Multitask Neural Tensor Factorization for Road Traffic Speed-Volume Correlation Pattern Learning and Joint Imputation.
IEEE Trans. Intell. Transp. Syst.(2022)
摘要
Missing data is a common and critical problem in the stage of traffic data collection and processing. How to impute the missing values in spatio-temporal traffic data has been a challenging topic for a long time. Recently, a variety of methods have been proposed to impute the missing values. Among them, the tensor-based methods show higher competence in multi-dimensional traffic data imputation. However, the previous studies of tensor factorization rarely considered the joint imputation of multiple correlative data such as traffic speed and traffic volume. In this paper, a novel method called Multi-Task Neural Tensor Factorization (MTNTF) is proposed to learn the non-linear correlation patterns of traffic speed-volume, and then address the joint imputation of traffic speed and traffic volume. Extensive experiments on a real dataset show our MTNTF significantly outperforms the state-of-the-art methods in element-wise missing and fiber-wise missing cases. In addition, our method can impute the slice-wise missing values of traffic volume based on incomplete traffic speed.
更多查看译文
关键词
Multi-task learning,neural tensor factorization,traffic data joint imputation,traffic speed-volume correlation pattern,automatic vehicle identification
AI 理解论文
溯源树
样例
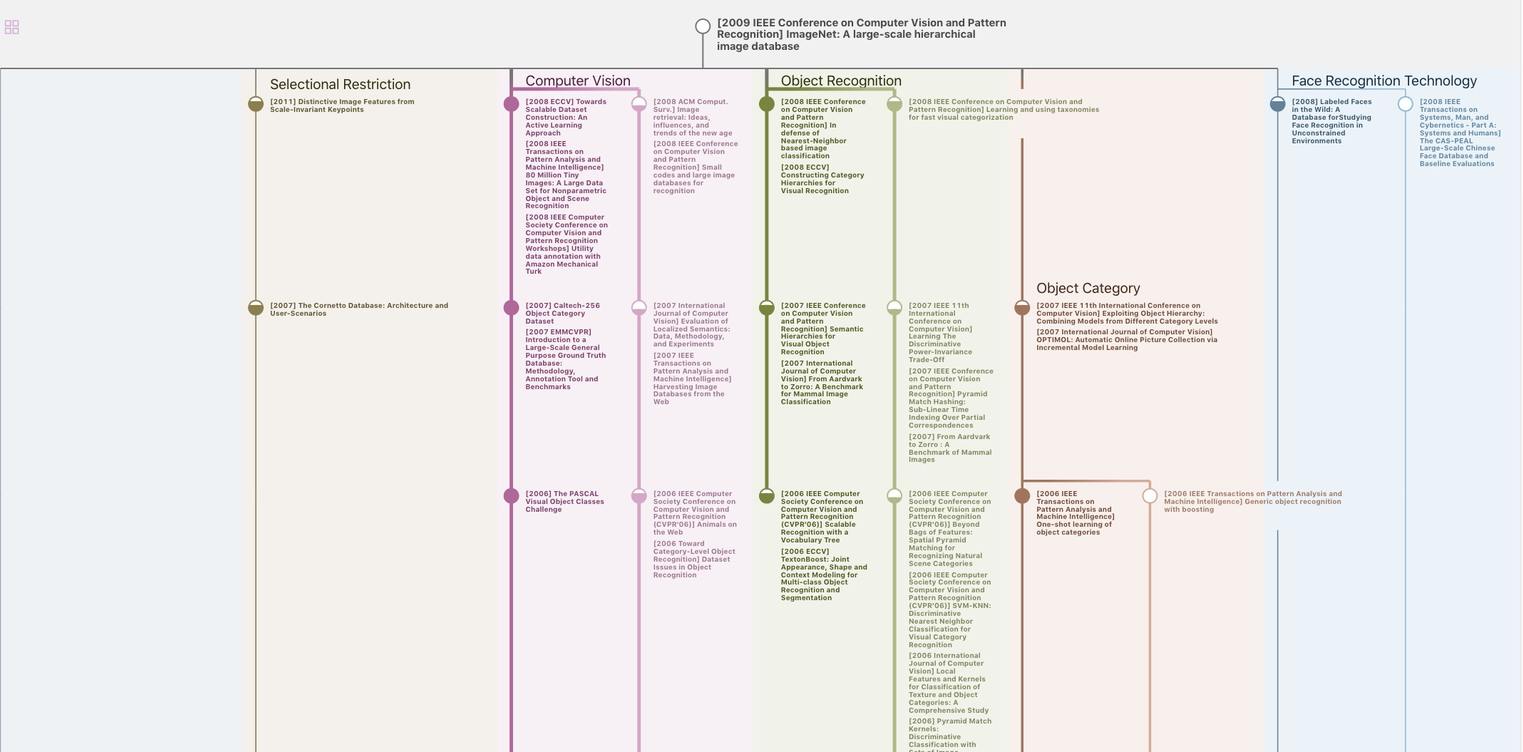
生成溯源树,研究论文发展脉络
Chat Paper
正在生成论文摘要