Routing for Fairness and Efficiency in a Queueing Model with Reentry and Continuous Customer Classes.
ACC(2022)
摘要
This paper presents a new framework to study customer routing fairness and efficiency in managing queueing systems with reentry; this framework is motivated by applications in healthcare and criminal justice. Each customer's reentry probability is captured by a proportional hazard model that depends on the type of service offered and the customer's continuous risk score, which is drawn from group-dependent distributions. Unlike the machine learning literature that focuses on fairness in classification with group-dependent risk distributions, we account for fairness directly in the resource allocation captured via the routing decisions. We derive an explicit analytical characterization of the system's efficiency and fairness for two policies: group-unaware and group-aware routing policies. Through the comparison, we quantify the fairness-efficiency trade-off. This trade-off generates useful insights for decision makers into how the cost of fairness depends on different system parameters and when caution should be taken for fairness considerations.
更多查看译文
关键词
group-dependent risk distributions,routing decisions,group-unaware,group-aware routing policies,fairness-efficiency trade-off,fairness considerations,queueing model,continuous customer classes,customer routing fairness,queueing systems,proportional hazard model,group-dependent distributions,healthcare applications,criminal justice,machine learning literature,resource allocation,decision makers
AI 理解论文
溯源树
样例
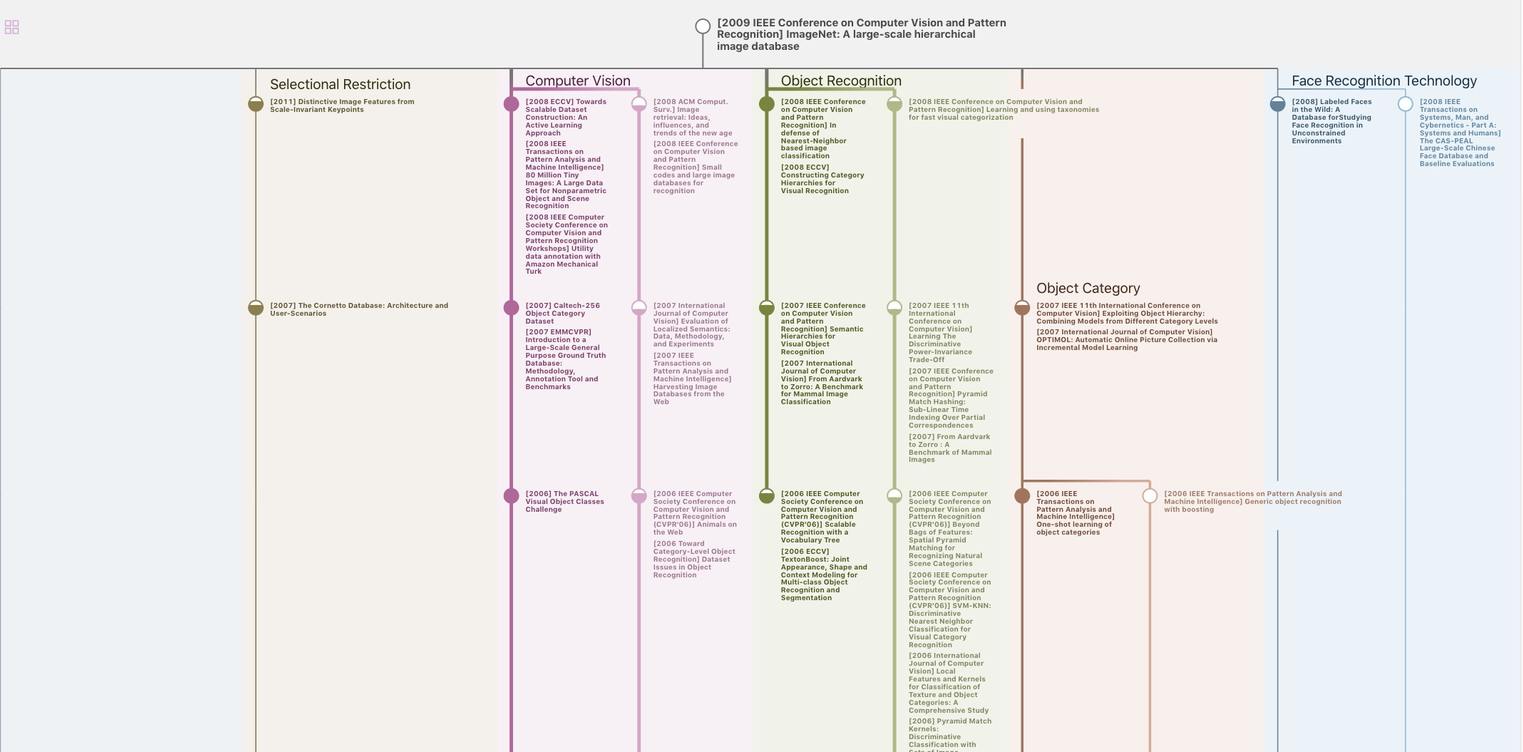
生成溯源树,研究论文发展脉络
Chat Paper
正在生成论文摘要