DAG Matters! GFlowNets Enhanced Explainer for Graph Neural Networks
ICLR 2023(2023)
摘要
Uncovering rationales behind predictions of graph neural networks (GNNs) has received increasing attention over the years. Existing literature mainly focus on selecting a subgraph, through combinatorial optimization, to provide faithful explanations. However, the exponential size of candidate subgraphs limits the applicability of state-of-the-art methods to large-scale GNNs. We enhance on this through a different approach: by proposing a generative structure – GFlowNets-based GNN Explainer (GFlowExplainer), we turn the optimization problem into a step-by-step generative problem. Our GFlowExplainer aims to learn a policy that generates a distribution of subgraphs for which the probability of a subgraph is proportional to its’ reward. The proposed approach eliminates the influence of node sequence and thus does not need any pre-training strategies. We also propose a new cut vertex matrix to efficiently explore parent states for GFlowNets structure, thus making our approach applicable in a large-scale setting. We conduct extensive experiments on both synthetic and real datasets, and both qualitative and quantitative results show the superiority of our GFlowExplainer.
更多查看译文
关键词
GNN,Interpretability
AI 理解论文
溯源树
样例
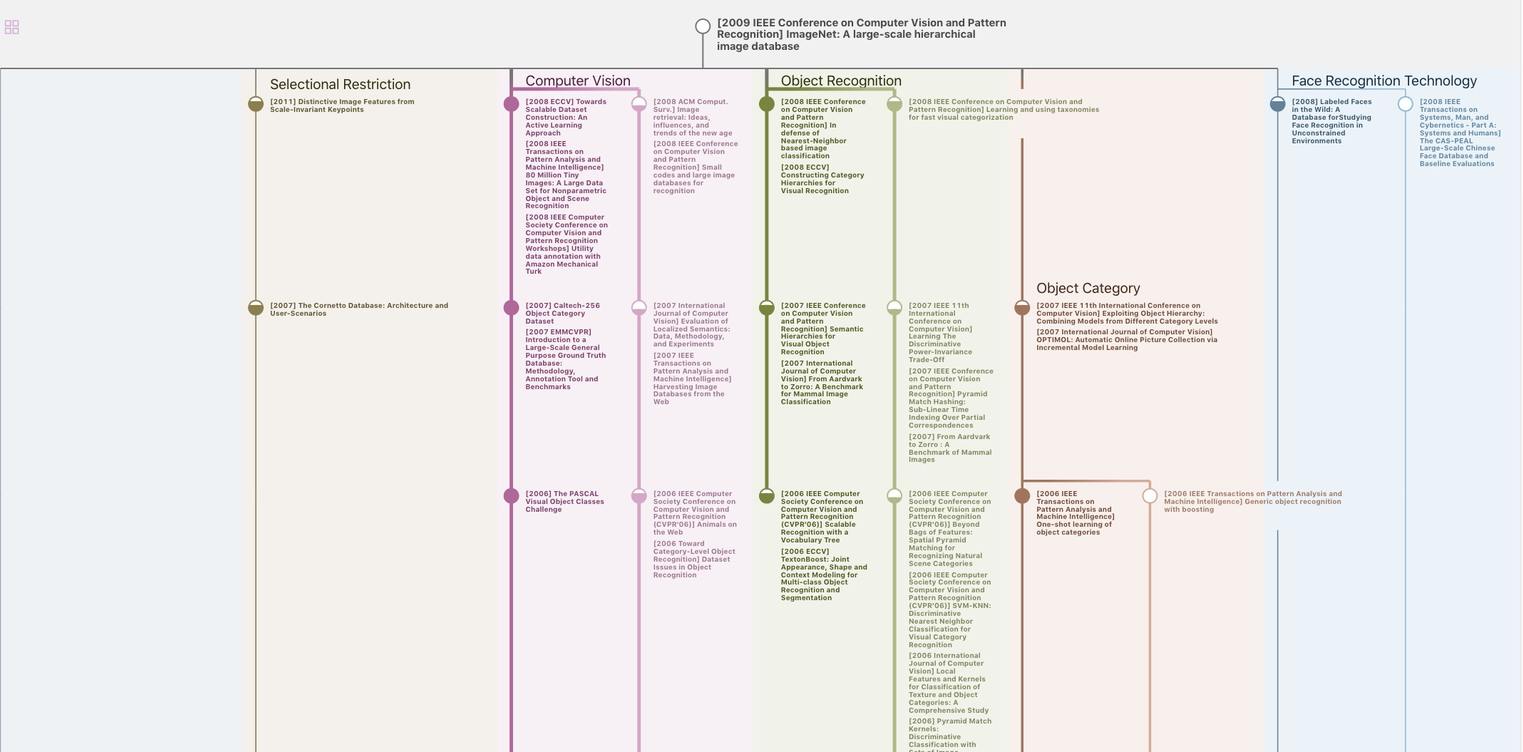
生成溯源树,研究论文发展脉络
Chat Paper
正在生成论文摘要