Hierarchical volumetric transformer with comprehensive attention for medical image segmentation.
Mathematical biosciences and engineering : MBE(2023)
摘要
Transformer is widely used in medical image segmentation tasks due to its powerful ability to model global dependencies. However, most of the existing transformer-based methods are two-dimensional networks, which are only suitable for processing two-dimensional slices and ignore the linguistic association between different slices of the original volume image blocks. To solve this problem, we propose a novel segmentation framework by deeply exploring the respective characteristic of convolution, comprehensive attention mechanism, and transformer, and assembling them hierarchically to fully exploit their complementary advantages. Specifically, we first propose a novel volumetric transformer block to help extract features serially in the encoder and restore the feature map resolution to the original level in parallel in the decoder. It can not only obtain the information of the plane, but also make full use of the correlation information between different slices. Then the local multi-channel attention block is proposed to adaptively enhance the effective features of the encoder branch at the channel level, while suppressing the invalid features. Finally, the global multi-scale attention block with deep supervision is introduced to adaptively extract valid information at different scale levels while filtering out useless information. Extensive experiments demonstrate that our proposed method achieves promising performance on multi-organ CT and cardiac MR image segmentation.
更多查看译文
关键词
convolutional neural network , deep supervision , double transformer , global multi-scale attention , local multi-channel attention , medical image segmentation
AI 理解论文
溯源树
样例
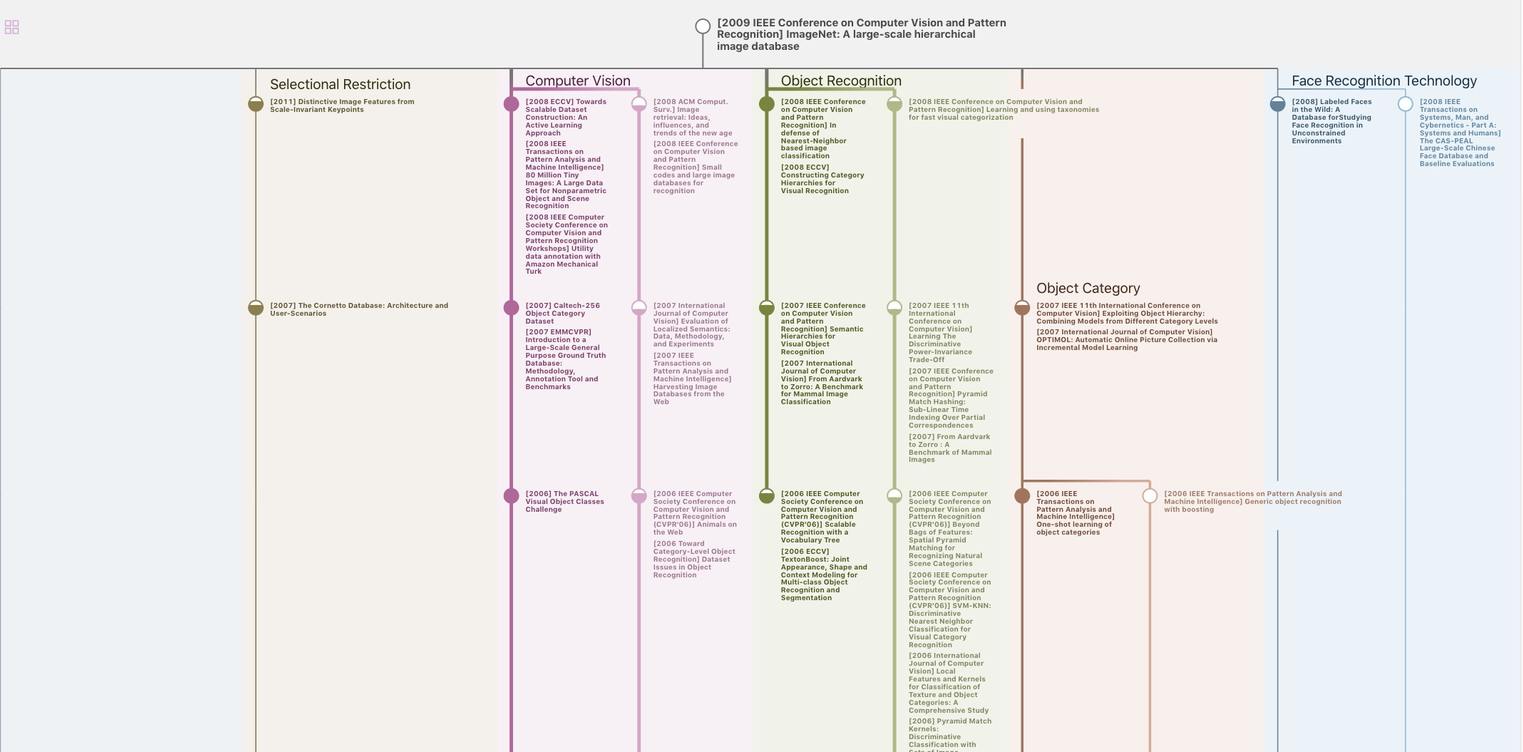
生成溯源树,研究论文发展脉络
Chat Paper
正在生成论文摘要