A Scheme for Effective Skin Disease Detection using Optimized Region Growing Segmentation and Autoencoder based Classification
Procedia Computer Science(2023)
摘要
Detecting skin disorders just through visual inspection is difficult due to the complicated and overlapping nature of sick lesions, background skin textures, skin hair, low illumination, etc. Computer Vision and Machine Learning are playing a great role in identifying the correct type of diseased lesion. However, in the presence of the aforementioned artefacts, current computational approaches are equally limited in their ability to detect complicated lesion structures. We provide a novel detection framework to enhance skin disease diagnosis. Beginning with segmentation and feature extraction from diseased lesions, this framework uses autoencoder based classification model. Diseased lesions are segmented using Optimized Region Growing using Grey Wolf Optimization (GWO). Texture features are extracted from the segmented lesion using Gray Level Co-occurrence Matrix (GLCM), and Weber Local Descriptor (WLD). Finally, a reduced feature set is generated through latent representation using the autoencoder. An integrated convolutional neural network is used to classify the diseased lesions from latent representation. The proposed framework significantly outperformed the traditional deep classification strategies, according to experimental findings.
更多查看译文
关键词
Grey Wolf Optimization,Gray Level Co-occurrence Matrix,Weber Local Descriptor
AI 理解论文
溯源树
样例
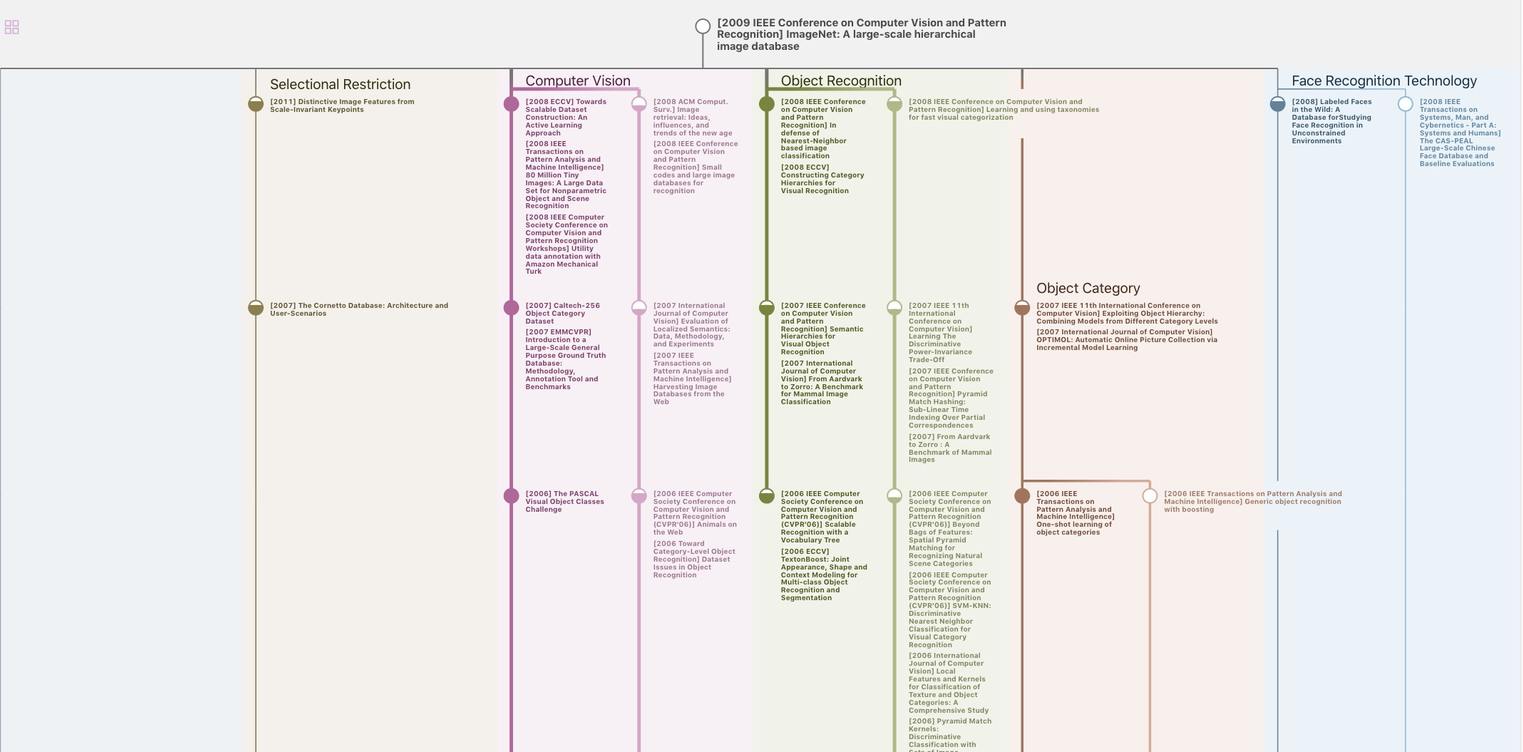
生成溯源树,研究论文发展脉络
Chat Paper
正在生成论文摘要