MIGT: Multi-modal image inpainting guided with text
Neurocomputing(2023)
摘要
In this work, we propose MIGT, a novel framework for multi-modal image inpainting that introduces textual description as guidance. We divide MIGT into three components: Coarse-to-Fine Image Inpainting Module (CFIM), Visual-Textual modalities Fusion Module (VTFM), and Multi-modal Semantic Alignment Module (MSAM). CFIM is a Unet-based inpainting model in a coarse-to-fine manner. The coarse inpainting stage produces images of rough shape and color according to the source corrupted images and the corresponding textual descriptions. The fine inpainting stage generates the final high-quality images with fine-grained textures. VTFM aims to reasonably fuse visual-textual modalities. First, we feed visual and textual features into the proposed visual-aware textual filtering mechanism to adaptively focus on desired words related to the missing areas. Then the filtered visual-aware textual features pass through an Attentional Generative Network (AGN) to obtain fusion features that are fed into the middle layers of the coarse stage for subsequent image inpainting. MSAM takes the generated image as input, reconstructing a textual description that semantically aligns the given one to guarantee the semantic consistency between the generated image and the input textual description. Extension experiments conducted on Oxford-102 flower and CUB-200–2011 bird datasets demonstrate the effectiveness of our proposed method.
更多查看译文
关键词
Multimodal learning,Generative Adversarial Network,Text-to-image,Image inpainting
AI 理解论文
溯源树
样例
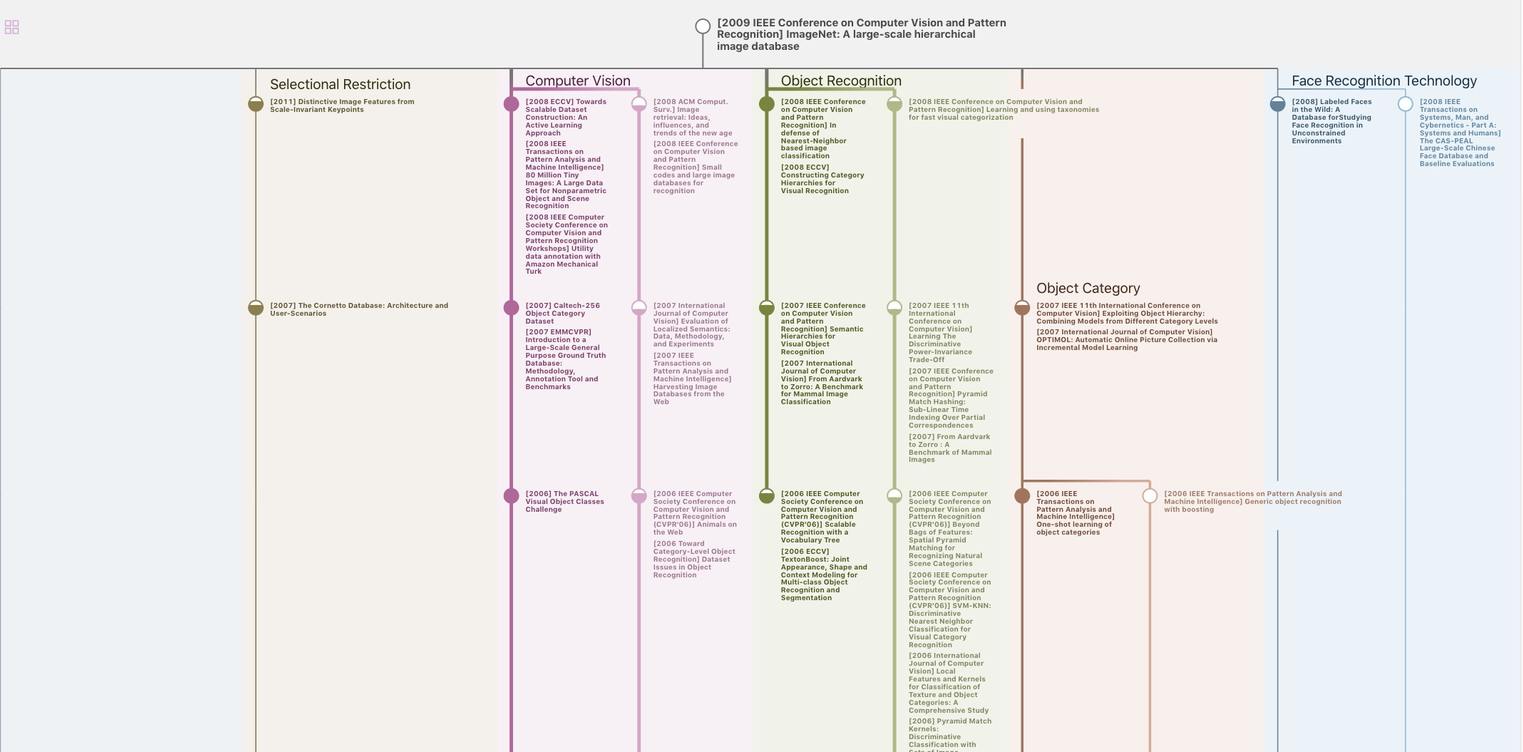
生成溯源树,研究论文发展脉络
Chat Paper
正在生成论文摘要