Category Knowledge-Guided Parameter Calibration for Few-Shot Object Detection
IEEE Transactions on Image Processing(2023)
摘要
Few-shot object detection (FSOD) aims to adapt generic detectors to the novel categories with only a few annotations, which is an important and realistic task. Although the generic object detection has been widely studied over the past years, the FSOD is under explored. In this paper, we propose a novel Category Knowledge-guided Parameter Calibration (CKPC) framework to solve the FSOD task. We first propagate the category relation information to explore the representative category knowledge. Then, we explore the RoI-RoI and RoI-Category relations to capture the local-global context information to enhance the RoI (Region of Interest) features. Next, we project the knowledge representations of foreground categories into a parameter space by a linear transformation to generate the parameters of the category-level classifier. For the background, we learn a proxy category by concluding the global characteristics of all foreground categories to help ensure the discrepancy between the foreground and background, which is then projected into the parameter space by the same linear transformation. Finally, we leverage the parameters of the category-level classifier to explicitly calibrate the instance-level classifier learned on the enhanced RoI features for both the foreground and background categories to improve the detection performance. We conduct extensive experiments on two popular FSOD benchmarks (i.e., Pascal VOC and MS COCO), and the experimental results show that the proposed framework can outperform state-of-the-art methods.
更多查看译文
关键词
Object detection,few-shot learning,graph neural network
AI 理解论文
溯源树
样例
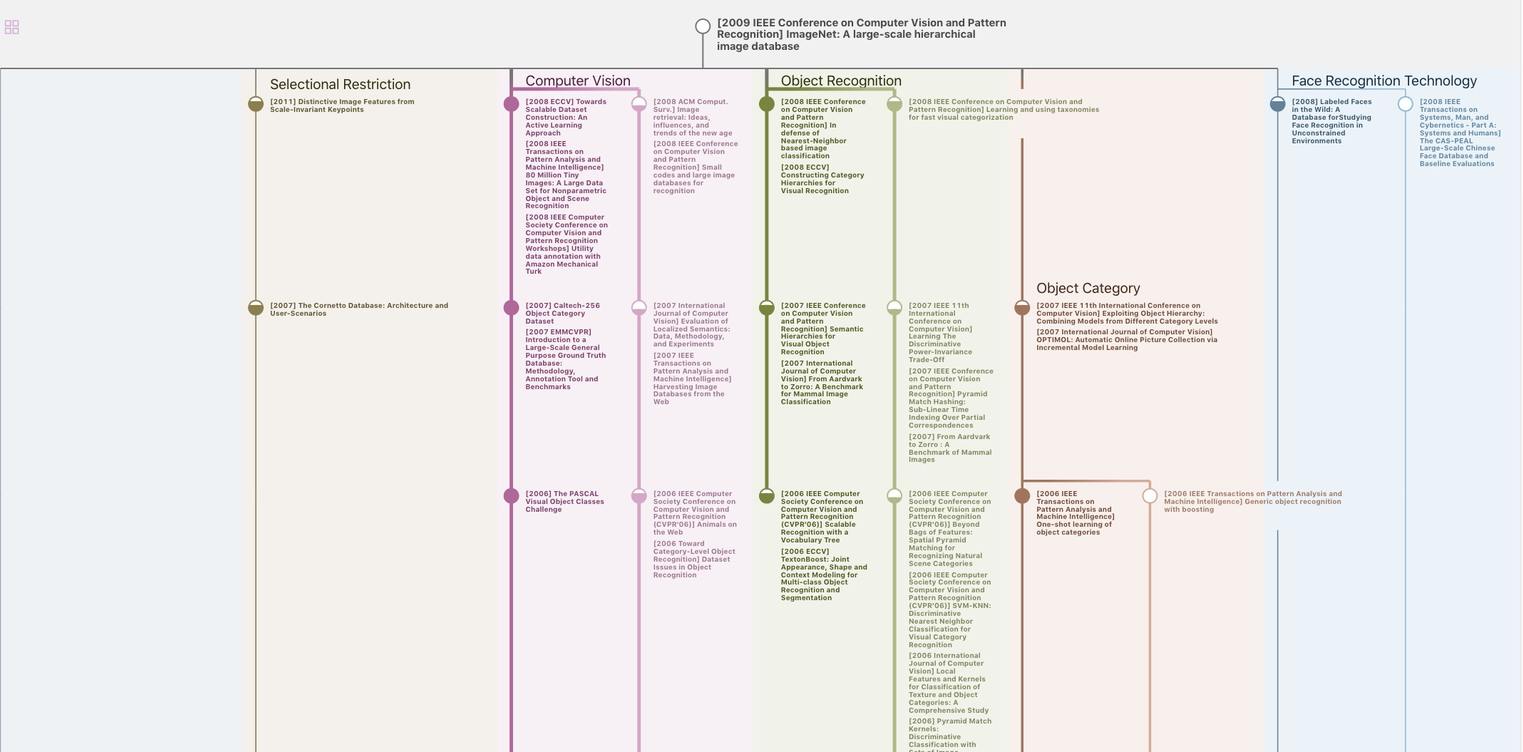
生成溯源树,研究论文发展脉络
Chat Paper
正在生成论文摘要