Lightweight Tensorized Neural Networks for Hyperspectral Image Classification.
IEEE Trans. Geosci. Remote. Sens.(2022)
摘要
Deep learning methods have demonstrated excellent performance in hyperspectral image (HSI) classification. However, these methods mainly focus on improving the classification accuracy while ignoring their high complexity. By considering that the data formats of both HSIs and network weights can be represented in the form of tensors, we develop a new lightweight tensorized neural network (TNN) for HSI classification that takes advantage of low-rank tensor decomposition techniques to reduce complexity. First, inspired by tensor train (TT)-based tensorized convolutional layers, a new tensorized 2D convolutional layer based on chain calculation (with better expression ability) is introduced. Based on this innovation, a new lightweight 2D-TNN is designed for HSI classification. Furthermore, to better preserve the intrinsic structure of HSI data, a new lightweight 3D-TNN is proposed by extending the tensorized 2D convolutional layers to their 3D versions. Quantitative and comparative experiments on three widely used datasets show that the proposed models are able to achieve the state-of-the-art performance (with a low number of model parameters) for different training sample sizes, especially for very small training sets.
更多查看译文
关键词
Classification,convolutional neural networks (CNNs),hyperspectral images (HSIs),network compression,tensor decomposition
AI 理解论文
溯源树
样例
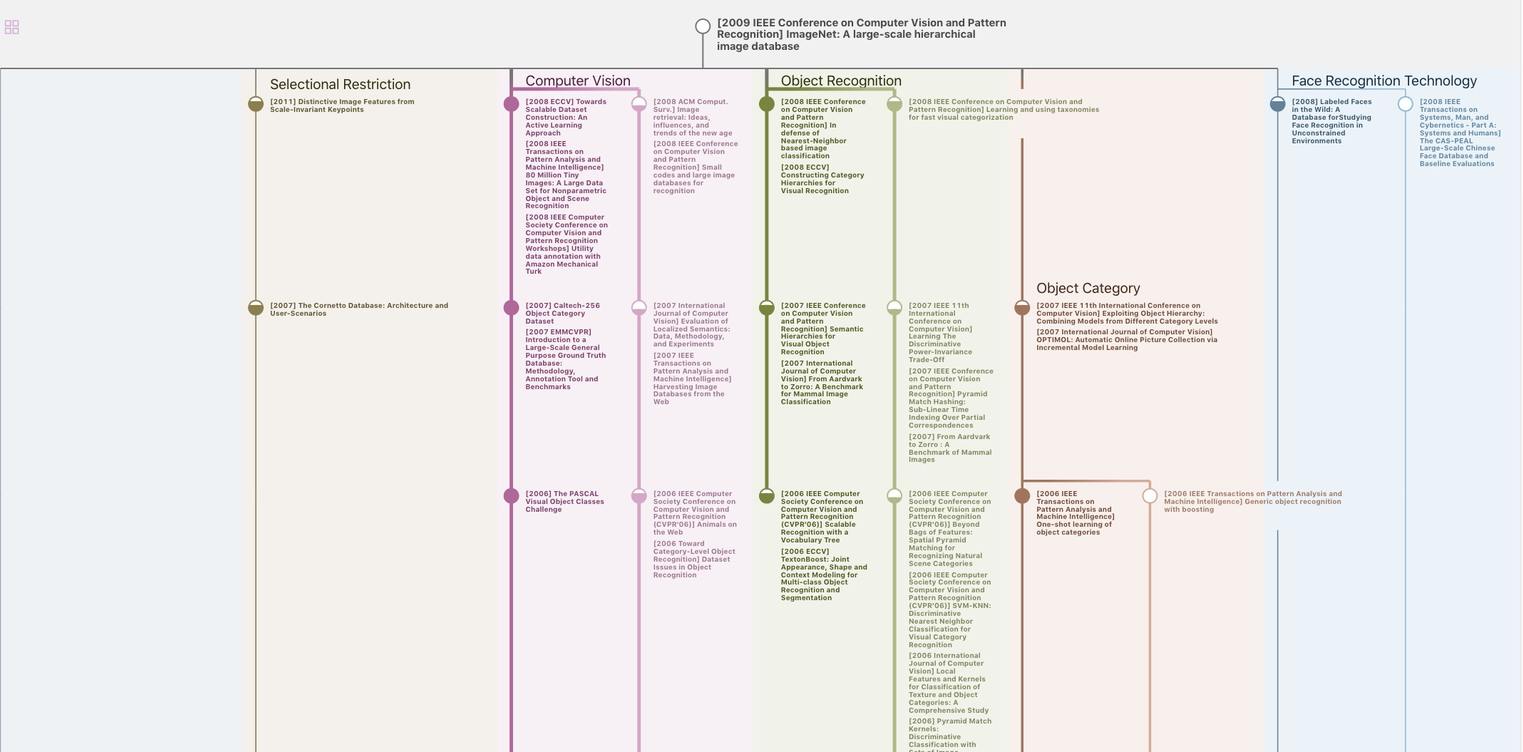
生成溯源树,研究论文发展脉络
Chat Paper
正在生成论文摘要