Adaptive Latent Transformation (ALT) for classification of resting state - fMRI
2022 IEEE Symposium Series on Computational Intelligence (SSCI)(2022)
摘要
Classification of resting state - functional Magnetic Resonance Imaging (rs-fMRI) data using deep learning algorithms is a challenging problem. Previous studies using latent space transformations to project the data into a discriminant space have shown promising results. However, their reliance on mathematical modelling of the latent space have limited their performance and generalization ability. Learnable latent space transformations have provided a new approach to solve such problems. In this paper, Adaptive Latent Transformation (ALT) method is proposed to address the shortcomings in literature. The ALT proposes an adaptable latent space transformation that can project the input data into a discriminant latent space of any pre-determined dimension using a learnable projection function. The ALT latent space transformation function is integrated into a convolutional neural network algorithm and its weights are updated using the backpropagation based learning strategy to minimize the error in the classification performance. Further, to prevent overfitting of the deep network classifier a novel Confidence based Sample Selection Strategy (CSSS) is proposed to selectively train the network with information-rich samples. The performance of the ALT classifier is evaluated using the ADHD200 rs-fMRI benchmark dataset over a 10 fold cross-validation study.
更多查看译文
关键词
Latent Space Transformation,Functional magnetic resonance imaging,ADHD200,Attention Deficit Hyperactivity Disorder
AI 理解论文
溯源树
样例
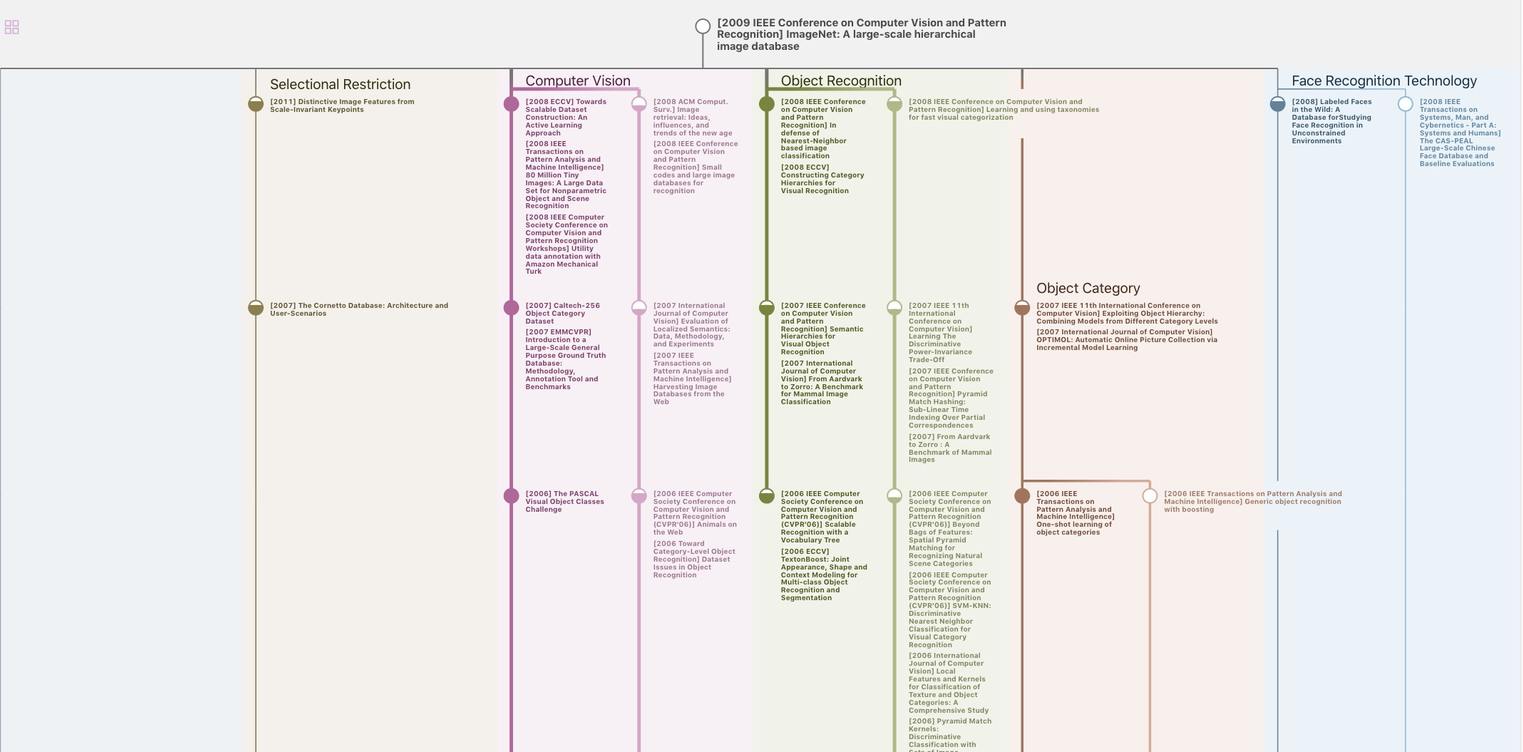
生成溯源树,研究论文发展脉络
Chat Paper
正在生成论文摘要