UniMax: Fairer and more Effective Language Sampling for Large-Scale Multilingual Pretraining
International Conference on Learning Representations(2023)
摘要
Pretrained multilingual large language models have typically used heuristic temperature-based sampling to balance between different languages. However previous work has not systematically evaluated the efficacy of different pretraining language distributions across model scales. In this paper, we propose a new sampling method, UniMax, that delivers more uniform coverage of head languages while mitigating overfitting on tail languages by explicitly capping the number of repeats over each language's corpus. We perform an extensive series of ablations testing a range of sampling strategies on a suite of multilingual benchmarks, while varying model scale. We find that UniMax outperforms standard temperature-based sampling, and the benefits persist as scale increases. As part of our contribution, we release: (i) an improved and refreshed mC4 multilingual corpus consisting of 29 trillion characters across 107 languages, and (ii) a suite of pretrained umT5 model checkpoints trained with UniMax sampling.
更多查看译文
AI 理解论文
溯源树
样例
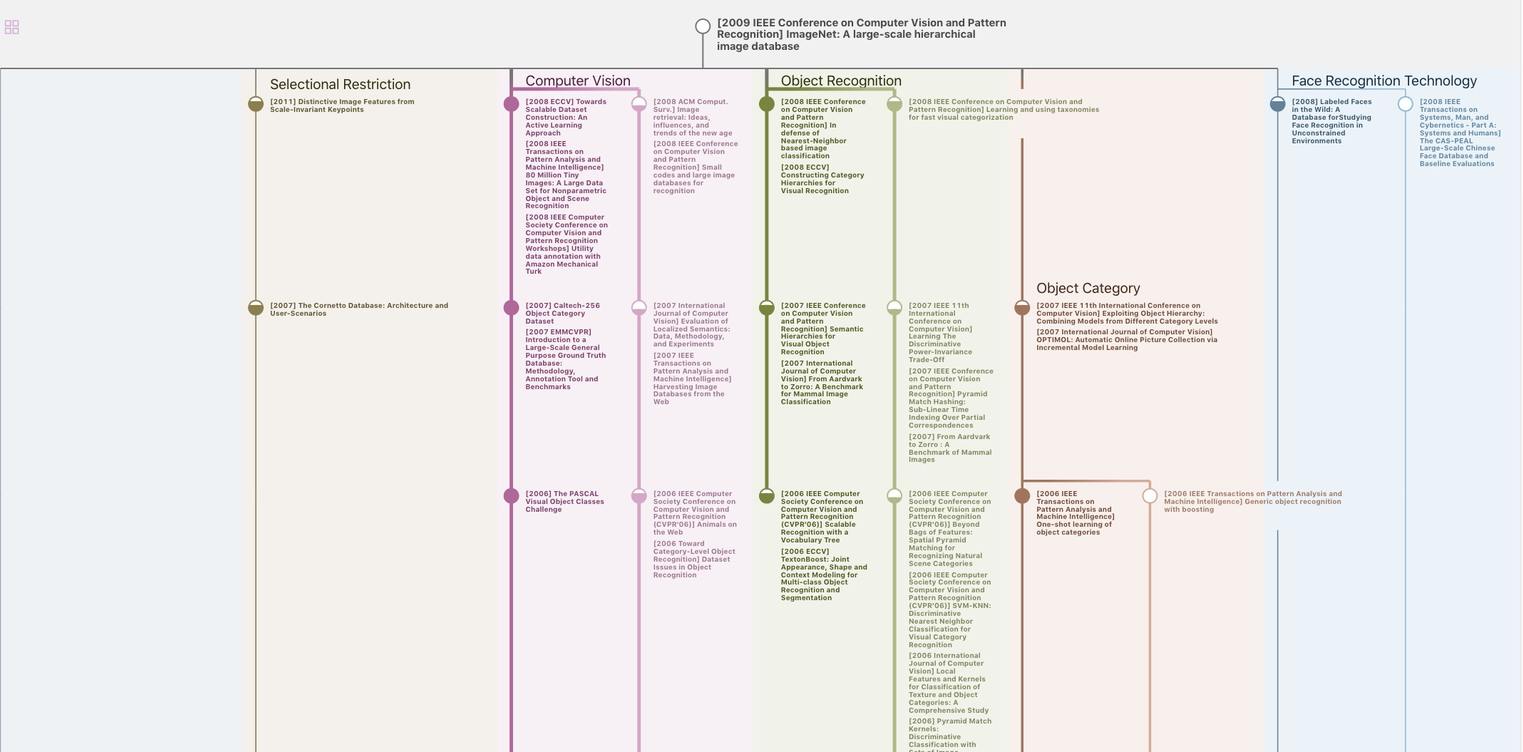
生成溯源树,研究论文发展脉络
Chat Paper
正在生成论文摘要