Speech Enhancement Using MLP-Based Architecture With Convolutional Token Mixing Module and Squeeze-and-Excitation Network.
IEEE Access(2022)
摘要
The Conformer has shown impressive performance for speech enhancement by exploiting the local and global contextual information, although it requires high computational complexity and many parameters. Recently, multi-layer perceptron (MLP)-based models such as MLP-mixer and gMLP have demonstrated comparable performances with much less computational complexity in the computer vision area. These models showed that all-MLP architectures may perform as good as more advanced structures, but the nature of the MLP limits the application of these architectures to the input with a variable length such as speech and audio. In this paper, we propose the cgMLP-SE model, which is a gMLP-based architecture with convolutional token mixing modules and squeeze-and-excitation network to utilize both local and global contextual information as in the Conformer. Specifically, the token-mixing modules in gMLP are replaced by convolutional layers, squeeze-and-excitation network-based gating is applied on top of the convolutional gating module, and additional feed-forward layers are added to make the cgMLP-SE module a macaron-like structure sandwiched by feed-forward layers like a Conformer block. Experimental results on the TIMIT-DNS noise dataset and the Voice Bank-DEMAND dataset showed that the proposed method exhibited similar speech quality and intelligibility to the Conformer with a smaller model size and less computational complexity.
更多查看译文
关键词
Speech enhancement,local and global information,low computational complexity
AI 理解论文
溯源树
样例
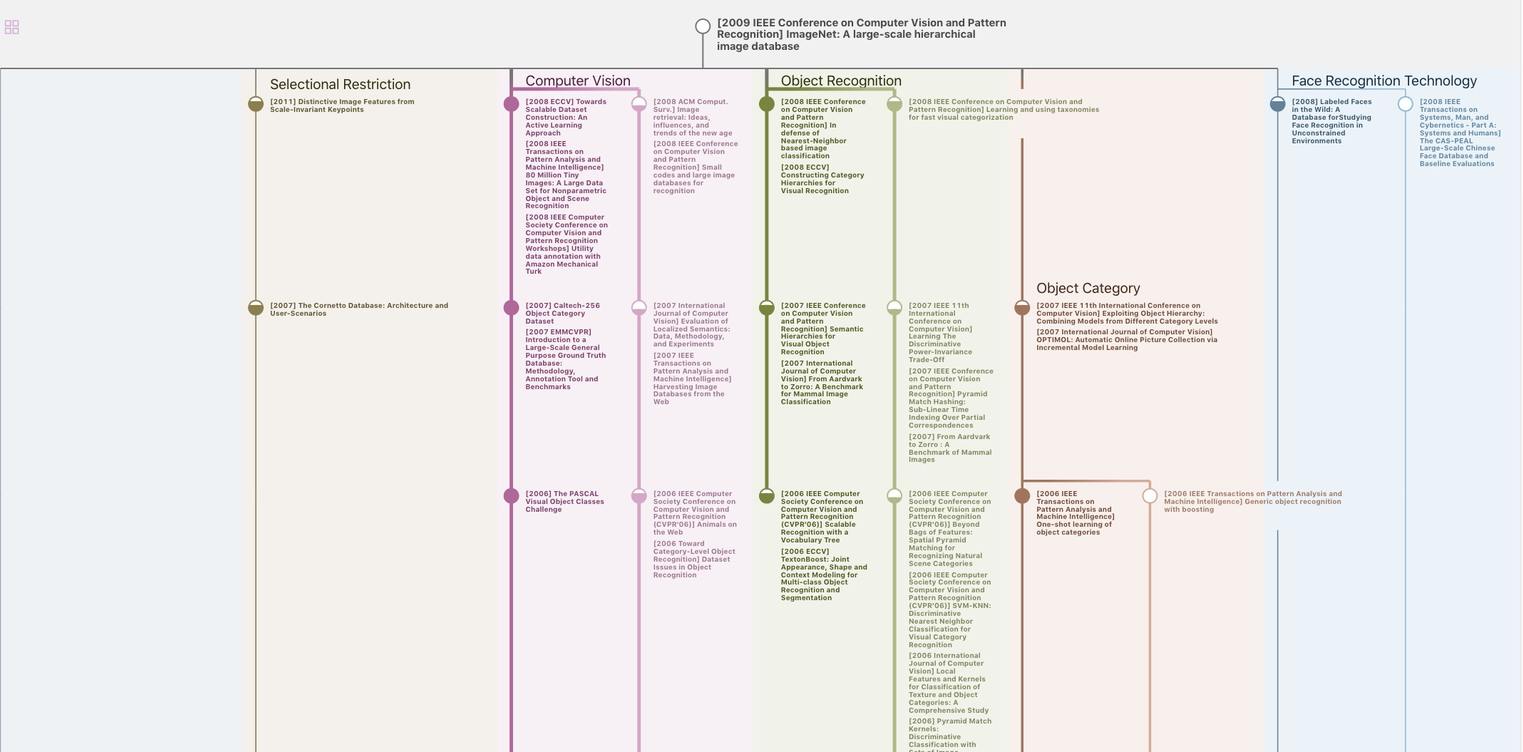
生成溯源树,研究论文发展脉络
Chat Paper
正在生成论文摘要