Depth Completion via A Dual-Fusion Method.
ICPR(2022)
摘要
Depth completion technology based on multi-level feature fusion (MF) strategy has recently achieved remarkable success. However, the existing MF-based methods treat RGB features and depth map features equally when performing modal fusion but ignore the difference in semantic richness and sparsity between them, which leads to the results generated by these methods overfitting the shape of RGB and harm to the accuracy of depth value. To address this problem, we proposed a novel dual fusion (DF) strategy for MF-based depth completion, which can prevent overfitting by weakening the influence of RGB features on the generated results through two fusion stages. The entire DF framework consists of two multi-level fusion modules. The first fusion module performs a simple fusion of RGB features and depth features, while the second fusion module enriches the sparse image representation with the previously obtained fused features. Besides, we utilize non-local sparse attention to solve the problem that ordinary convolution is not capable of expressing depth map features enough. We test our approach on the outdoor KITTI test set and achieve the state-of-the-art (SOTA) performance in RMSE. Extensive experiments on the indoor NYUv2 dataset and KITTI validation set further demonstrate that our approach outperforms existing MF-based methods.
更多查看译文
关键词
depth completion technology,depth features,depth map features,dual-fusion method,fused features,fusion stages,indoor NYUv2 dataset,KITTI validation set,MF-based depth completion,MF-based methods,multilevel feature fusion strategy,multilevel fusion modules,novel dual fusion strategy,outdoor KITTI test set,RGB features,semantic richness
AI 理解论文
溯源树
样例
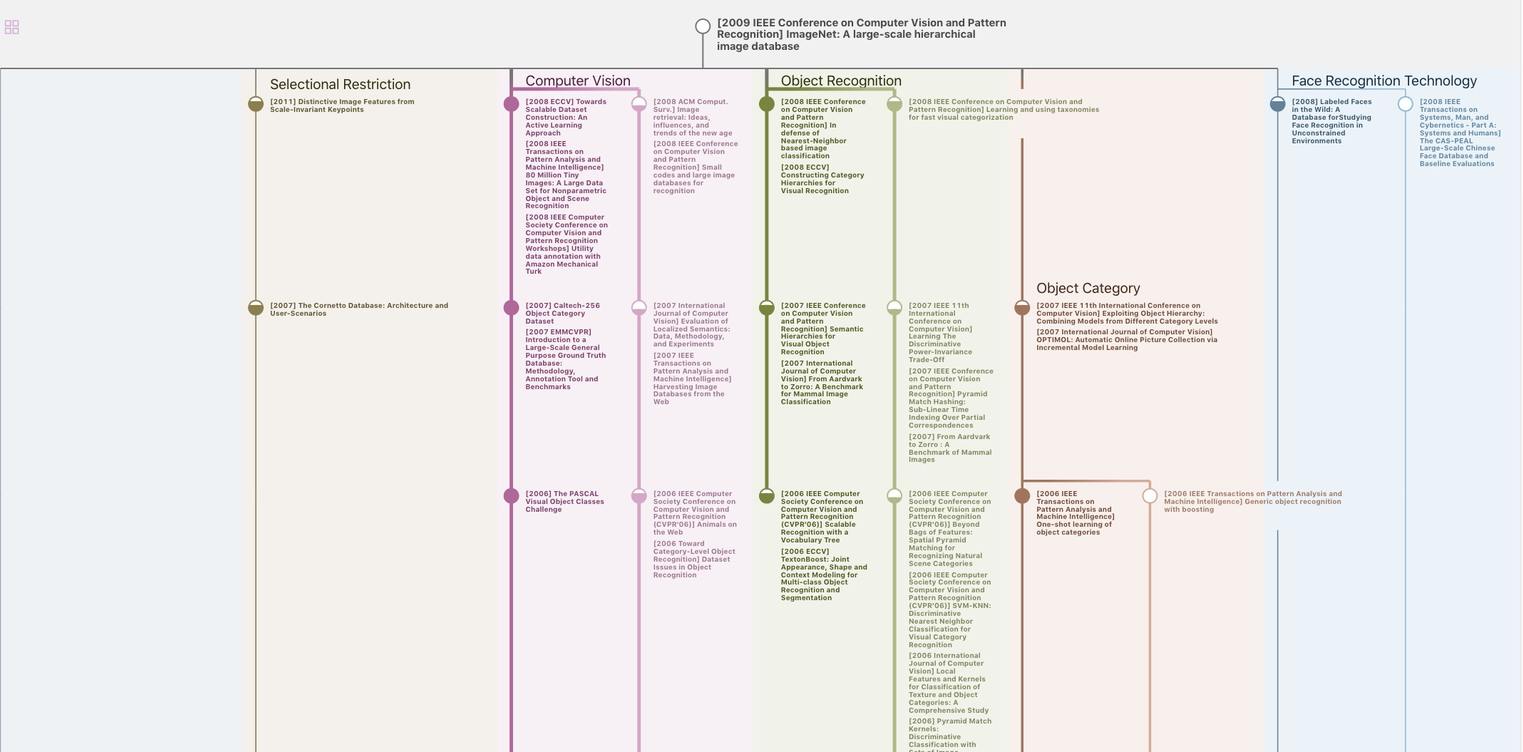
生成溯源树,研究论文发展脉络
Chat Paper
正在生成论文摘要