ROCO: A General Framework for Evaluating Robustness of Combinatorial Optimization Solvers on Graphs
ICLR 2023(2023)
摘要
Solving combinatorial optimization (CO) on graphs has been attracting increasing interests from the machine learning community whereby data-driven approaches were recently devised to go beyond traditional manually-designated algorithms. In this paper, we study the robustness of a combinatorial solver as a blackbox regardless it is classic or learning-based though the latter can often be more interesting to the ML community. Specifically, we develop a practically feasible robustness metric for general CO solvers. A no-worse optimal cost guarantee is developed as such the optimal solutions are not required to achieve for solvers, and we tackle the non-differentiable challenge {in input instance disturbance} by resorting to black-box adversarial attack methods. Extensive experiments are conducted on 14 unique combinations of solvers and CO problems, and we demonstrate that the performance of state-of-the-art solvers like Gurobi can degenerate by over 20\% under the given time limit bound on the hard instances discovered by our robustness metric, raising concerns about the robustness of combinatorial optimization solvers. Source code and configuration details will be all made publicly available.
更多查看译文
关键词
Combinatorial Optimization,Robustness,Graph Neural Networks,Reinforcement Learning
AI 理解论文
溯源树
样例
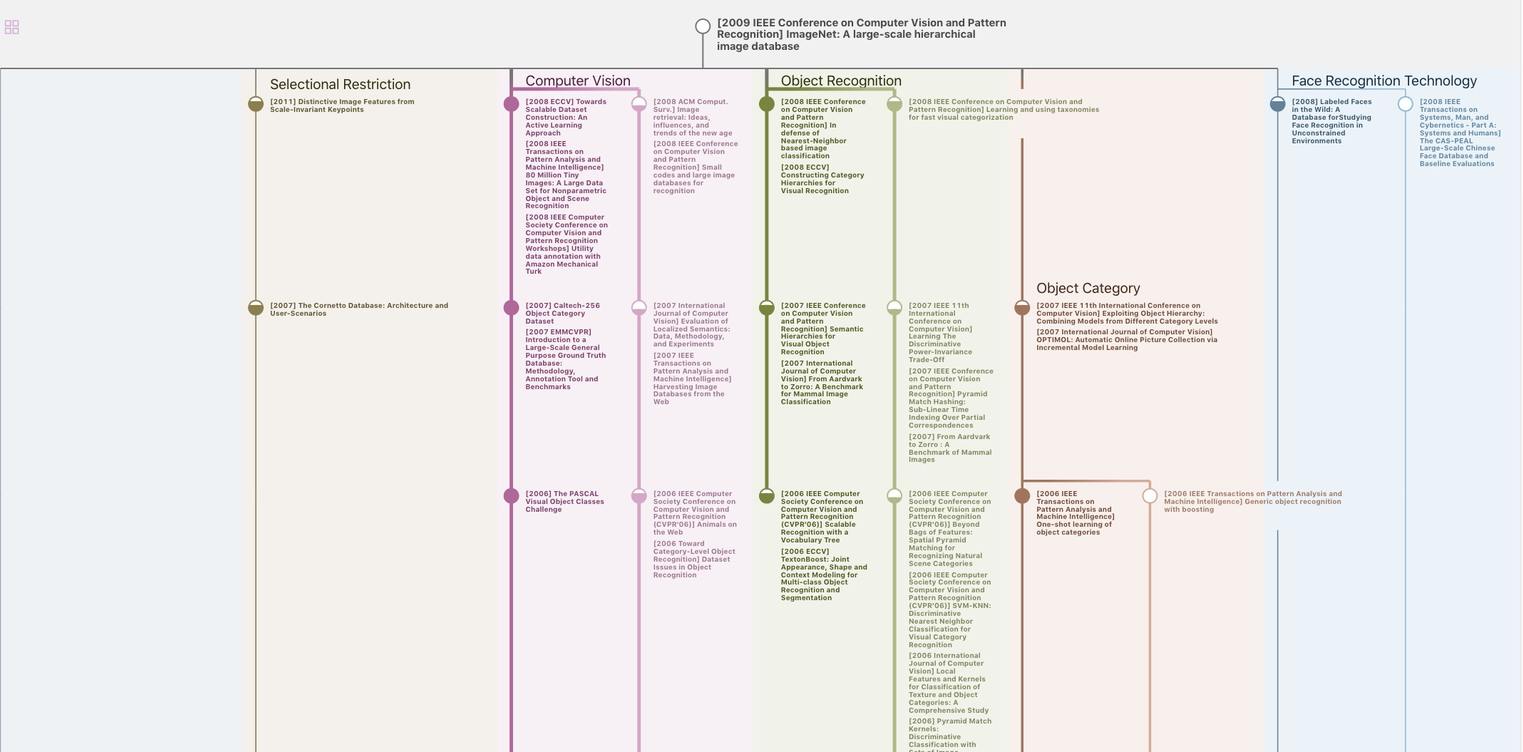
生成溯源树,研究论文发展脉络
Chat Paper
正在生成论文摘要