Rapid Identification and Classification of Eccentric Gravitational Wave Inspirals with Machine Learning
arxiv(2023)
摘要
Current templated searches for gravitational waves (GWs) emanated from compact binary coalescences (CBCs) assume that the binaries have circularized by the time they enter the sensitivity band of the LIGO-Virgo-KAGRA (LVK) network. However, certain formation channels predict that in future observing runs (O4 and beyond), a fraction of detectable binaries could enter the sensitivity band with a measurable eccentricity $e$. Constraining $e$ for each GW event with Bayesian parameter estimation methods is computationally expensive and time-consuming. This motivates the need for a machine learning based identification and classification scheme, which could weed out the majority of GW events as non-eccentric and drastically reduce the set of candidate eccentric GWs. As a proof of principle, we train a separable-convolutional neural network (SCNN) with spectrograms of synthetic GWs added to Gaussian noise characterized by O4 representative PSDs. We use the trained network to (i) segregate candidates as either eccentric or non-eccentric (henceforth called the detection problem) and (ii) classify the events as non-eccentric $(e = 0)$, moderately eccentric $(e \in (0, 0.2])$, and highly eccentric $(e \in (0.2, 0.5])$. On the detection problem, our best performing network detects eccentricity with $0.986$ accuracy and true and false positive rates of $0.984$ and $1.6\times 10^{-3}$, respectively. On the classification problem, the best performing network classifies signals with $0.962$ accuracy. We also find that our trained classifier displays close to ideal behavior for the data we consider.
更多查看译文
关键词
eccentric gravitational wave,machine learning,classification,rapid identification
AI 理解论文
溯源树
样例
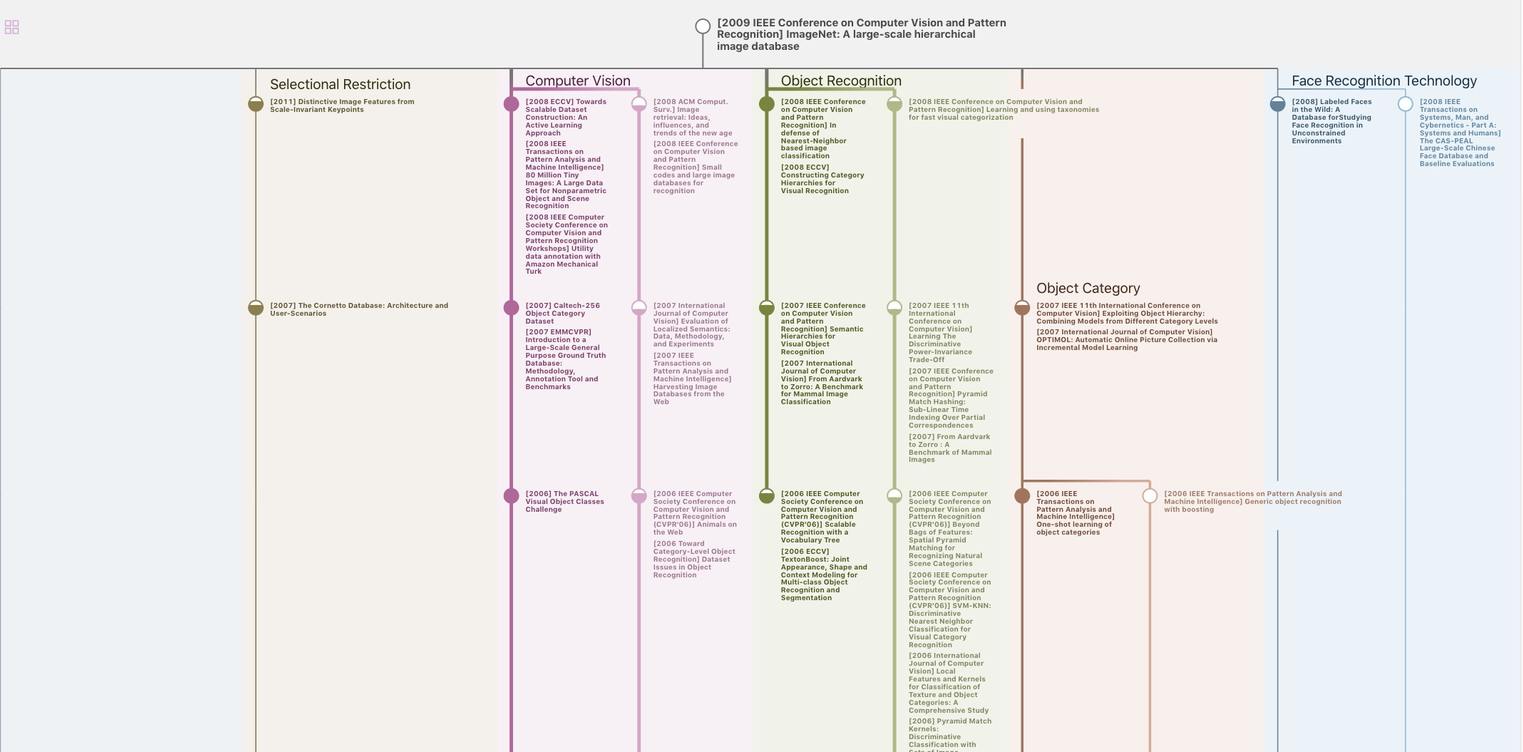
生成溯源树,研究论文发展脉络
Chat Paper
正在生成论文摘要