Completing Scientific Facts in Knowledge Graphs of Research Concepts.
IEEE Access(2022)
摘要
In the last few years, we have witnessed the emergence of several knowledge graphs that explicitly describe research knowledge with the aim of enabling intelligent systems for supporting and accelerating the scientific process. These resources typically characterize a set of entities in this space (e.g., tasks, methods, evaluation techniques, proteins, chemicals), their relations, and the relevant actors (e.g., researchers, organizations) and documents (e.g., articles, books). However, they are usually very partial representations of the actual research knowledge and may miss several relevant facts. In this paper, we introduce SciCheck, a new triple classification approach for completing scientific statements in knowledge graphs. SciCheck was evaluated against other state-of-the-art approaches on seven benchmarks, yielding excellent results. Finally, we provide a real-world use case and applied SciCheck to the Artificial Intelligence Knowledge Graph (AI-KG), a large-scale automatically-generated open knowledge graph including 1.2M statements extracted from the 333K most cited articles in the field of Artificial Intelligence, and generated a new version of this knowledge graph with 300K additional triples.
更多查看译文
关键词
Machine learning,Feature extraction,Semantic Web,Task analysis,Context modeling,Computational modeling,Benchmark testing,Knowledge based systems,Knowledge graphs,science of science,knowledge graph completion,triple classification,machine learning,semantic web
AI 理解论文
溯源树
样例
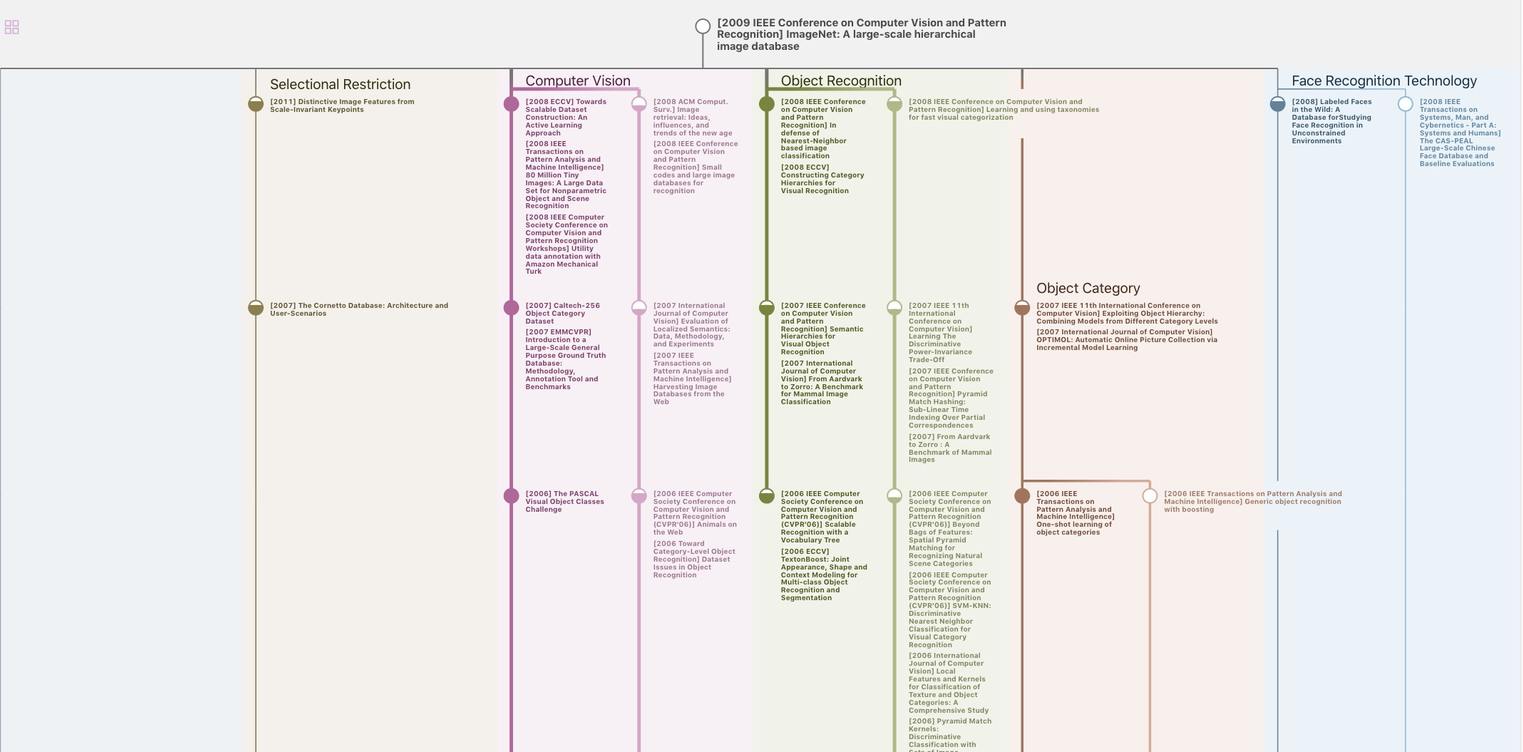
生成溯源树,研究论文发展脉络
Chat Paper
正在生成论文摘要