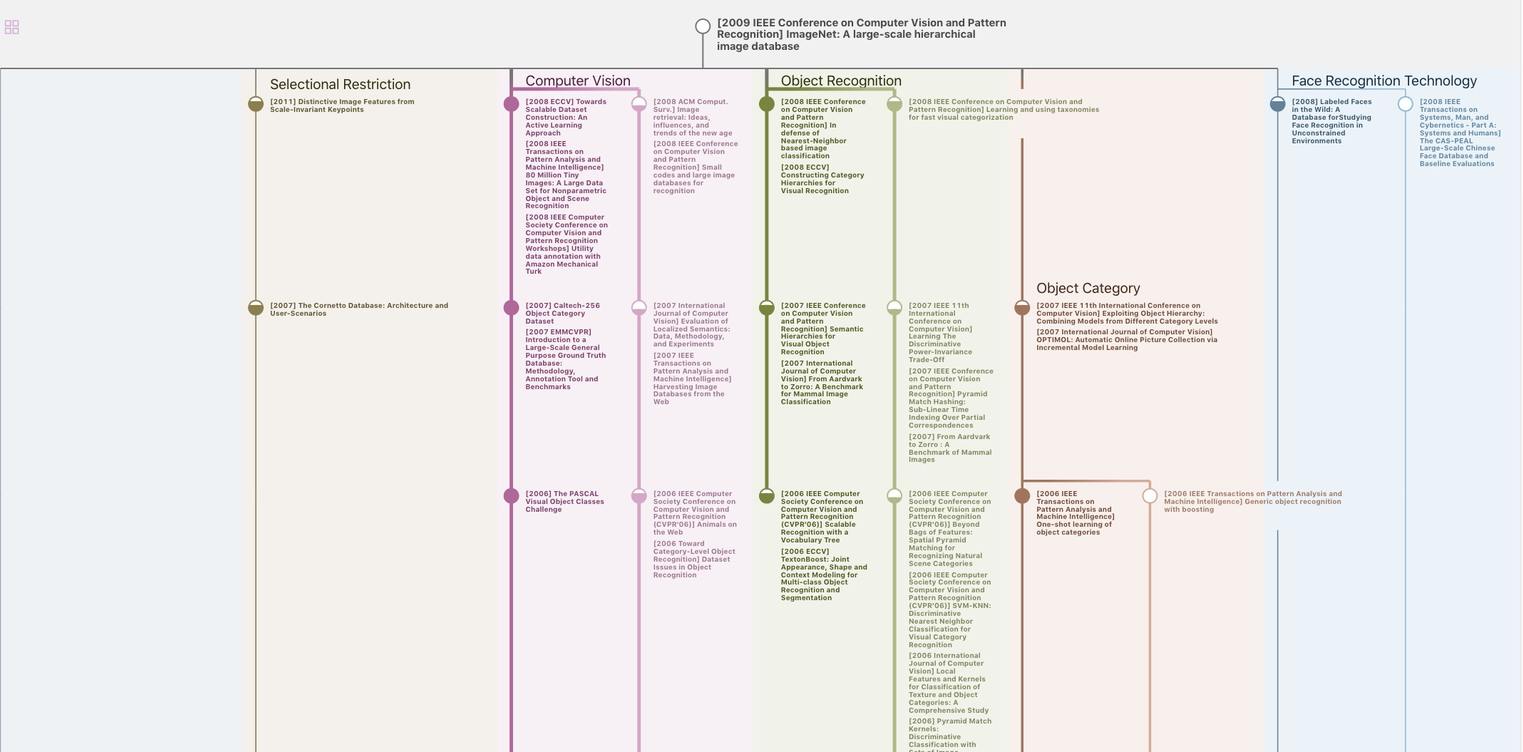
生成溯源树,研究论文发展脉络
Chat Paper
正在生成论文摘要