Improved dequantization and normalization methods for tabular data pre-processing in smart buildings.
BuildSys@SenSys(2022)
摘要
Ubiquitous deployment of IoT sensors marks a defining characteristic of smart buildings, for they constitute the source of data on building operation, diagnosis, and maintenance. For machine learning applications in buildings, often the sensor data is augmented with several other artificial variables or metadata corresponding to building components including the occupants. Above datasets are usually organized in the form of a table with rows and columns, and inherently comprise a mix of continuous and discrete (nominal, ordinal) features/columns, thus are called tabular datasets. A vast majority of smart building datasets are tabular in nature. Machine learning algorithms, especially deep neural networks are generally designed as smooth function approximators, and hence are difficult to train optimally with tabular data without appropriate pre-processing. In this work, we analyze the challenges faced by conventional methods for tabular data pre-processing, and propose the use of two improved data transformation methods, namely variational dequantization (for discrete features), and mode-specific normalization (for continuous features). We show improved thermal preference classification performance for two key thermal comfort datasets with the proposed pre-processing. Since the methods are designed in a generalizable way to work for any tabular dataset, we envision them to be an integral part of machine learning algorithm development pipeline for a plethora of smart building applications.
更多查看译文
关键词
Tabular Data, Continuous and Discrete Features, Data Pre-Processing, Thermal Comfort, Classification
AI 理解论文
溯源树
样例
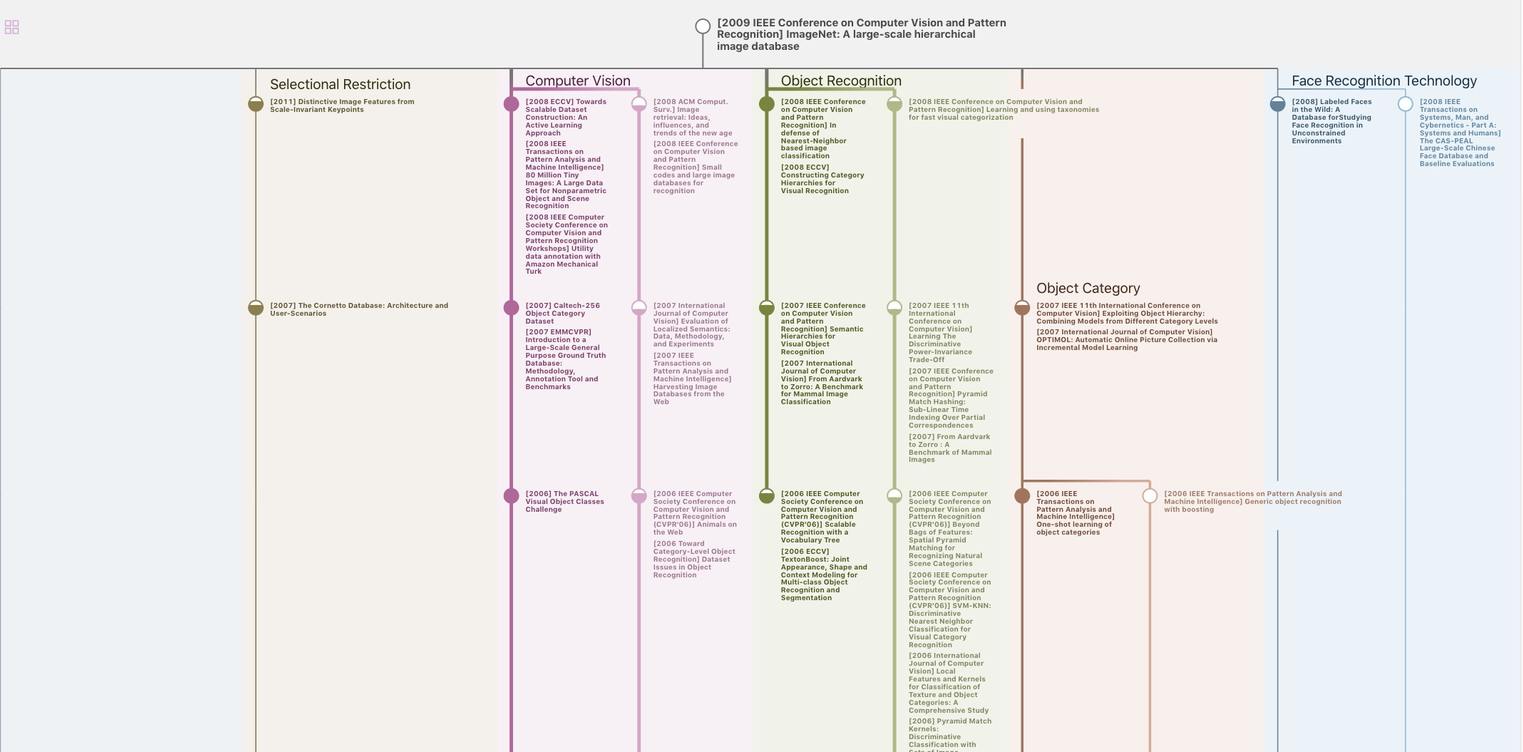
生成溯源树,研究论文发展脉络
Chat Paper
正在生成论文摘要