$k$NN Prompting: Learning Beyond the Context with Nearest Neighbor Inference
ICLR 2023(2023)
摘要
In-Context Learning, which formulates target tasks as prompt completion conditioned on in-context demonstrations, has become the prevailing and standard utilization of large language models.
In this paper, we disclose an actual predicament for this typical usage that it can not scale up with training data due to context length restrictions.
We then advocate a simple and effective solution, $k$NN Prompting, which not only outperforms In-Context Learning under few shot scenarios, but more importantly, can scale up with as many training data as are available.
$k$NN Prompting queries LLM with training data for distributed representations and caches them locally as anchors.
At inference time, it predicts by simply aggregating nearest neighbors.
We conduct comprehensive experiments and ablations across different scales of LLMs to demonstrate its substantial improvements, as well as other appealing aspects such as robustness and explainability.
The proposed approach successfully bridges data scaling into model scaling, and brings new potentials for the gradient-free paradigm of LLM deployment.
更多查看译文
关键词
Large Language Models,In-Context Learning,K Nearest Neighbors
AI 理解论文
溯源树
样例
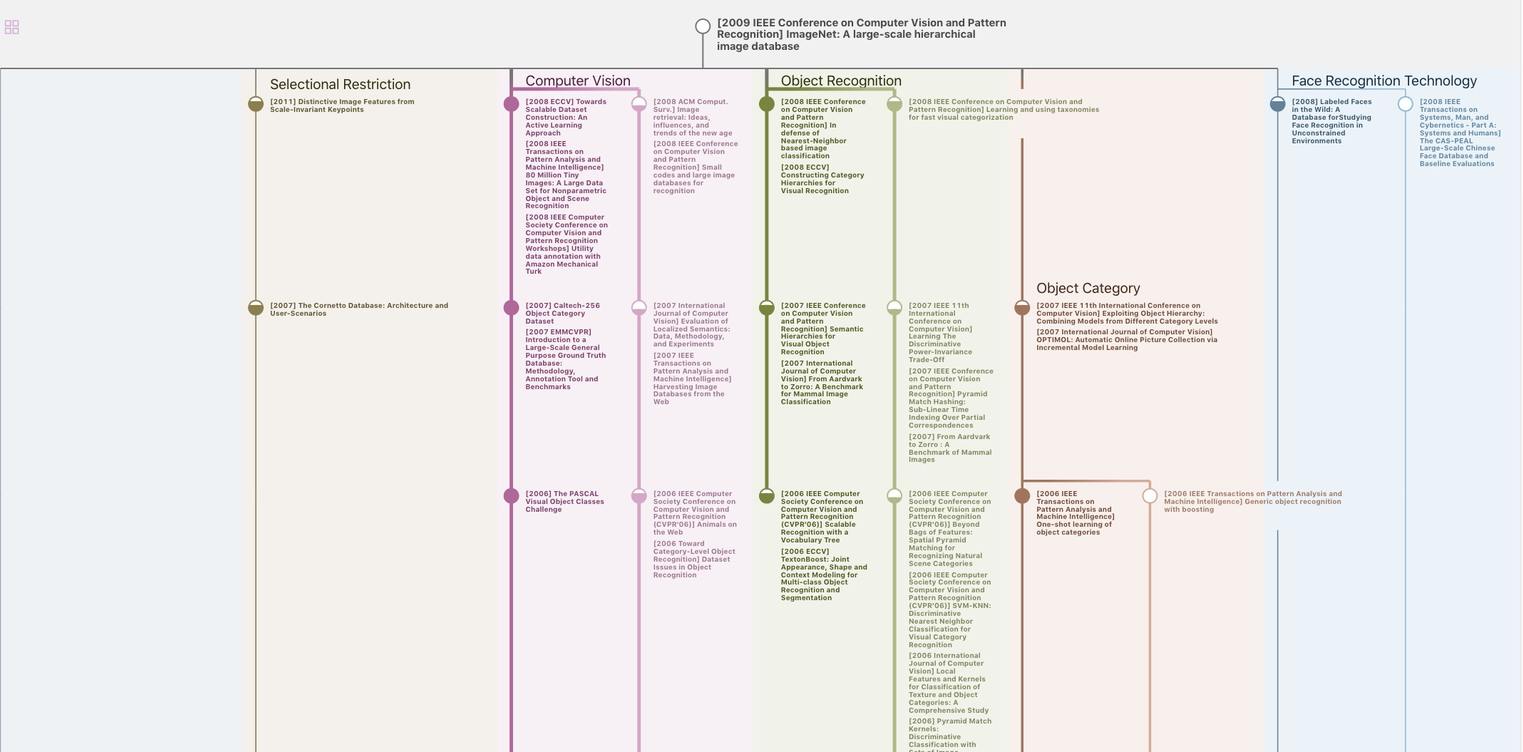
生成溯源树,研究论文发展脉络
Chat Paper
正在生成论文摘要