G-Rank: Unsupervised Continuous Learn-to-Rank for Edge Devices in a P2P Network
arxiv(2023)
摘要
Ranking algorithms in traditional search engines are powered by enormous training data sets that are meticulously engineered and curated by a centralized entity. Decentralized peer-to-peer (p2p) networks such as torrenting applications and Web3 protocols deliberately eschew centralized databases and computational architectures when designing services and features. As such, robust search-and-rank algorithms designed for such domains must be engineered specifically for decentralized networks, and must be lightweight enough to operate on consumer-grade personal devices such as a smartphone or laptop computer. We introduce G-Rank, an unsupervised ranking algorithm designed exclusively for decentralized networks. We demonstrate that accurate, relevant ranking results can be achieved in fully decentralized networks without any centralized data aggregation, feature engineering, or model training. Furthermore, we show that such results are obtainable with minimal data preprocessing and computational overhead, and can still return highly relevant results even when a user's device is disconnected from the network. G-Rank is highly modular in design, is not limited to categorical data, and can be implemented in a variety of domains with minimal modification. The results herein show that unsupervised ranking models designed for decentralized p2p networks are not only viable, but worthy of further research.
更多查看译文
关键词
edge devices,p2p
AI 理解论文
溯源树
样例
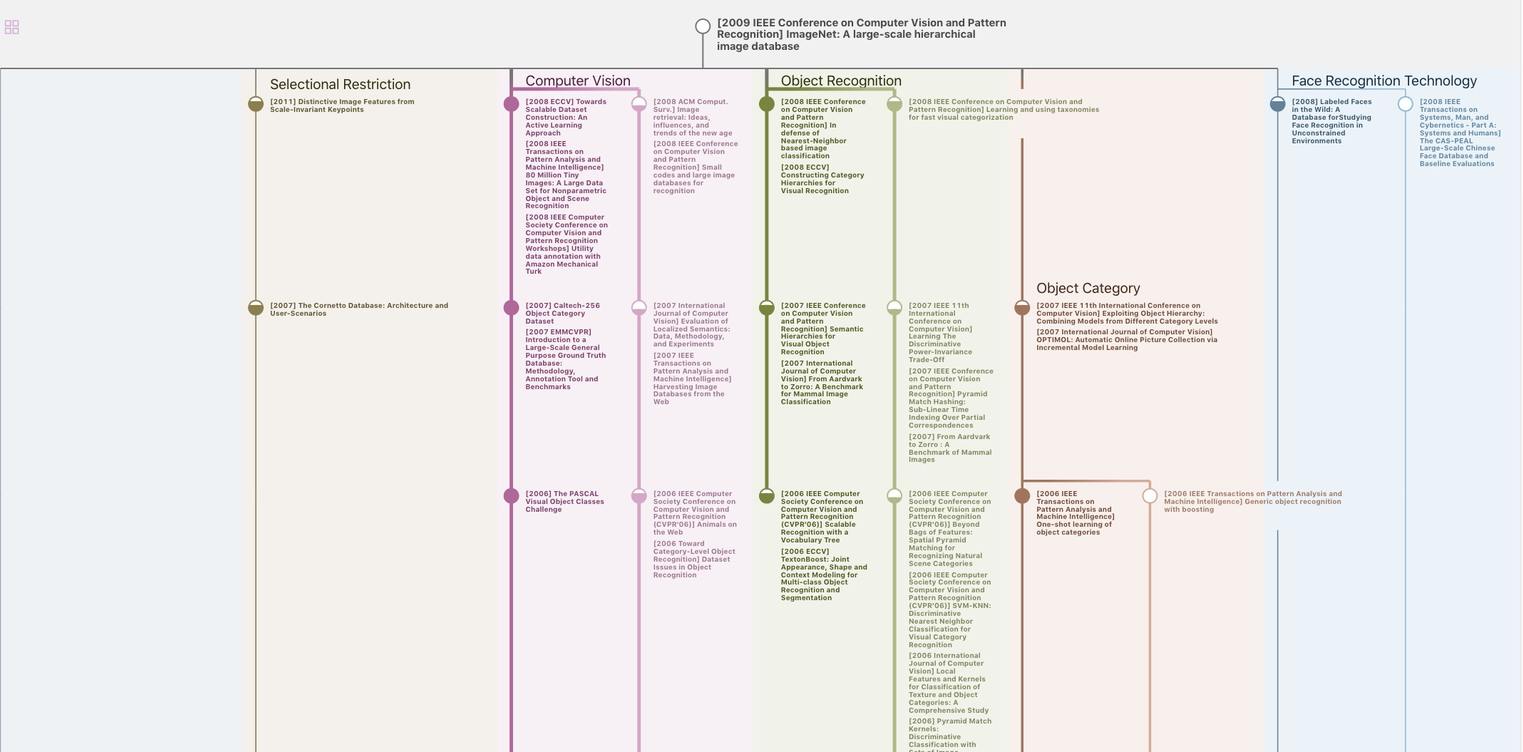
生成溯源树,研究论文发展脉络
Chat Paper
正在生成论文摘要