Text Edges Guided Network for Historical Document Super Resolution.
ICFHR(2022)
摘要
Super-resolution aims to increase the resolution and the clarity of the details in low-resolution images, and document images are no exception. Although significant improvements have been achieved in super-resolution for different domains, historical document images have not been addressed well. Most of the current works in the text domain deal with modern fonts and rely on extracting prior semantic information from a recognizer to super-resolve images. The absence of a reliable handwritten recognizer for Arabic documents, where historical documents have a complex structure and overlapping parts, makes these text-domain works inapplicable. This paper presents a Text-Attentioned Super Resolution GAN (TASR-GAN) to address this problem. The model deals with historical Arabic documents and does not rely on prior semantic information. Since our input domain documents, text edges are essential for quality and readability; thus, we introduce a new loss function called text edge loss. This loss function provides more attention and weight to text edge information and guides through optimization to super-resolve images with accurate small regions' details and fine edges to improve image quality. Experiments on six Arabic manuscripts show that the proposed TASR achieves state-of-the-art performance in terms of PSNR/SSIM metrics and significantly improves the visual image quality, mainly the edges of small regions details, and eliminates artifacts noises. Also, a grid search experiment has been conducted to tune the best hyperparameters values for our text edge loss function.
更多查看译文
关键词
Super-resolution, Historical handwritten documents, Generative adversarial networks
AI 理解论文
溯源树
样例
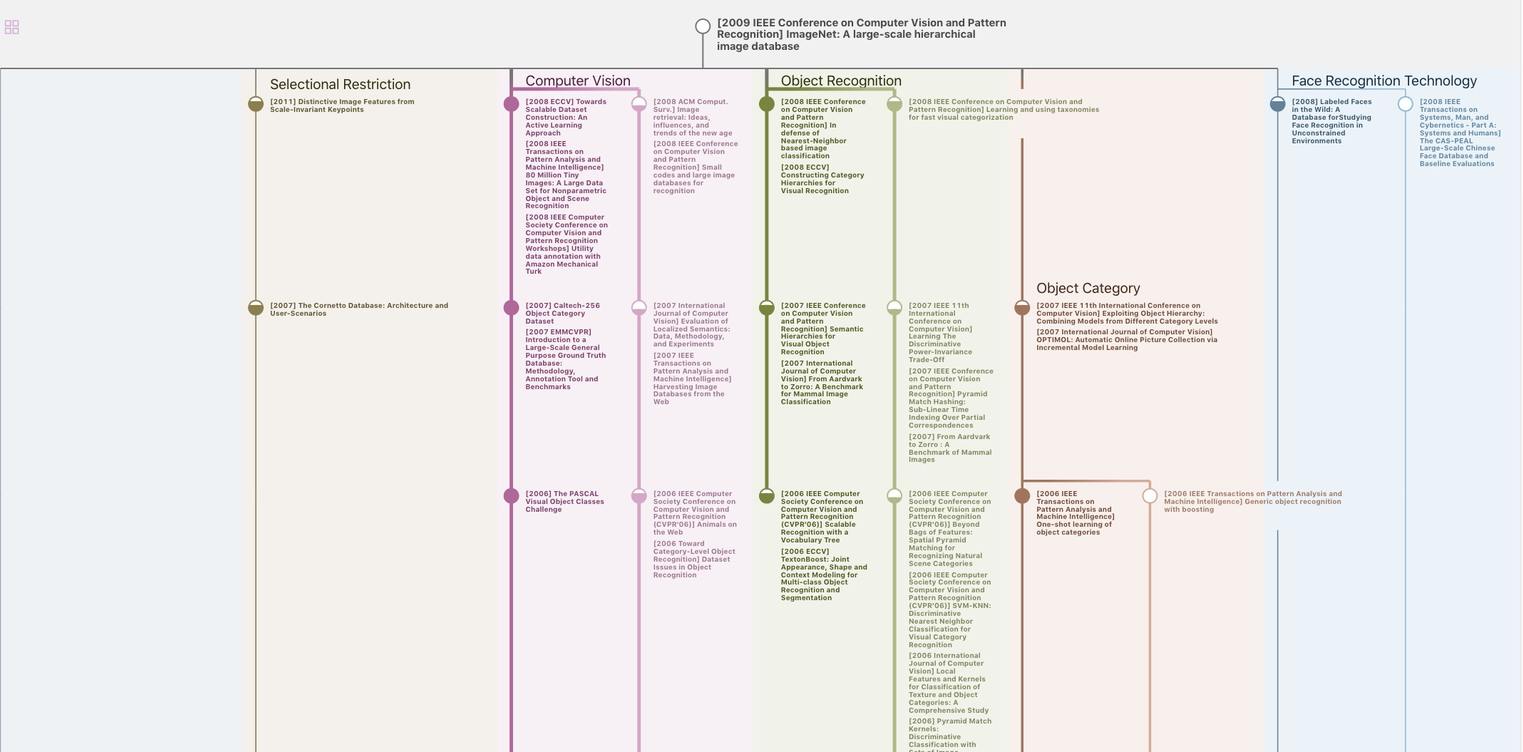
生成溯源树,研究论文发展脉络
Chat Paper
正在生成论文摘要