ISD-QA: Iterative Distillation of Commonsense Knowledge from General Language Models for Unsupervised Question Answering.
ICPR(2022)
摘要
Commonsense question answering has primarily been tackled through supervised transfer learning, where a language model pre-trained on large amounts of data is used as the starting point. While successful, the approach requires large amounts of labeled question-answer pairs, with increasingly larger amounts of data required as the complexity of scenarios or tasks such as commonsense QA increases. In this paper, we hypothesize that large-scale pre-training of language models encodes the necessary commonsense knowledge to answer common questions in context without labeled data. We propose a novel framework called Iterative Self Distillation for QA (ISD-QA), which extracts the "dark knowledge" encoded during largescale pre-training of language models to provide supervision for commonsense question answering. We show that the approach can be used to train common neural QA models for commonsense question answering by distilling knowledge from language models in an unsupervised manner. With no bells and whistles, we achieve an average of 68% of the performance of fully supervised QA models while requiring no labeled training data. Extensive experiments on three public benchmarks (OpenBookQA, HellaSWAG, and CommonsenseQA) show the effectiveness of the proposed approach.
更多查看译文
关键词
commonsense knowledge,general language models
AI 理解论文
溯源树
样例
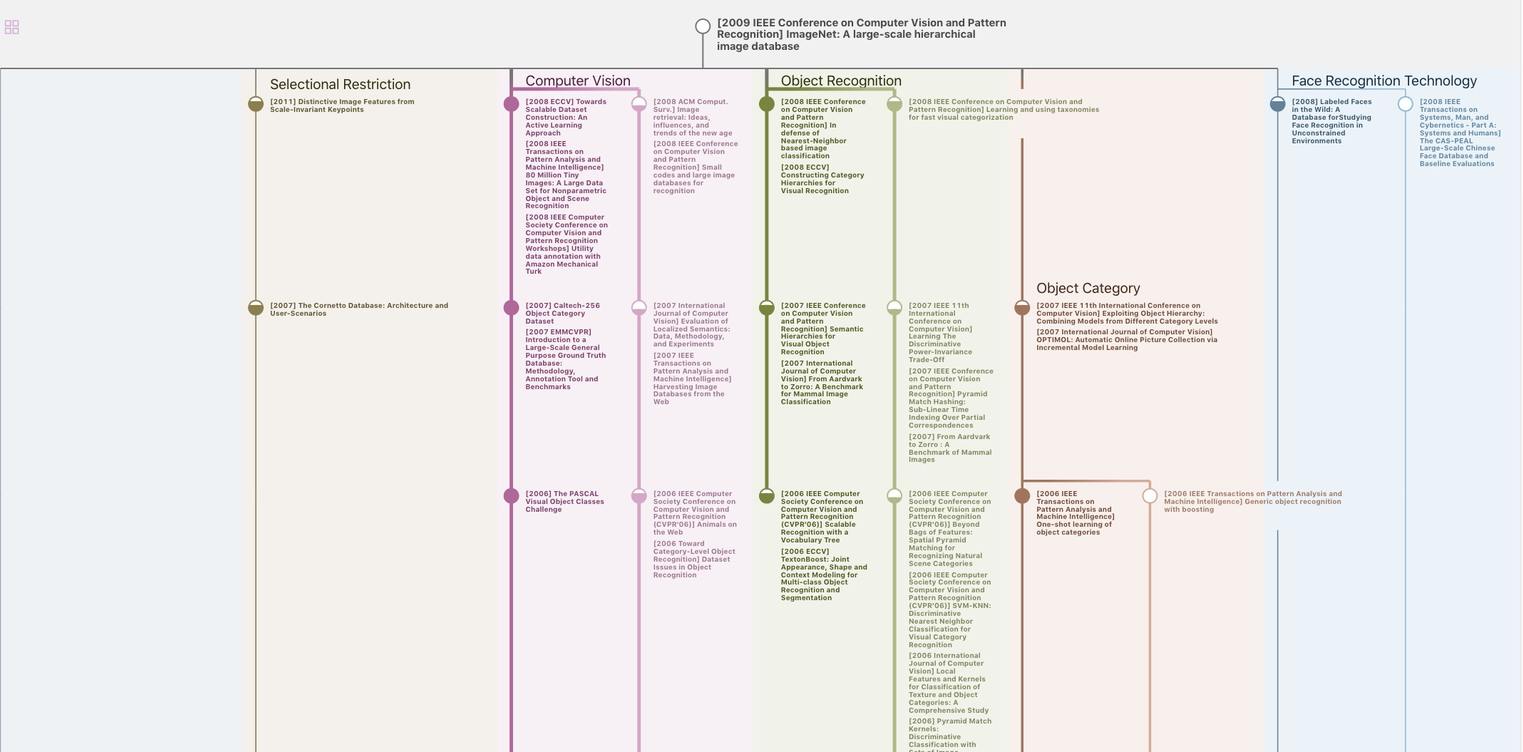
生成溯源树,研究论文发展脉络
Chat Paper
正在生成论文摘要