Semi-Supervised Fine-Grained Classification with Web Data via Noisy Sample Selection.
ICPR(2022)
摘要
For fine-grained classification, it is extremely difficult and costly to acquire the annotated data. Hence, some studies propose to use web data for fine-grained classification. However, the web data contains tremendous noisy labels, which can affect the classification results. Although many previous studies propose to discard noisy data via sample selection, they also discard some valid data. The valid data denotes hard or mislabeled samples that can enhance the robustness of the model. To solve the above problems, we propose a novel method to discard irrelevant noisy data from web data while keeping valid data for fine-grained classification. Specifically, we divide the web data into clean and noisy samples and then distinguish the noisy samples into openset and close-set noises. Finally, the model is constructed in a semi-supervised manner, where the clean samples are used as the labeled set, and the close-set noises are used as the unlabeled set. Extensive experiments verify that our method can improve the classification performance by an average of 1.89% on three fine-grained benchmark datasets compared with the current methods. The experimental results prove the effectiveness of the combination of sample selection and semi-supervised training strategy.
更多查看译文
关键词
annotated data,fine-grained benchmark datasets,fine-grained classification,irrelevant noisy data,noisy sample selection,noisy samples,tremendous noisy labels,valid data denotes,web data
AI 理解论文
溯源树
样例
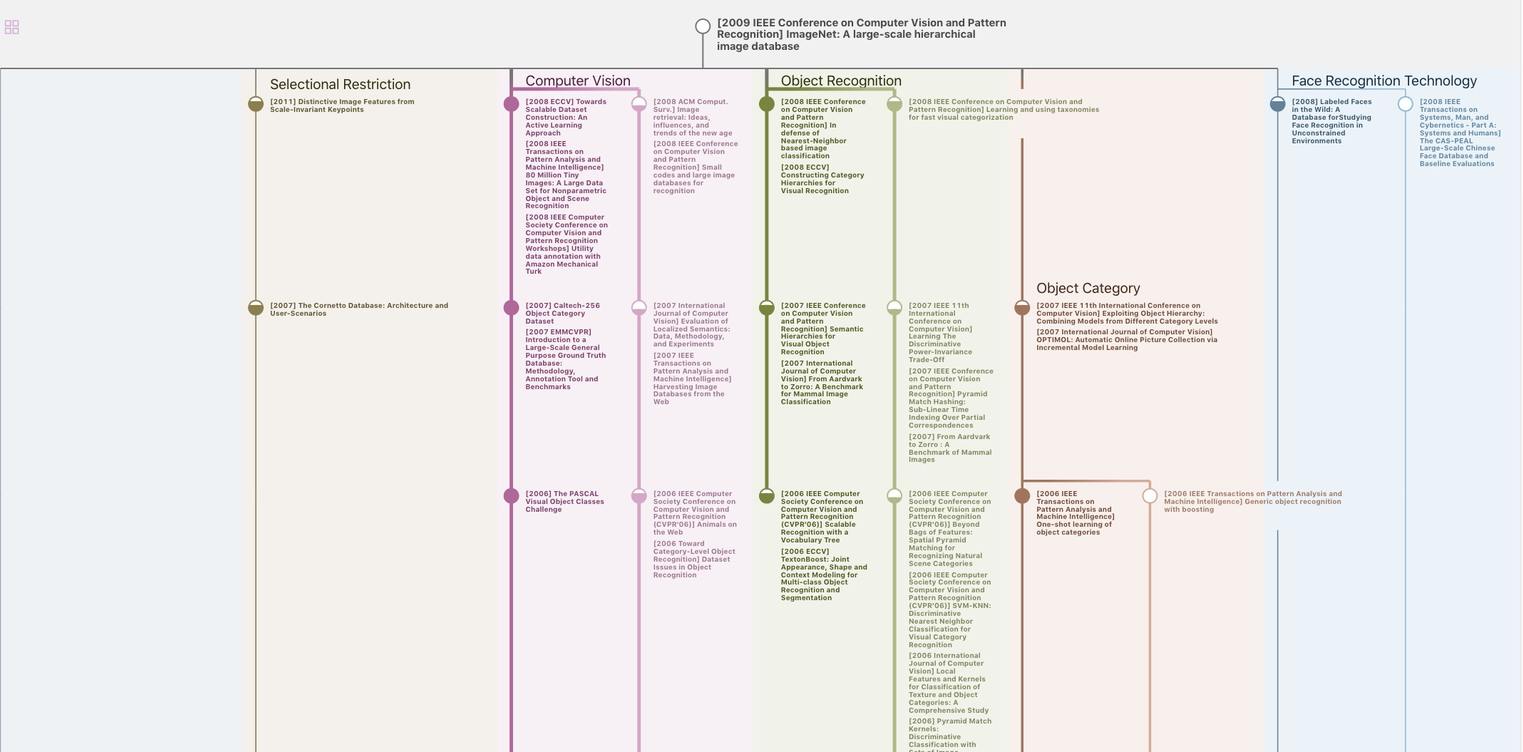
生成溯源树,研究论文发展脉络
Chat Paper
正在生成论文摘要