End-to-end pest detection on an improved deformable DETR with multihead criss cross attention.
Ecol. Informatics(2022)
摘要
In the ecological environment, pest infestation seriously affects the ecological balance; therefore, pest control is both necessary and urgent. However, most popular pest detection methods require various handcrafted com-ponents, which further limits the improvement of their pest detection performance. This study attempts to overcome this challenge from a new perspective by achieving an end-to-end network that eliminates the introduction of handcrafted components and effectively detects pests by combining residual networks and transformers. First, a squeeze-and-excitation module was introduced to assist the residual network fully extracting pest features. Second, a novel multihead criss cross attention module was designed to fully obtain the mutual relations between object queries with minimal computational cost. Third, data augmentation was incorporated to balance the sample categories. Finally, our model performance was evaluated based on a public pest dataset consisting of 25,378 images and 24 categories, and an average accuracy of 72.5% was achieved. The experimental results demonstrate that our method outperforms state-of-the-art methods. In addition to its excellent detection performance, this method demonstrates promising potential as an effective means of detecting other targets with similar characteristics. Therefore, it can be be effectively applied to practice and provide a new pest detection solution.
更多查看译文
关键词
Pest detection, Transformer, Attention mechanism, Deep learning, Criss cross attention
AI 理解论文
溯源树
样例
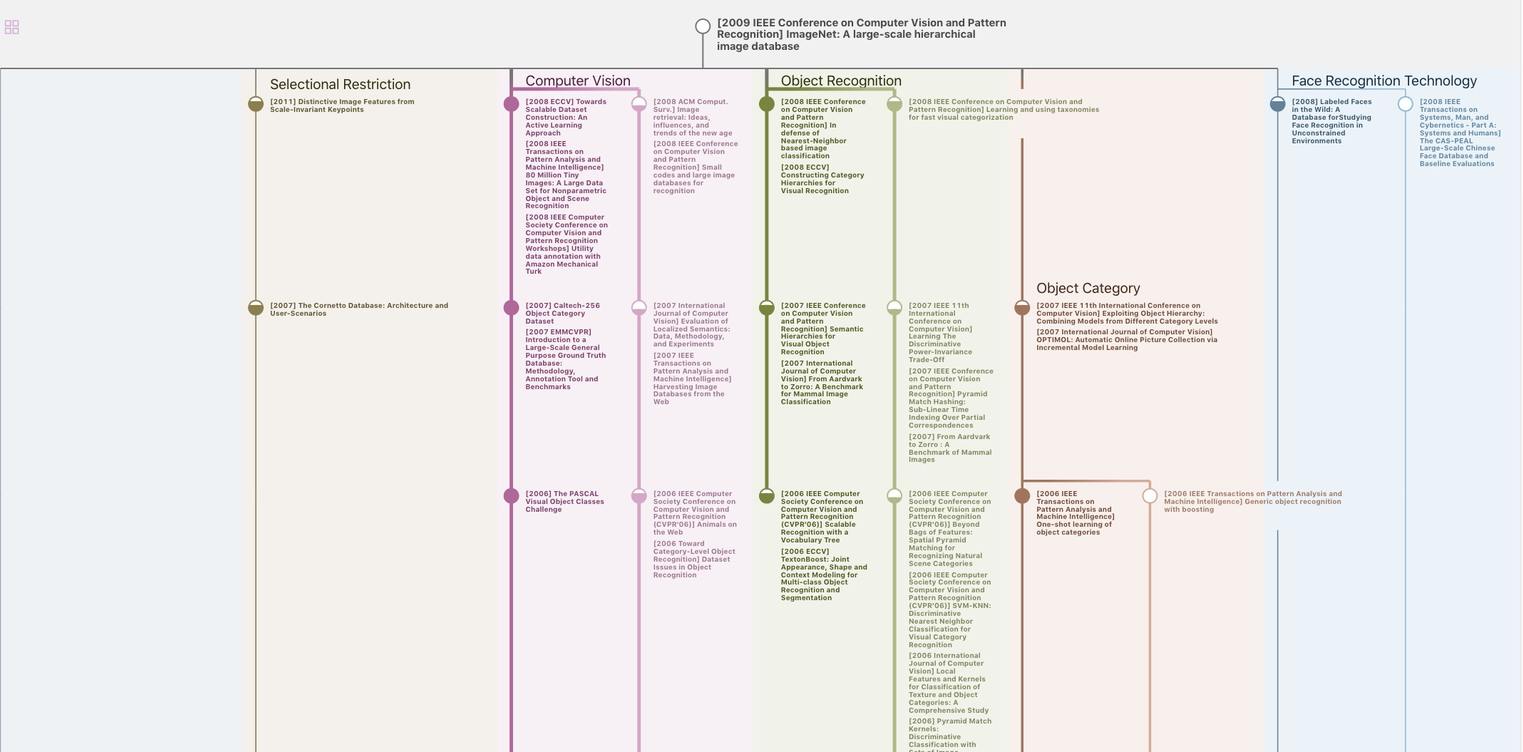
生成溯源树,研究论文发展脉络
Chat Paper
正在生成论文摘要