Learning multi-scale local conditional probability models of images
ICLR 2023(2023)
摘要
Deep neural networks can learn powerful prior probability models for images, as evidenced by high-quality synthesis results achieved with VAEs, GANs, and recent score-based diffusion methods. But these models are implicit, and the means by which these networks capture complex global statistical structure, apparently without suffering from the curse of dimensionality, remain a mystery. To study this, we generalize a multi-scale model class motivated by the renormalization group of theoretical physics. It circumvents the curse of dimensionality by assuming Markov structure of multi-scale wavelet coefficients conditioned on coarser scale coefficients. We parameterize the model using conditional convolutional neural networks with local receptive fields, which enforce stationary Markov properties. We test the capabilities of the model on a dataset of face images, which are highly non-stationary and contain long-range geometric structures. Remarkably, denoising, super-resolution, and image synthesis results demonstrate that these structures are well-captured, using significantly smaller neighborhoods than required by a CNN operating in the pixel domain.
更多查看译文
关键词
Image priors,Markov wavelet conditional models,multi-scale score-based image synthesis,denoising,super-resolution
AI 理解论文
溯源树
样例
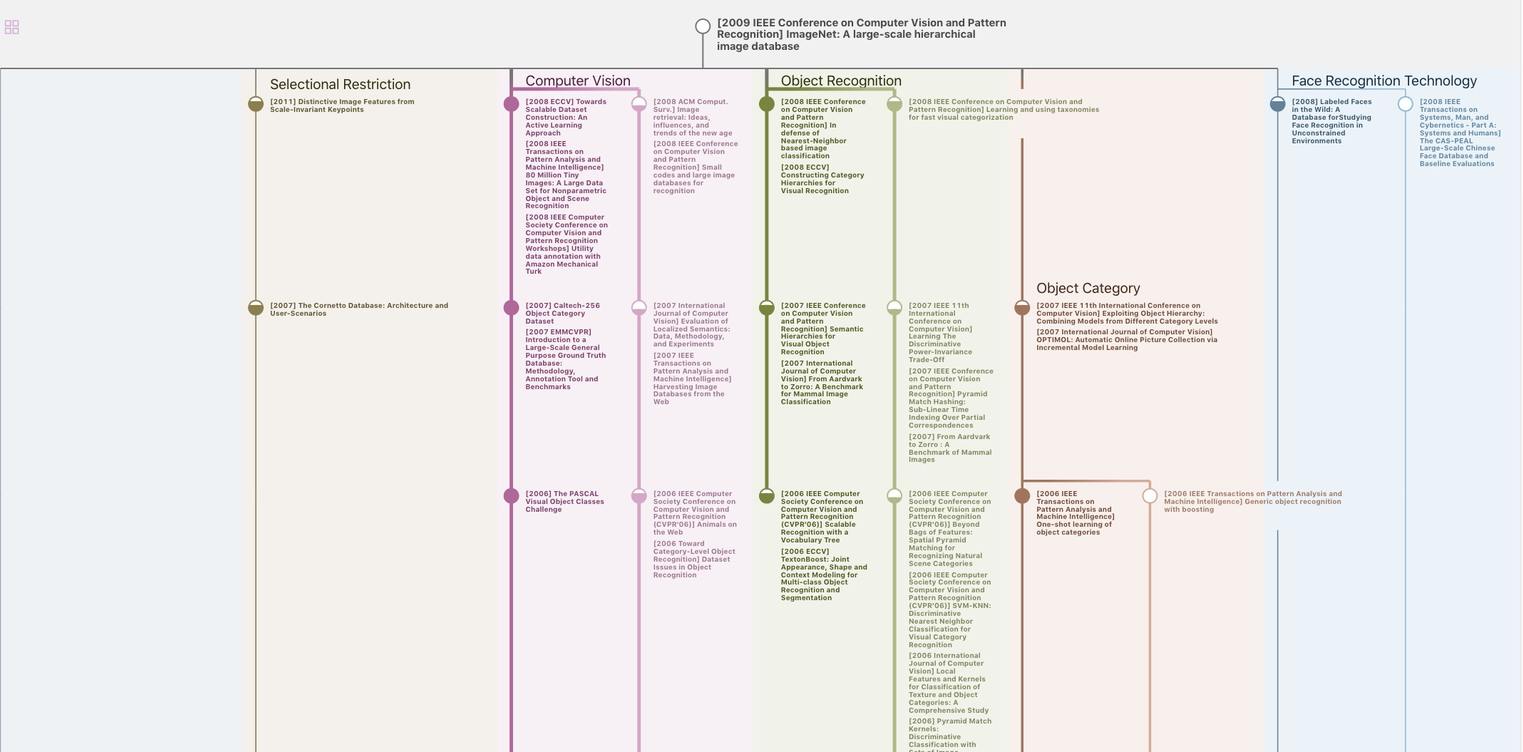
生成溯源树,研究论文发展脉络
Chat Paper
正在生成论文摘要