A co-training approach for spatial data disaggregation
SIGSPATIAL/GIS(2022)
摘要
Socio-demographic information is usually only accessible at relatively coarse spatial resolutions. However, its availability at thinner granularities is of substantial interest for several stakeholders, since it enhances the formulation of informed hypotheses on the distribution of population indicators. Spatial disaggregation methods aim to compute these fine-grained estimates, often using regression algorithms that employ ancillary data to re-distribute the aggregated information. However, since disaggregation tasks are ill-posed, and given that examples of disaggregated data at the target geospatial resolution are seldom available, model training is particularly challenging. We propose to address this problem through a self-supervision framework that iteratively refines initial estimates from seminal disaggregation heuristics. Specifically, we propose to co-train two different models, using the results from one model to train/refine the other. By doing so, we are able to explore complementary views from the data. We assessed the use of co-training with a fast regressor based on random forests that takes individual raster cells as input, together with a more expressive model, based on a fully-convolutional neural network, that takes raster patches as input. We also compared co-training against the use of self-training with a single model. In experiments involving the disaggregation of a socio-demographic variable collected for Continental Portugal, the results show that our co-training approach outperforms alternative disaggregation approaches, including methods based on self-training or co-training with two similar fully-convolutional models. Co-training is effective at exploring the characteristics of both regression algorithms, leading to a consistent improvement in different types of error metrics.
更多查看译文
关键词
spatial data disaggregation,spatial data,co-training
AI 理解论文
溯源树
样例
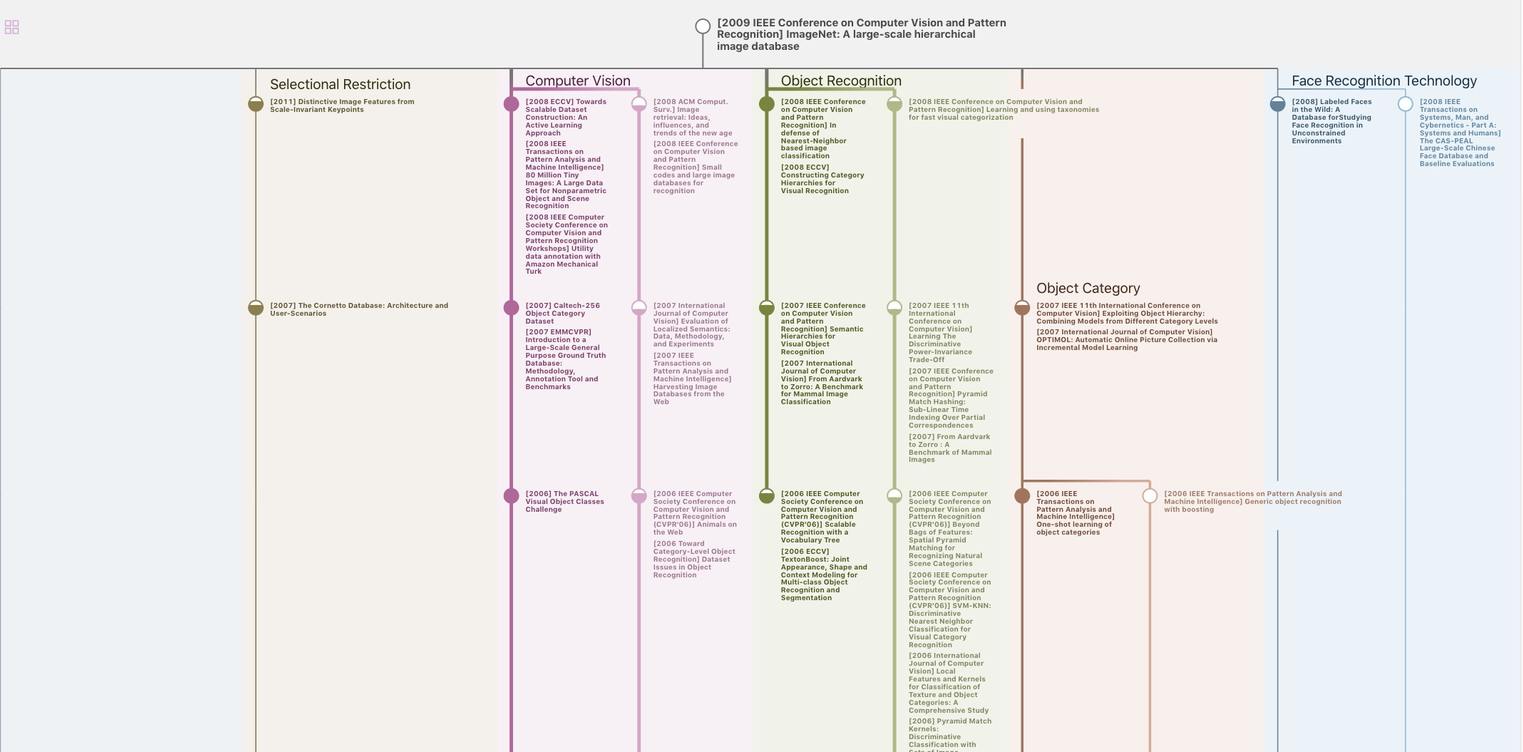
生成溯源树,研究论文发展脉络
Chat Paper
正在生成论文摘要